Spectral CT Image-Domain Material Decomposition via Sparsity Residual Prior and Dictionary Learning.
IEEE Trans. Instrum. Meas.(2023)
摘要
The spectral computed tomography (CT) system based on a photon-counting detector (PCD) can quantitatively analyze the material composition of the inspected object by material decomposition. Nonetheless, the raw projection of spectral CT is frequently disturbed by noise and artifacts, resulting in poor quality material decomposition images. Recently, a generalized dictionary learning based image-domain material decomposition (GDLIMD) to obtain high-quality material images. DL has great advantages in noise suppression and artifacts, while its protection of the fine structure and edge information is insufficient. To address this limitation, we proposed a sparsity residual prior and dictionary learning (SRPDL) algorithm for spectral CT image-domain material decomposition. The SRPDL method retains the noise-resistance performance of dictionary learning (DL) while introducing the pixel-value-based l(0) norm constraint to guide the material decomposition process by using the structural redundancy information between the prior image and the material images, which further improves structure protection and reduces material misclassification. We conducted numerical simulations, physical phantom, and preclinical experiments to validate and evaluate the SRPDL method. The results demonstrate that the proposed SRPDL method obtained better material decomposition accuracy than the state-of-the-art methods in noise reduction and edge protection.
更多查看译文
关键词
Computed tomography,Image reconstruction,Machine learning,Photonics,Matching pursuit algorithms,Noise reduction,Image edge detection,Dictionary learning (DL),image domain,l(0)-norm,material decomposition,prior image,spectral computed tomography (CT)
AI 理解论文
溯源树
样例
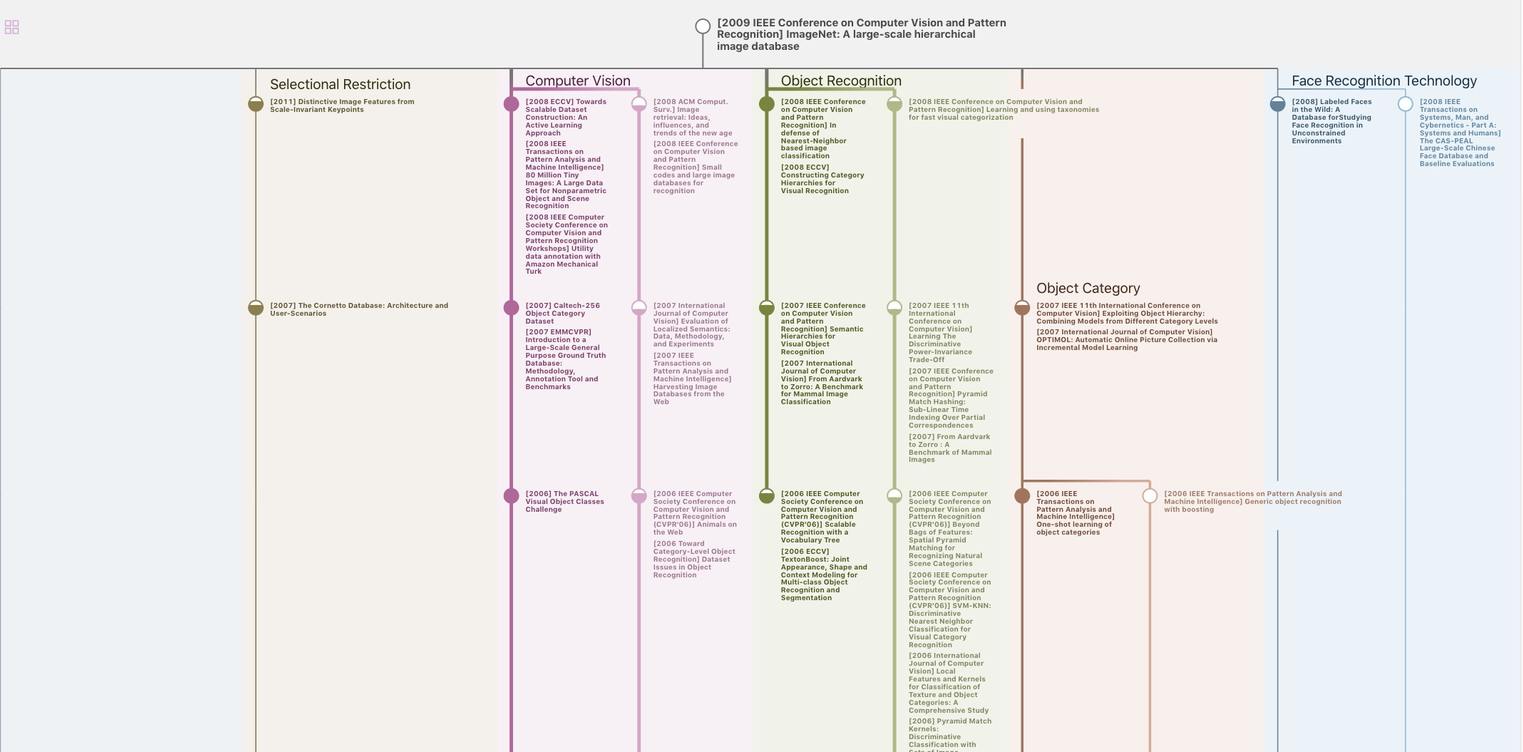
生成溯源树,研究论文发展脉络
Chat Paper
正在生成论文摘要