Audio-Visual Class Association Based on Two-stage Self-supervised Contrastive Learning towards Robust Scene Analysis
2023 IEEE/SICE International Symposium on System Integration (SII)(2023)
摘要
This paper proposes a novel audio and visual class association method based on contrastive learning that can obtain not only one-to-one but also one-to-many, many-to-many, and even no correspondence between audio and visual classes. The proposed method consists of two training stages. In the first stage, for “correspondence” training, using self-supervised contrastive learning, one-to-one, one-to-many, and many-to-many correspondences are trained under a criterion that corresponding AV pairs become close and non-corresponding pairs are far. In the second stage, for “non-correspondence” training, those relationships are acquired through contrastive learning using a dataset consisting of pairs of visual and audio classes that have no correspondence. To build such a dataset, we utilize the trends of change in the class embeddings and split a set of all the classes into two subsets, with AV correspondence and without AV correspondence. The trained model with the proposed method was evaluated by the F1-score for the class embedding, and indoor experiment on mapping of two sound sources. As a result, the F1-score was 74.7% after the first stage, and it improved by 1.86 points to 76.6% after the second stage, confirming, the proposed method is effective for mapping between general audio and visual classes, including one-to-many, many-to-many, and non-corresponding classes. Indoor experiment revealed that our model could predict correct correspondence even in real environment.
更多查看译文
关键词
contrastive learning,audio-visual,two-stage,self-supervised
AI 理解论文
溯源树
样例
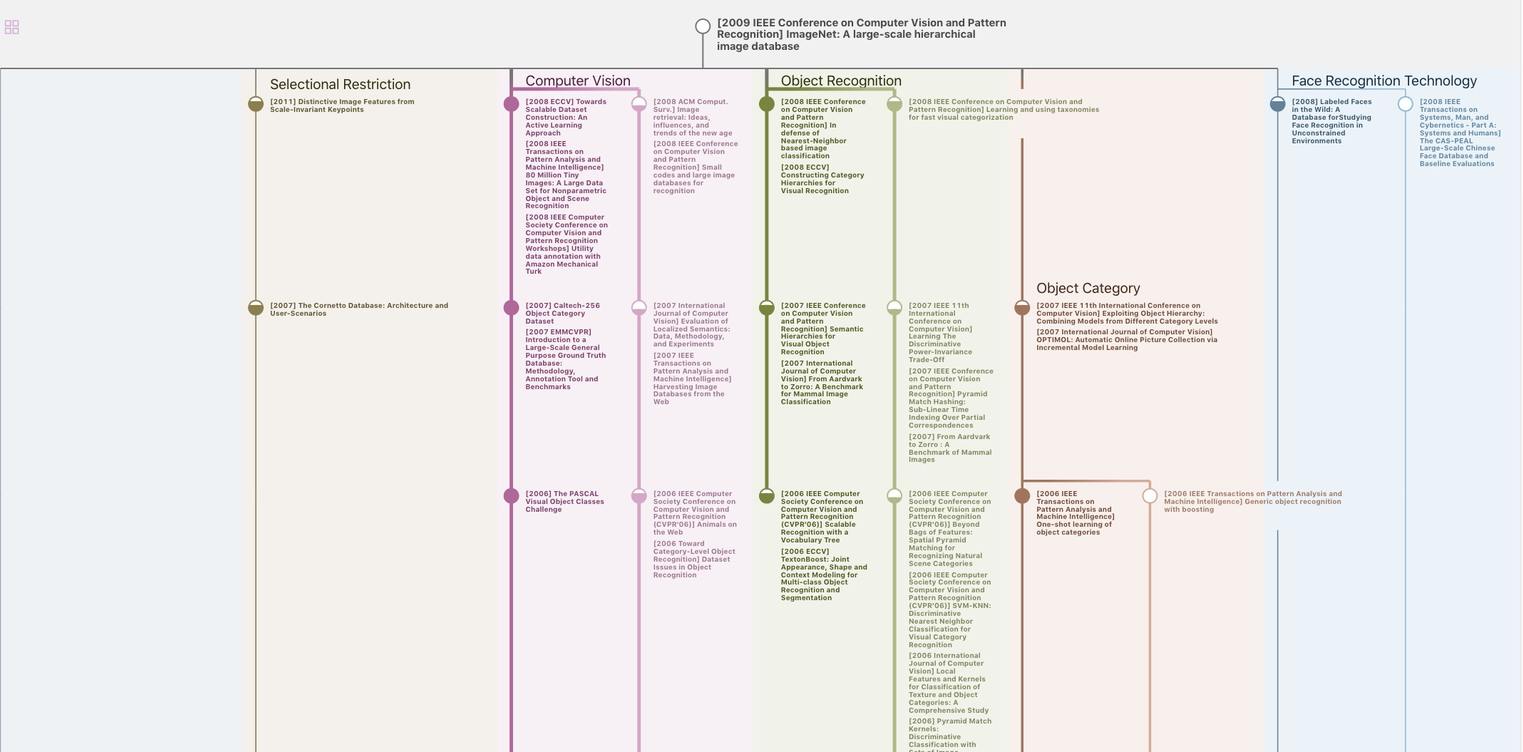
生成溯源树,研究论文发展脉络
Chat Paper
正在生成论文摘要