ClaPIM: Scalable Sequence CLAssification using Processing-In-Memory
arXiv (Cornell University)(2023)
Abstract
DNA sequence classification is a fundamental task in computational biology with vast implications for applications such as disease prevention and drug design. Therefore, fast high-quality sequence classifiers are significantly important. This paper introduces ClaPIM, a scalable DNA sequence classification architecture based on the emerging concept of hybrid in-crossbar and near-crossbar memristive processing-in-memory (PIM). We enable efficient and high-quality classification by uniting the filter and search stages within a single algorithm. Specifically, we propose a custom filtering technique that drastically narrows the search space and a search approach that facilitates approximate string matching through a distance function. ClaPIM is the first PIM architecture for scalable approximate string matching that benefits from the high density of memristive crossbar arrays and the massive computational parallelism of PIM. Compared with Kraken2, a state-of-the-art software classifier, ClaPIM provides significantly higher classification quality (up to 20x improvement in F1 score) and also demonstrates a 1.8x throughput improvement. Compared with EDAM, a recently-proposed SRAM-based accelerator that is restricted to small datasets, we observe both a 30.4x improvement in normalized throughput per area and a 7% increase in classification precision.
MoreTranslated text
Key words
Accelerator,approximate string matching,bioinformatics,deoxyribonucleic acid (DNA) classification,processing-in-memory (PIM)
AI Read Science
Must-Reading Tree
Example
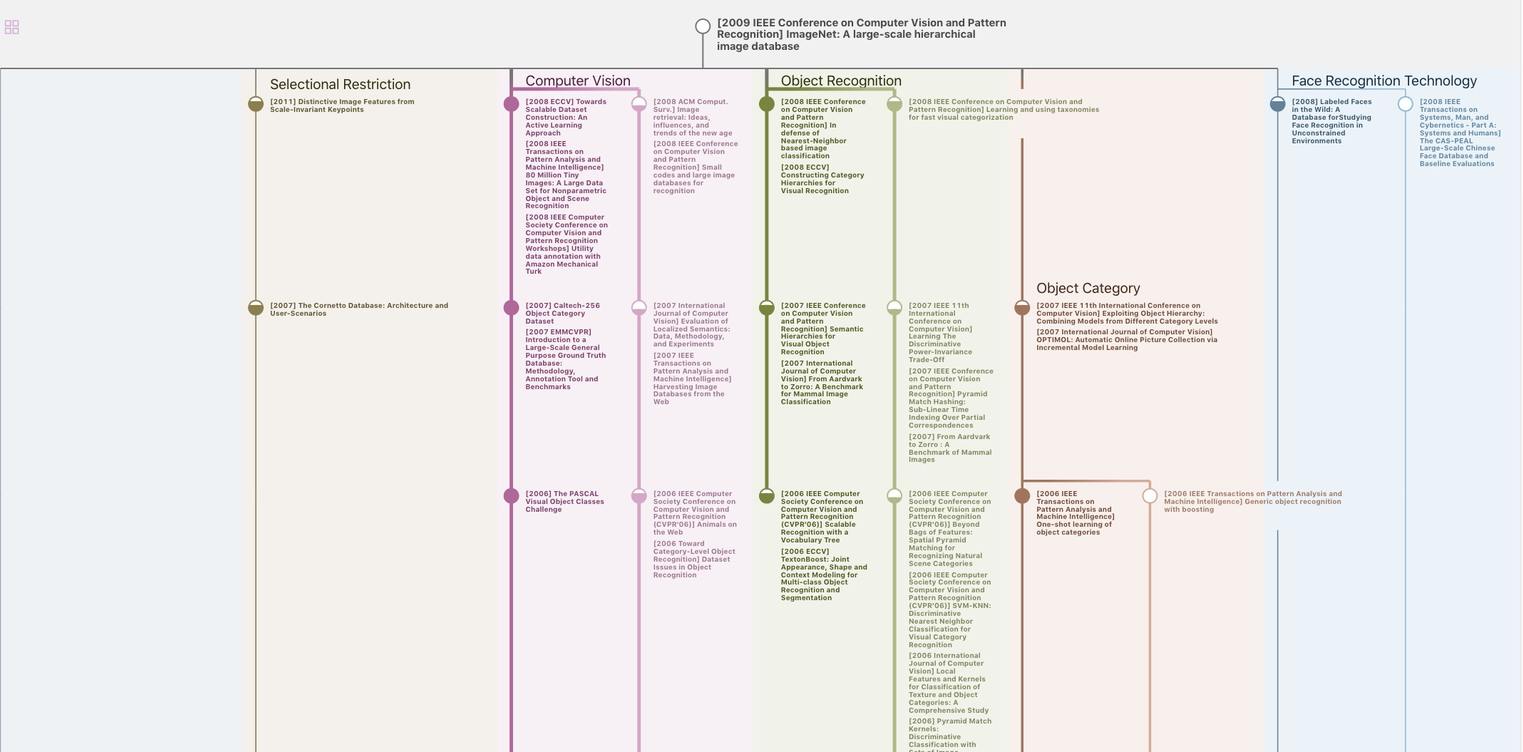
Generate MRT to find the research sequence of this paper
Chat Paper
Summary is being generated by the instructions you defined