Generative Molecular Design and Experimental Validation of Selective Histamine H1 Inhibitors
biorxiv(2023)
摘要
Generative molecular design (GMD) is an increasingly popular strategy for drug discovery, using machine learning models to propose, evaluate and optimize chemical structures against a set of target design criteria. We present the ATOM-GMD platform, a scalable multiprocessing framework to optimize many parameters simultaneously over large populations of proposed molecules. ATOM-GMD uses a junction tree variational autoencoder mapping structures to latent vectors, along with a genetic algorithm operating on latent vector elements, to search a diverse molecular space for compounds that meet the design criteria. We used the ATOM-GMD framework in a lead optimization case study to develop potent and selective histamine H1 receptor antagonists. We synthesized 103 of the top scoring compounds and measured their properties experimentally. Six of the tested compounds bind H1 with Kis between 10 and 100 nM and are at least 100-fold selective relative to muscarinic M2 receptors, validating the effectiveness of our GMD approach.
### Competing Interest Statement
The authors have declared no competing interest.
更多查看译文
关键词
selective histamine,molecular design,generative molecular design,inhibitors
AI 理解论文
溯源树
样例
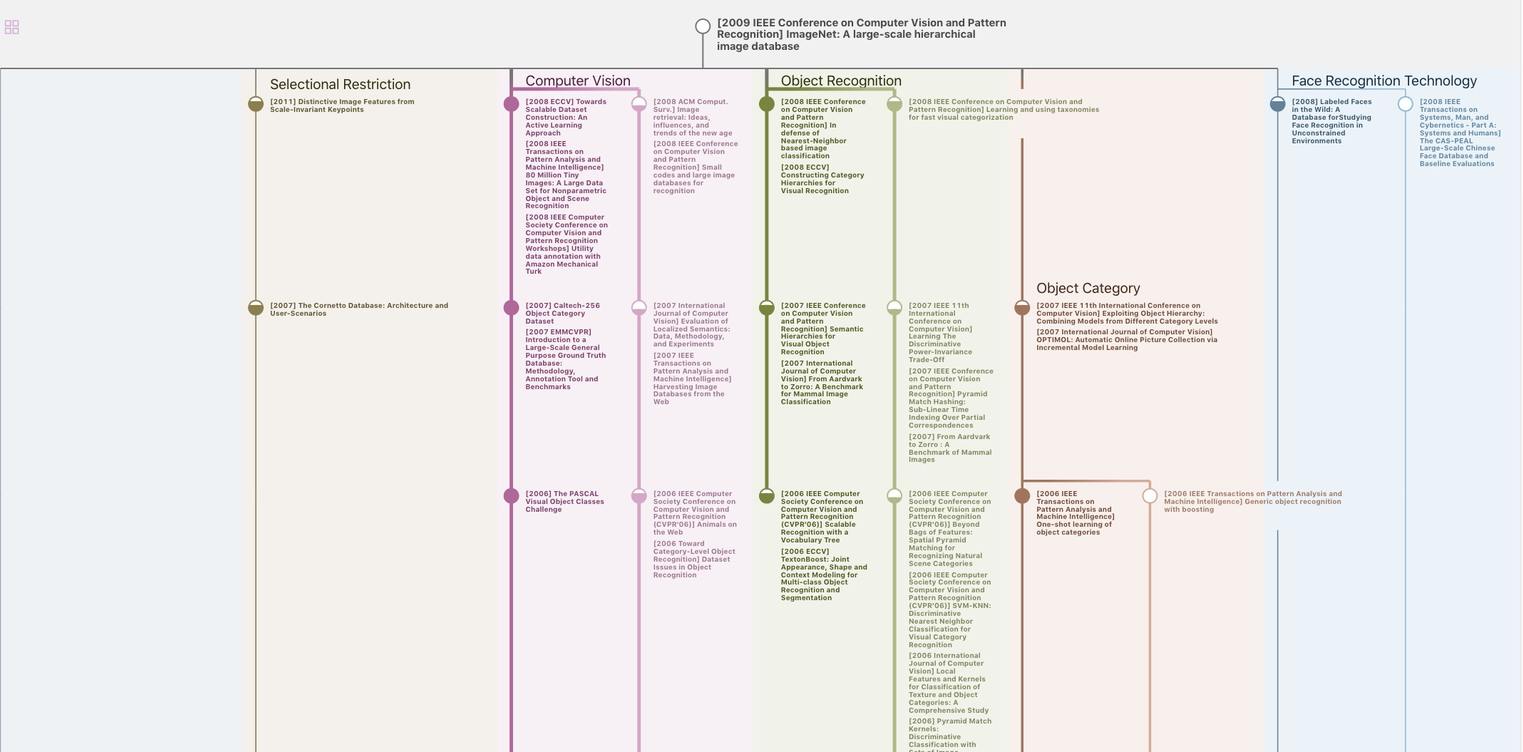
生成溯源树,研究论文发展脉络
Chat Paper
正在生成论文摘要