Rendering Involved and Machine-Learning-based Environment Interpretation
2023 IEEE/SICE International Symposium on System Integration (SII)(2023)
摘要
In this work, I present a methodology for interpreting depth maps obtained from the environment. However, unlike previous studies, the geometry is sequentially estimated by combining CNN classifiers and reinforcement modules rather than using an end-to-end approach. It is assumed that the geometry of the environment is determined from a combination of basic shapes (panel, box, cylinder, sphere). Therefore, the inference process is performed by manipulating the basic geometrical shapes in 3D space. Ultimately, the agent's goal is to compare the depth map obtained from its 3D space with the actual map and approximate it as much as possible. Therefore, the inference process of this algorithm is explainable, and the learning process has the advantage of not requiring a process such as the creation a label manually, and it can run on a relatively small network.
更多查看译文
关键词
environment,interpretation,machine-learning-based machine-learning-based
AI 理解论文
溯源树
样例
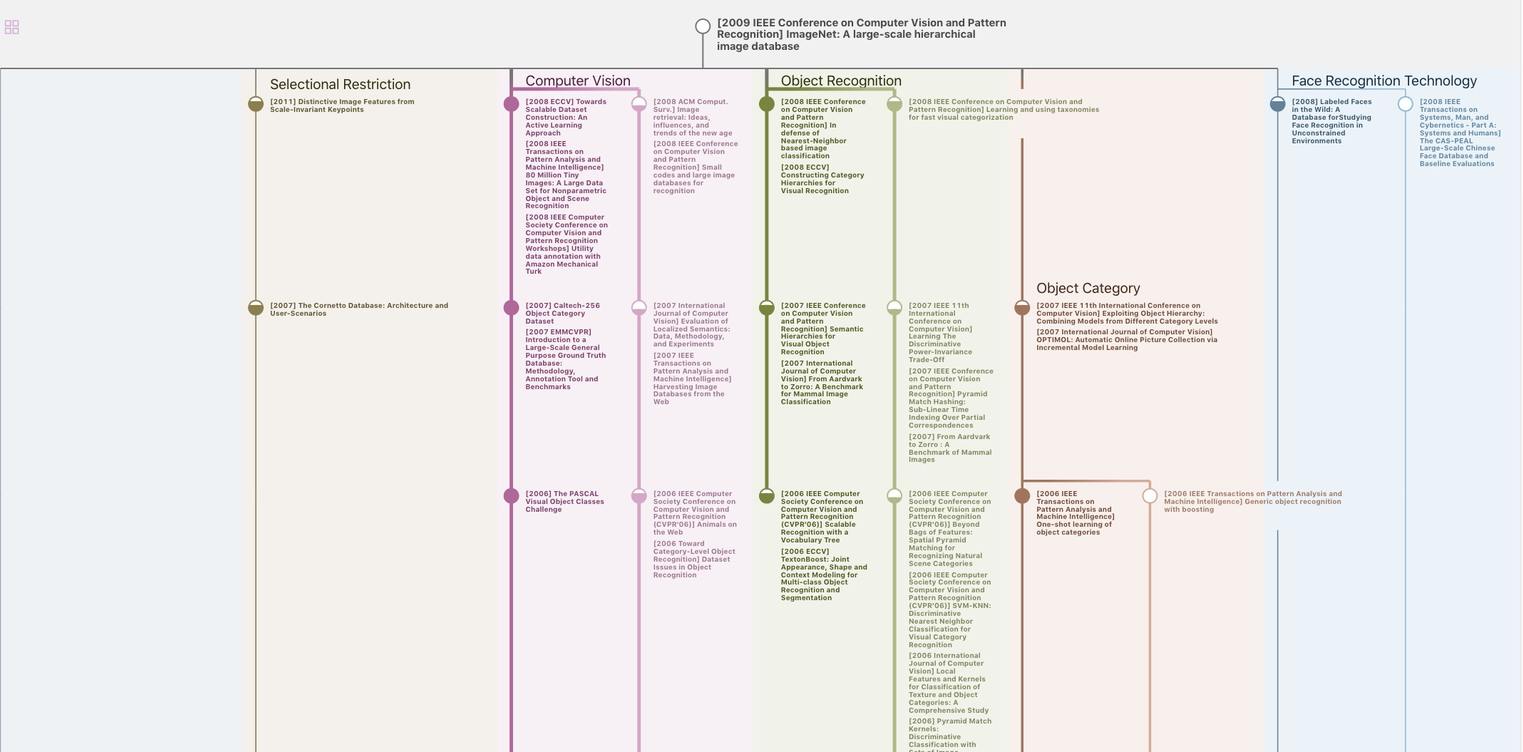
生成溯源树,研究论文发展脉络
Chat Paper
正在生成论文摘要