Segmentation of the aorta in systolic phase from 4D flow MRI: multi-atlas vs. deep learning
Magma (New York, N.Y.)(2023)
摘要
Objective In the management of the aortic aneurysm, 4D flow magnetic resonance Imaging provides valuable information for the computation of new biomarkers using computational fluid dynamics (CFD). However, accurate segmentation of the aorta is required. Thus, our objective is to evaluate the performance of two automatic segmentation methods on the calculation of aortic wall pressure. Methods Automatic segmentation of the aorta was performed with methods based on deep learning and multi-atlas using the systolic phase in the 4D flow MRI magnitude image of 36 patients. Using mesh morphing, isotopological meshes were generated, and CFD was performed to calculate the aortic wall pressure. Node-to-node comparisons of the pressure results were made to identify the most robust automatic method respect to the pressures obtained with a manually segmented model. Results Deep learning approach presented the best segmentation performance with a mean Dice similarity coefficient and a mean Hausdorff distance (HD) equal to 0.92+/− 0.02 and 21.02+/− 24.20 mm, respectively. At the global level HD is affected by the performance in the abdominal aorta. Locally, this distance decreases to 9.41+/− 3.45 and 5.82+/− 6.23 for the ascending and descending thoracic aorta, respectively. Moreover, with respect to the pressures from the manual segmentations, the differences in the pressures computed from deep learning were lower than those computed from multi-atlas method. Conclusion To reduce biases in the calculation of aortic wall pressure, accurate segmentation is needed, particularly in regions with high blood flow velocities. Thus, the deep learning segmen-tation method should be preferred.
更多查看译文
关键词
4D flow MRI,Deep learning segmentation,Multi-atlas segmentation,CFD
AI 理解论文
溯源树
样例
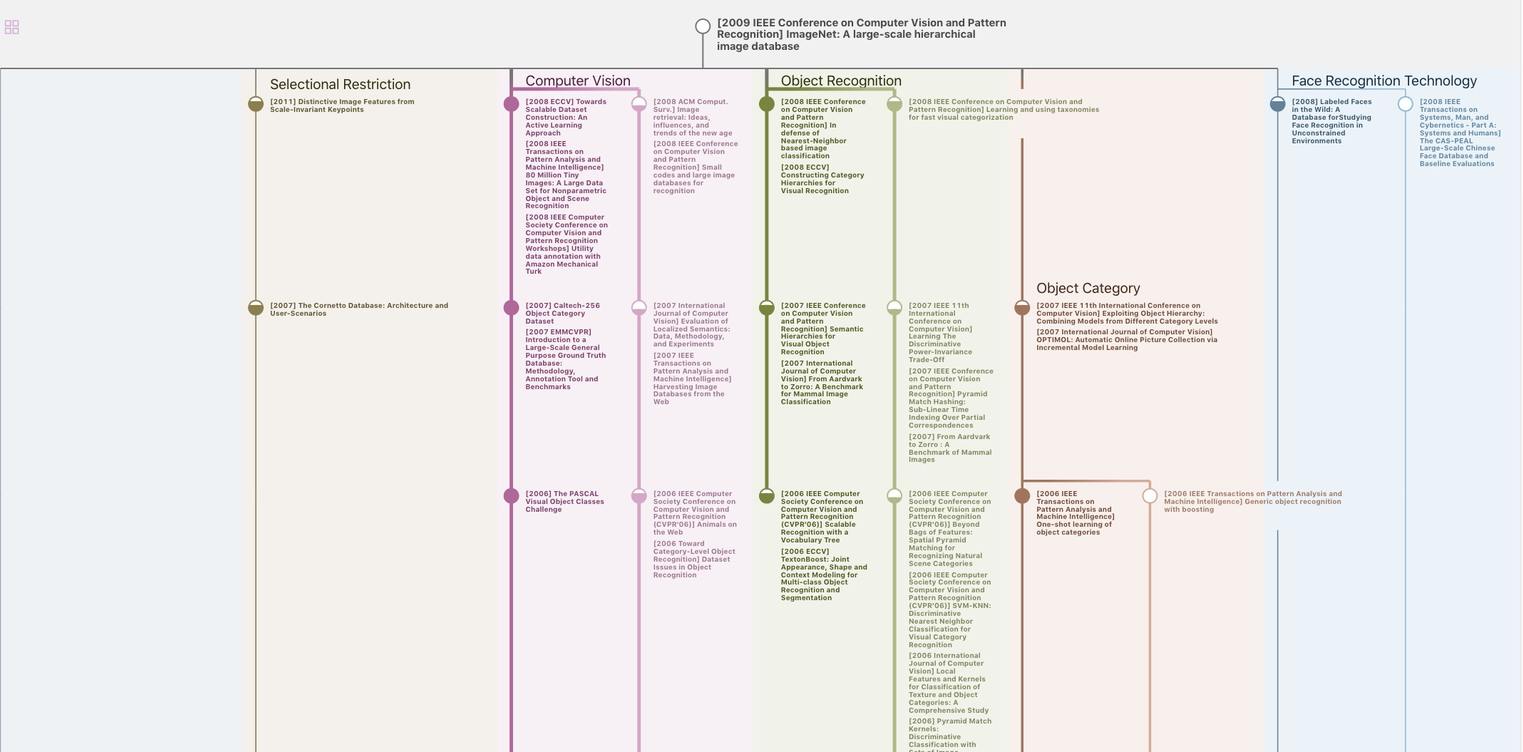
生成溯源树,研究论文发展脉络
Chat Paper
正在生成论文摘要