Machine Learning-based mmWave Path Loss Prediction for Urban/Suburban Macro Sites
GLOBECOM(2022)
Abstract
Millimeter-Wave (mmWave) has great potential to provide high data dates given its large available bandwidth, but its severe path loss and high propagation sensitivity to different environmental conditions make deployment planning particularly challenging. Traditional slope-intercept models fall short in capturing large site-specific variations due to urban clutter, terrain tilt or foliage, and ray-tracing faces challenges in characterizing mmWave propagation accurately with reasonable complexity. In this work, we apply machine learning (ML) techniques to predict mmWave path loss on a link-to-link basis over an extensive set of 28 GHz field measurements collected in a major city of USA, with over 120,000 links from both urban and suburban scenarios, with over 40 dB variation for links at similar distances. Either raw environmental profile (terrain+clutter) of each link or 8 selected expert features are used to either directly predict path loss via regression-based approaches or predict the best performing option out of a pool of theoretical/empirical propagation models. Our evaluation shows that Lasso regression provides the best path loss prediction with a performance (RMSE 8.1 dB) comparable to the per-site slope-intercept fit (RMSE 8.0 dB), whereas model selection method achieves 8.6 dB RMSE, both are significantly better than the best a posteriori 3GPP model (UMa-NLOS, 10.0 dB).
MoreTranslated text
Key words
mmwave path loss prediction,urban/suburban,learning-based
AI Read Science
Must-Reading Tree
Example
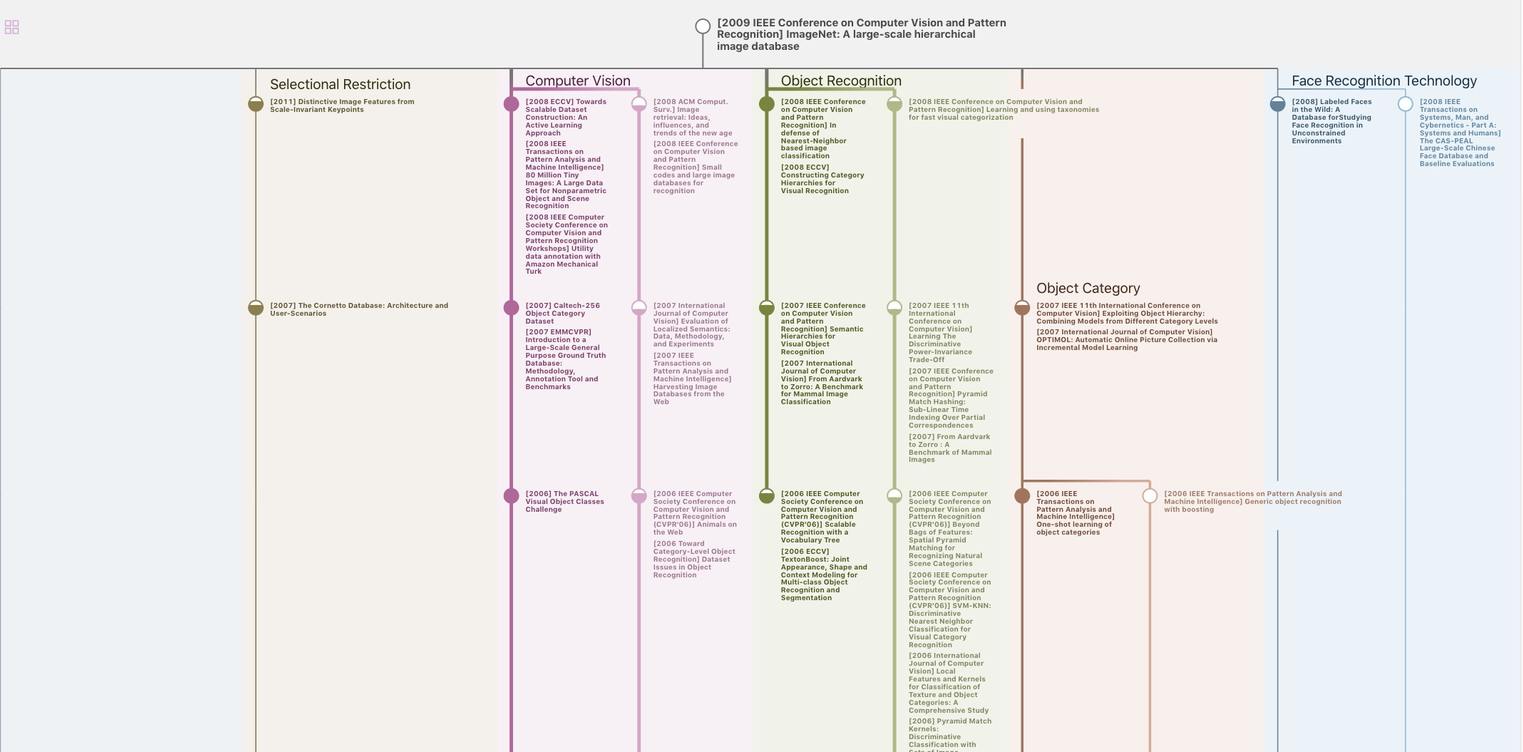
Generate MRT to find the research sequence of this paper
Chat Paper
Summary is being generated by the instructions you defined