Knowledge-Assisted Few-Shot Fault Diagnosis in Cellular Networks
GLOBECOM (Workshops)(2022)
摘要
Data-driven network fault diagnosis faces the great challenge of lacking sufficient high-quality labeled data from real networks. Also, how to exploit existing domain knowledge to aid the diagnosis in an efficient and effective manner needs to be addressed. To obtain a high-performance diagnosis system based on a coarse data set and knowledge base, we propose a Robust Belief Weighting Framework (RBWF) based on abductive learning and belief rule structure for the few-shot fault diagnosis. The correction weight is obtained by the matching rules selected from the insufficient knowledge base to revise pseudo-labels for model retraining. Moreover, the revised pseudo-labels enhance the coarse knowledge base to become more comprehensive. Therefore, the knowledge base and the data-driven model can mutually reinforce to improve the respective performance. Experimental results illustrate the effectiveness and stability of the proposed framework using the cellular network data. Our method enables the automation of fault diagnosis for 5G and 6G networks to achieve highly reliable communication services.
更多查看译文
关键词
Few-Shot Fault Diagnosis,Belief Rule Structure,Abductive Learning,Mutual Reinforcement,Cellular Networks
AI 理解论文
溯源树
样例
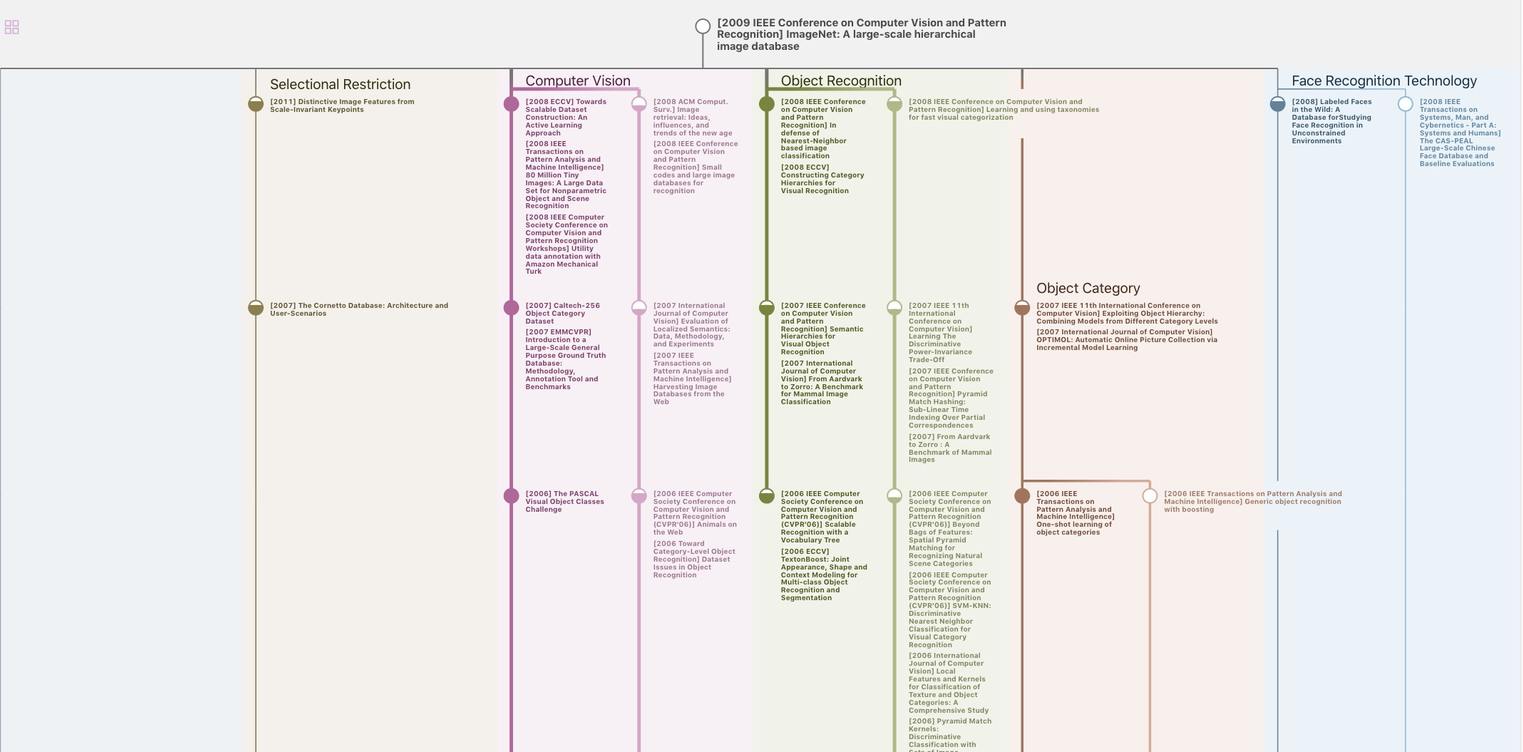
生成溯源树,研究论文发展脉络
Chat Paper
正在生成论文摘要