Internal-Model-Principle-Based Fast Adaptive Iterative Learning Trajectory Tracking Control for Autonomous Farming Vehicle Under Alignment Condition and Input Constraint
IEEE TRANSACTIONS ON SYSTEMS MAN CYBERNETICS-SYSTEMS(2023)
摘要
A novel adaptive iterative learning control (NAILC) strategy is proposed to enhance static and dynamic control performances for the autonomous farming vehicle tracking repetitive trajectories of alternating parallel straight and large curvature. The method integrates an exponential decay function, an internal model principle (IMP), and an input constraint auxiliary variable design system in an adaptive iterative learning control framework. The introduction of exponential decay improves the convergence rate of the iterative process and ensures the control system's dynamic performance. The IMP enhances the static performance of the trajectory tracking control system and guarantees the alignment condition setting. The auxiliary variable design system reduces the adverse effects of input constraints on the control system performance during the iterative process. Moreover, the adaptive iterative learning updating law estimates the total time-varying disturbance to improve the robustness of the tracking process against the actual disturbance. Simulation comparisons with existing results verify the effectiveness and advantages of the designed NAILC strategy under the MATLAB/Simulink environment.
更多查看译文
关键词
Farming,Process control,Trajectory,Trajectory tracking,Vehicle dynamics,Control systems,Convergence,Adaptive iterative learning control (AILC),alignment condition,autonomous farming vehicle,exponential decay function,input constraint,internal model principle (IMP)
AI 理解论文
溯源树
样例
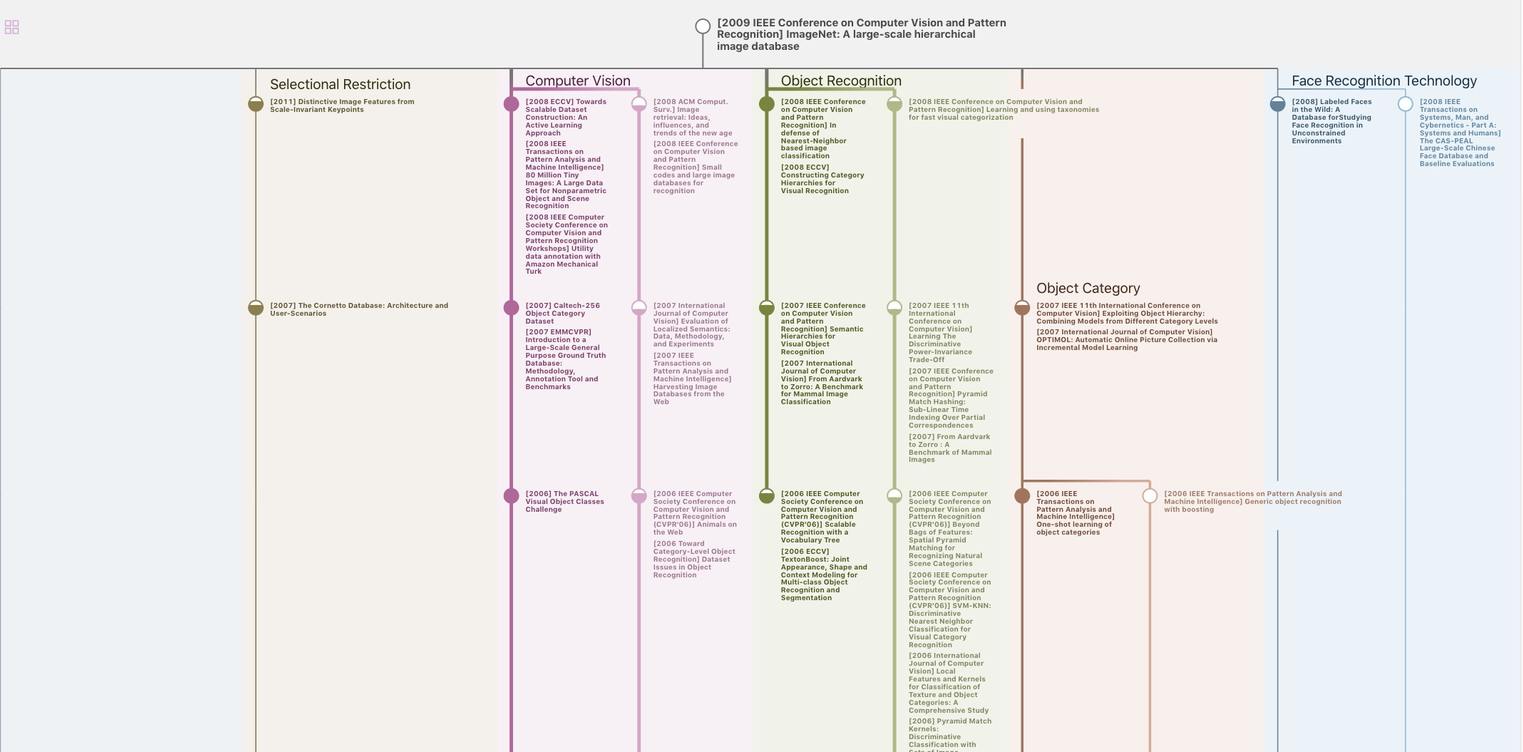
生成溯源树,研究论文发展脉络
Chat Paper
正在生成论文摘要