Regularized Linear Programming Discriminant Rule with Folded Concave Penalty for Ultrahigh-Dimensional Data
JOURNAL OF COMPUTATIONAL AND GRAPHICAL STATISTICS(2022)
摘要
We propose the regularized linear programming discriminant (LPD) rule with folded concave penalty in the ultrahigh-dimensional regime. We use the local linear approximation (LLA) algorithm to redirect the model with folded concave penalty to a weighted l(1) model. The strong oracle property of the solution constructed by the one-step local linear approximation (LLA) algorithm is verified. In addition, we propose efficient and parallelizable algorithms based on feature space split to address the computational challenges due to ultrahigh dimensionality. The proposed feature-split algorithm is compared to existing methods by both numerical simulations and applications to real data examples. The numerical comparisons suggest that the proposed method works well for ultrahigh dimensions, while the linear programming solver and alternating direction method of multiplier (ADMM) algorithm may fail for such high dimensions. for this article are available online.
更多查看译文
关键词
ADMM,Feature-split,Folded concave penalty,Linear programming discriminant
AI 理解论文
溯源树
样例
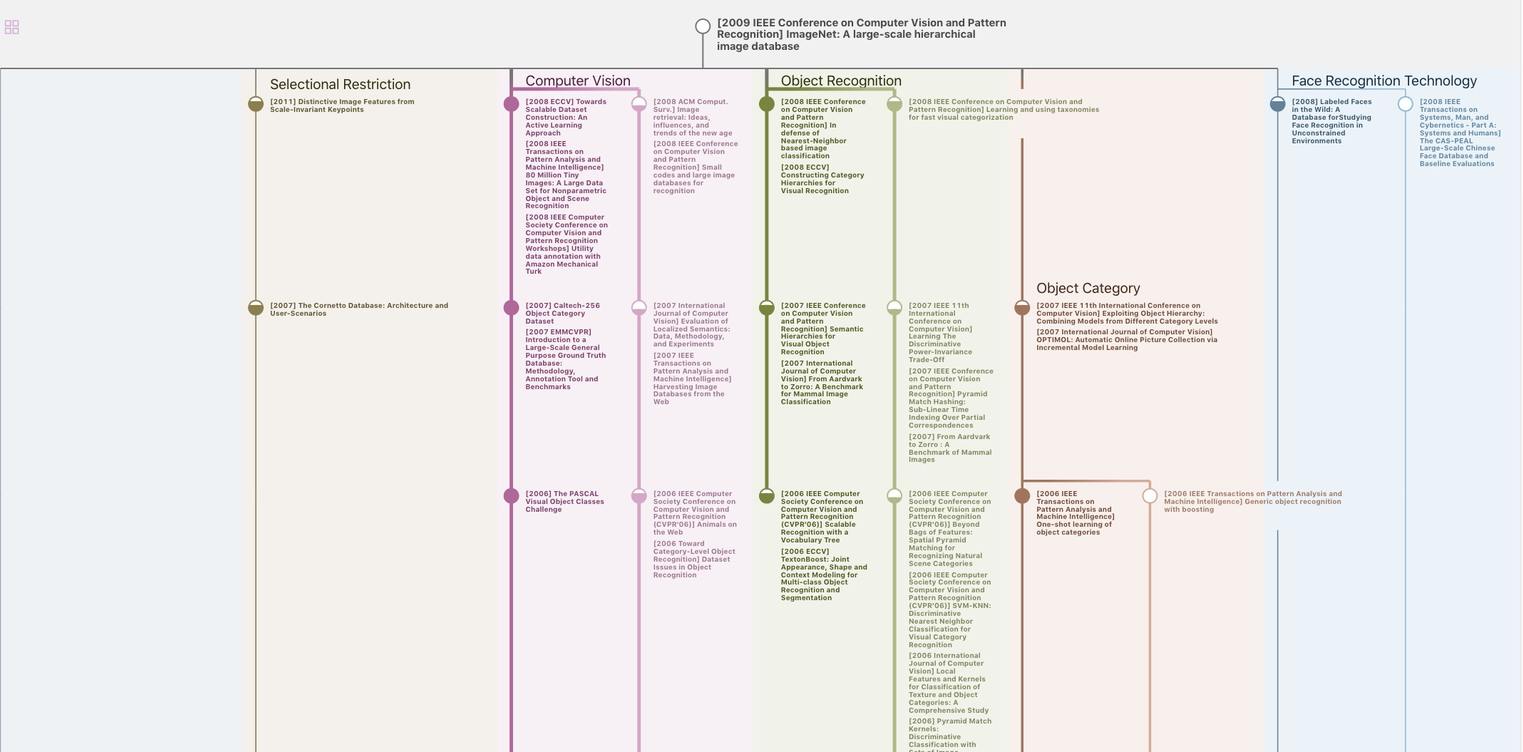
生成溯源树,研究论文发展脉络
Chat Paper
正在生成论文摘要