Pose-Oriented Transformer with Uncertainty-Guided Refinement for 2D-to-3D Human Pose Estimation
arxiv(2023)
摘要
There has been a recent surge of interest in introducing transformers to 3D human pose estimation (HPE) due to their powerful capabilities in modeling long-term dependencies. However, existing transformer-based methods treat body joints as equally important inputs and ignore the prior knowledge of human skeleton topology in the self-attention mechanism. To tackle this issue, in this paper, we propose a Pose-Oriented Transformer (POT) with uncertainty guided refinement for 3D HPE. Specifically, we first develop novel pose-oriented self-attention mechanism and distance-related position embedding for POT to explicitly exploit the human skeleton topology. The pose-oriented self-attention mechanism explicitly models the topological interactions between body joints, whereas the distance-related position embedding encodes the distance of joints to the root joint to distinguish groups of joints with different difficulties in regression. Furthermore, we present an Uncertainty-Guided Refinement Network (UGRN) to refine pose predictions from POT, especially for the difficult joints, by considering the estimated uncertainty of each joint with uncertainty-guided sampling strategy and self-attention mechanism. Extensive experiments demonstrate that our method significantly outperforms the state-of-the-art methods with reduced model parameters on 3D HPE benchmarks such as Human3.6M and MPI-INF-3DHP
更多查看译文
关键词
transformer,human,pose-oriented,uncertainty-guided
AI 理解论文
溯源树
样例
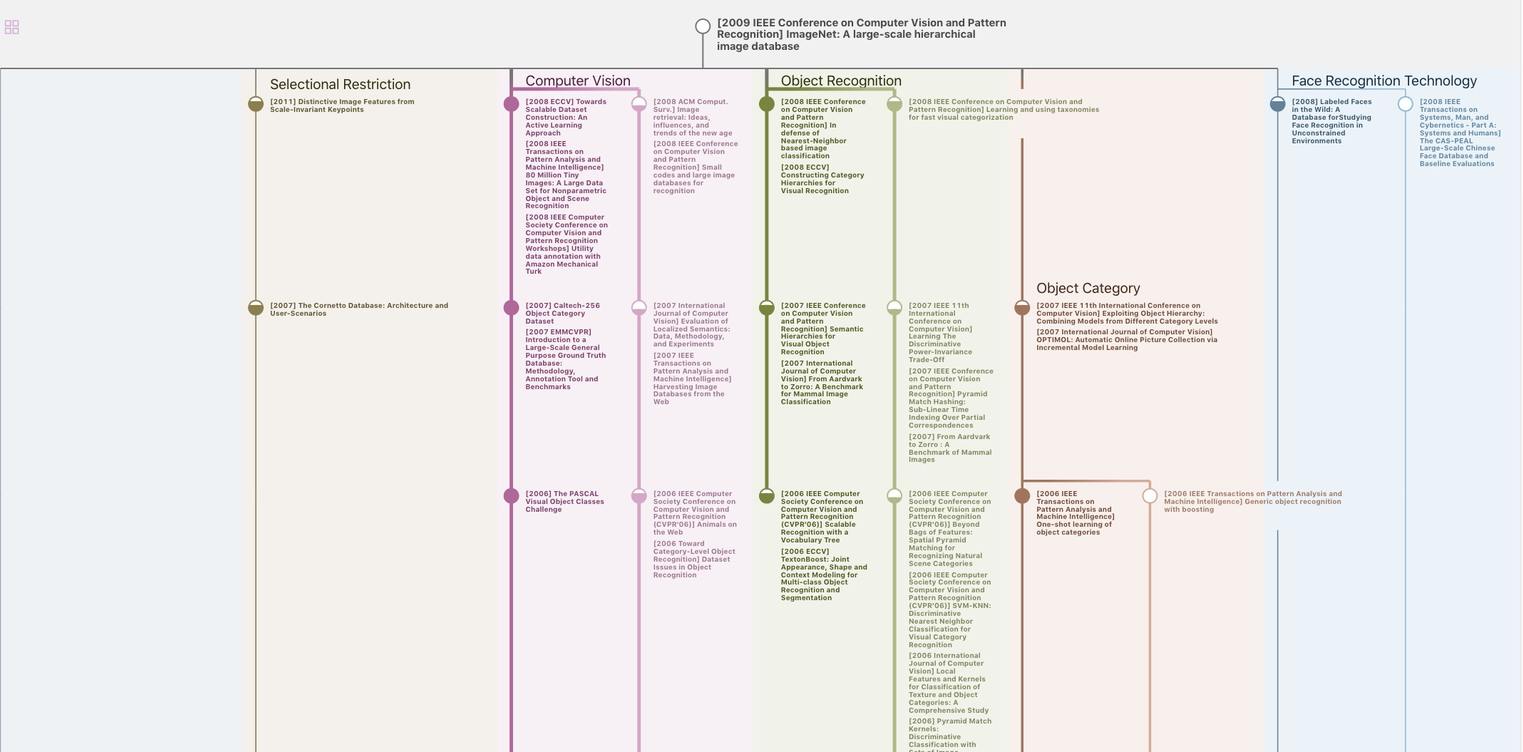
生成溯源树,研究论文发展脉络
Chat Paper
正在生成论文摘要