Reachability-based Trajectory Design with Neural Implicit Safety Constraints
arxiv(2023)
摘要
Generating safe motion plans in real-time is a key requirement for deploying robot manipulators to assist humans in collaborative settings. In particular, robots must satisfy strict safety requirements to avoid self-damage or harming nearby humans. Satisfying these requirements is particularly challenging if the robot must also operate in real-time to adjust to changes in its environment.This paper addresses these challenges by proposing Reachability-based Signed Distance Functions (RDFs) as a neural implicit representation for robot safety. RDF, which can be constructed using supervised learning in a tractable fashion, accurately predicts the distance between the swept volume of a robot arm and an obstacle. RDF's inference and gradient computations are fast and scale linearly with the dimension of the system; these features enable its use within a novel real-time trajectory planning framework as a continuous-time collision-avoidance constraint. The planning method using RDF is compared to a variety of state-of-the-art techniques and is demonstrated to successfully solve challenging motion planning tasks for high-dimensional systems faster and more reliably than all tested methods.
更多查看译文
关键词
trajectory design,neural implicit safety
AI 理解论文
溯源树
样例
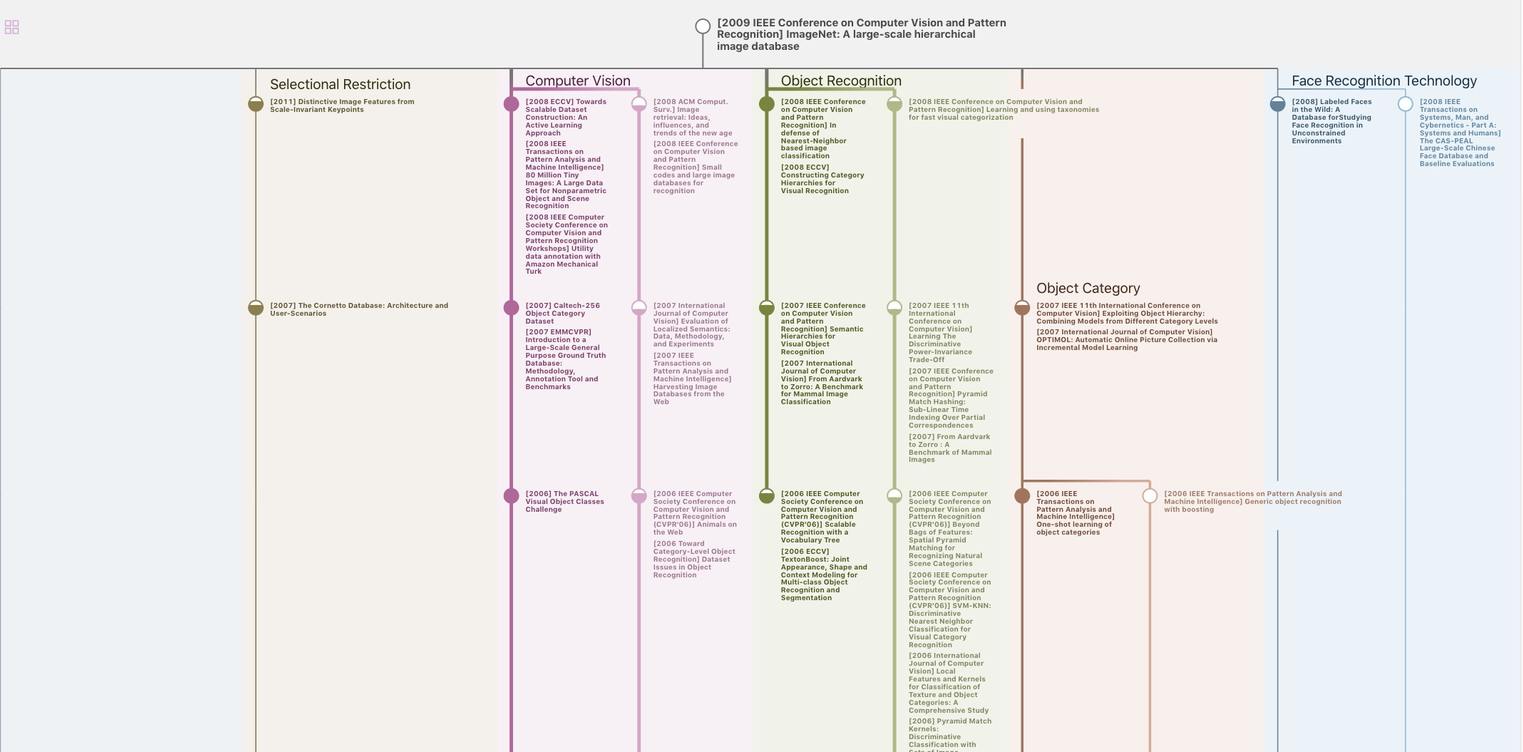
生成溯源树,研究论文发展脉络
Chat Paper
正在生成论文摘要