PatchMask: A Data Augmentation Strategy with Gaussian Noise in Hyperspectral Images.
Remote. Sens.(2022)
Abstract
Data augmentation (DA) is an effective way to enrich the richness of data and improve a model's generalization ability. It has been widely used in many advanced vision tasks (e.g., classification, recognition, etc.), while it can hardly be seen in hyperspectral image (HSI) tasks. In this paper, we analyze whether existing augmentation methods are suitable for the task of HSI denoising and find that the biggest challenge lies in neither losing the spatial information of the original image nor destroying the correlation between the various bands for HSI denoising. Based on this, a new data augmentation method named PatchMask is proposed, which makes the training samples as diverse as possible while preserving the spatial and spectral information. The training data augmented by this method are somewhere between clear and noisy, which can make the network learn more effectively and generalize. Experiments demonstrate that our method outperforms other data augmentation methods, such as the benchmark CutBlur, in enhancing HSI denoising. In addition, the given DA method was used on several popular denoising networks, such as QRNN3D, DnCNN, MPRnet, CBDNet, and HSID-CNN, to verify the effectiveness of the proposed method. The results show that the given DA could increase the value of the PSNR by 0.2 similar to 0.5 dB in various examples.
MoreTranslated text
Key words
data augmentation,hyperspectral image (HSI),HSI denoising,deep learning,convolutional neural network,Gaussian noise
AI Read Science
Must-Reading Tree
Example
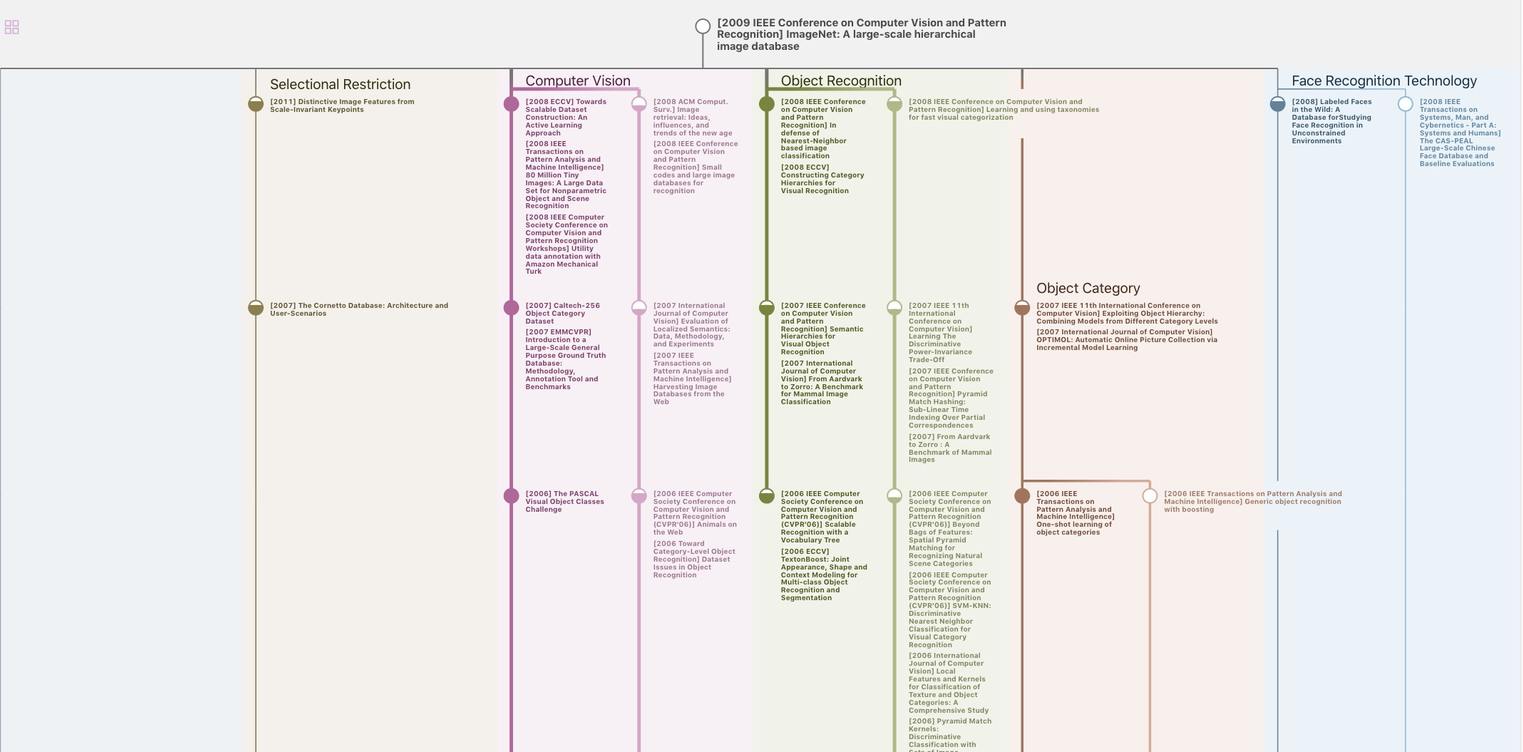
Generate MRT to find the research sequence of this paper
Chat Paper
Summary is being generated by the instructions you defined