An Intelligent Defect Detection Approach Based on Cascade Attention Network Under Complex Magnetic Flux Leakage Signals
IEEE Transactions on Industrial Electronics(2023)
摘要
Magnetic flux leakage (MFL) detection robots are broadly employed in acquiring MFL signals to detect pipeline defects. However, influenced by the complex pipeline environments, the accuracy of defect detection under complex MFL signals is undesirable. To resolve this problem, a cascaded attention feature fusion network (CAFF-Net) is proposed in this article. First, a novel feature enhancement method named bidirectional compression is proposed to enhance the feature expression of defects. Second, a multilevel attention feature extraction module is presented, which consists of two cascade networks with different attention mechanisms so that the explicit and implicit features can be fully extracted. Third, a feature aggregation module is put forward to fuse adjacent feature maps from the feature extraction module, which enhances effective dissemination between features. Finally, a feature loss function based on the mechanism feature is designed, and the CAFF-Net model is trained with the proposed loss function. The proposed method can focus on the multilevel explicit and implicit features of complex defects more effectively, and introduces mechanism feature to guide the training of the network for the first time. The proposed method is evaluated by real-world pipelines. The results show that the detection accuracy of the proposed method for complex defects can reach 92% on average, which is 6.4% higher than the best result of state-of-the-art methods.
更多查看译文
关键词
Cascaded attention feature fusion network (CAFF-Net),defect detection,magnetic flux leakage (MFL),mechanism feature
AI 理解论文
溯源树
样例
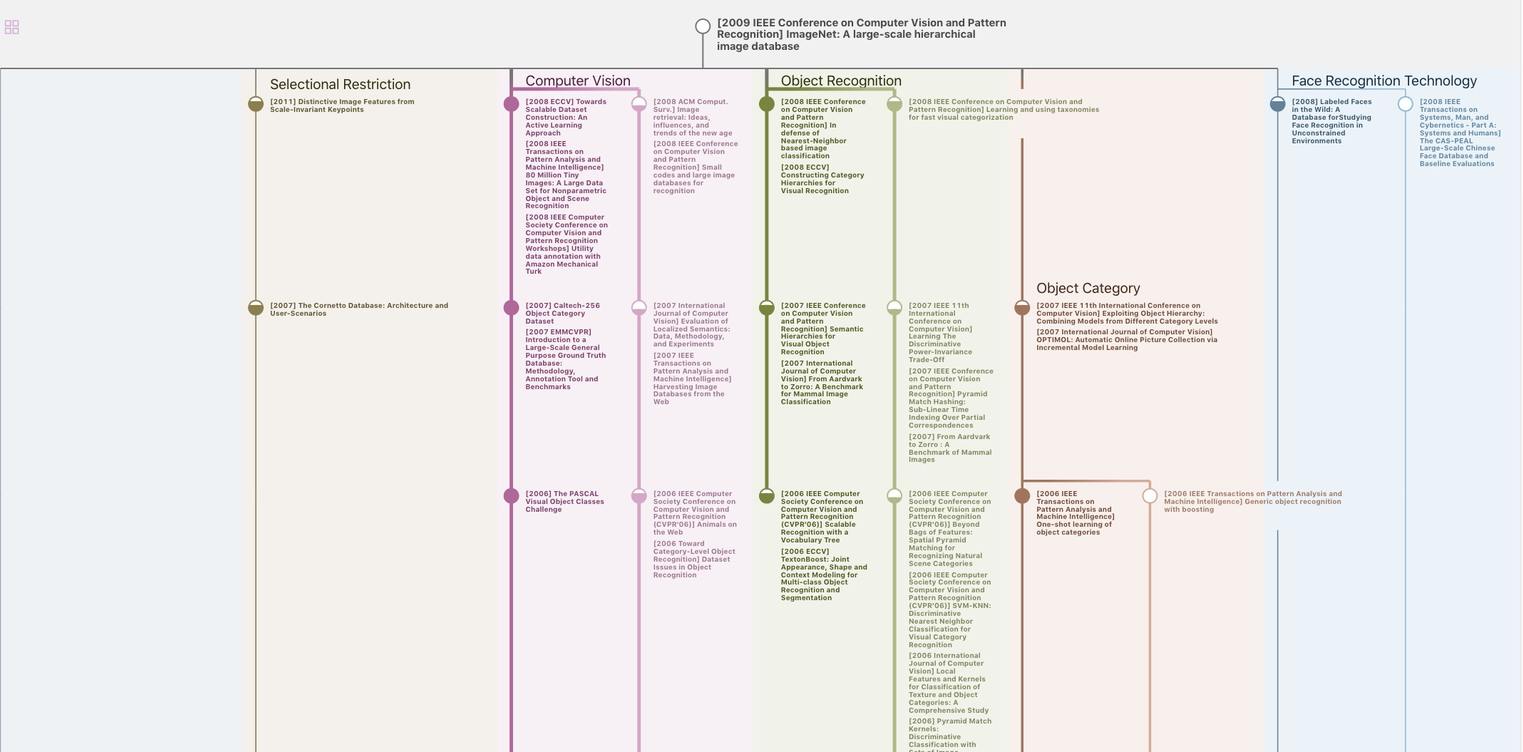
生成溯源树,研究论文发展脉络
Chat Paper
正在生成论文摘要