Auto-Parallelizing Large Models with Rhino: A Systematic Approach on Production AI Platform
arxiv(2023)
摘要
We present Rhino, a system for accelerating tensor programs with automatic parallelization on AI platform for real production environment. It transforms a tensor program written for a single device into an equivalent distributed program that is capable of scaling up to thousands of devices with no user configuration. Rhino firstly works on a semantically independent intermediate representation of tensor programs, which facilitates its generalization to unprecedented applications. Additionally, it implements a task-oriented controller and a distributed runtime for optimal performance. Rhino explores on a complete and systematic parallelization strategy space that comprises all the paradigms commonly employed in deep learning (DL), in addition to strided partitioning and pipeline parallelism on non-linear models. Aiming to efficiently search for a near-optimal parallel execution plan, our analysis of production clusters reveals general heuristics to speed up the strategy search. On top of it, two optimization levels are designed to offer users flexible trade-offs between the search time and strategy quality. Our experiments demonstrate that Rhino can not only re-discover the expert-crafted strategies of classic, research and production DL models, but also identify novel parallelization strategies which surpass existing systems for novel models.
更多查看译文
关键词
production ai platform,large models,auto-parallelizing
AI 理解论文
溯源树
样例
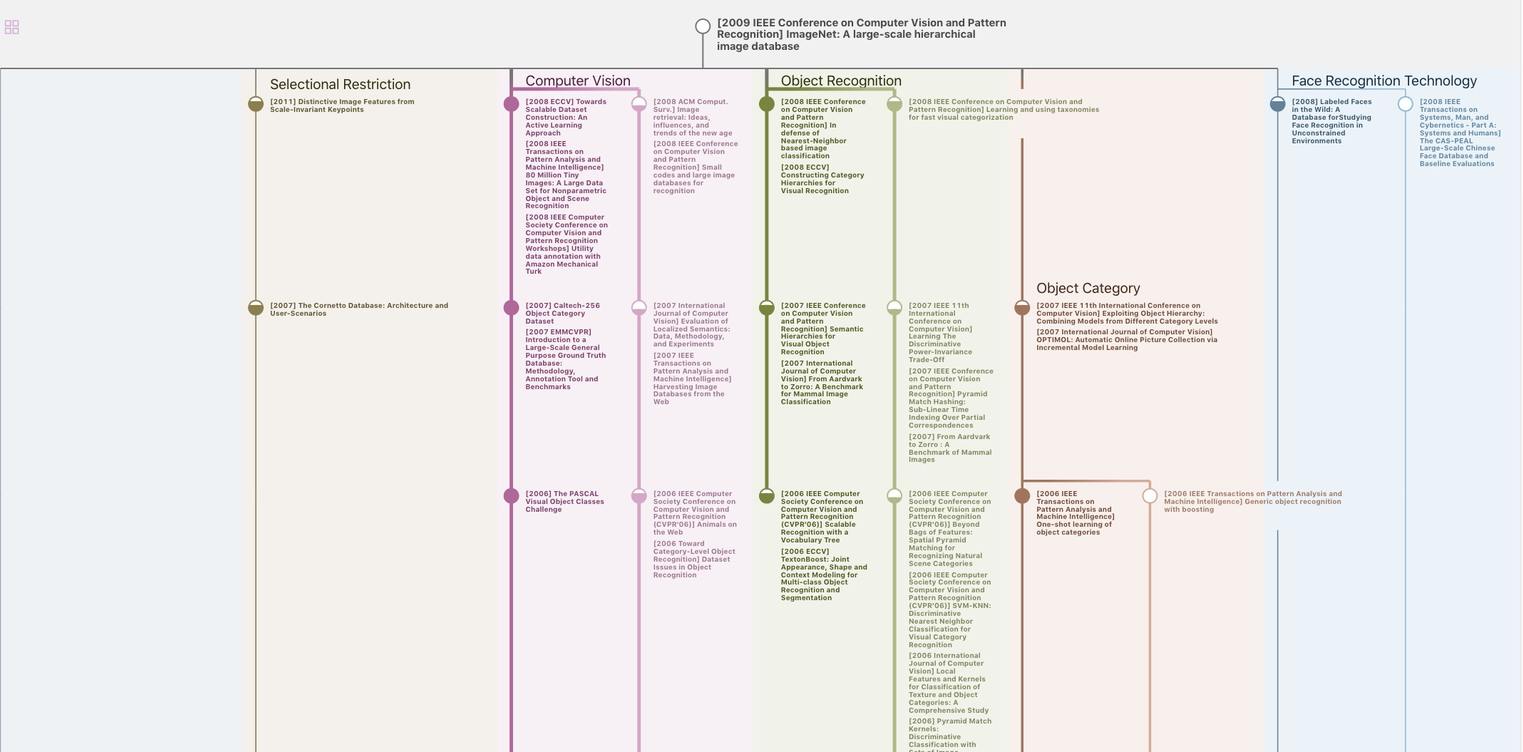
生成溯源树,研究论文发展脉络
Chat Paper
正在生成论文摘要