RAG-PaDiM: Residual Attention Guided PaDiM for Defects Segmentation in Railway Tracks
JOURNAL OF ELECTRICAL ENGINEERING & TECHNOLOGY(2022)
摘要
Railway tracks are facilities for trains that can efficiently transport large-scale objects and it is inevitable to occur track defects that threaten the safety of train operation. Therefore, an inspection of track defects is essential to prevent large-scale accidents caused by anomaly events such as abrasion, fatigue, and deformation. In recent years, Deep Learning-based strategies for robust and highly accurate defect detection have been proposed to replace methods depending on the subjectivity and expertise of inspectors. However, the diversity of abnormal types and the rarity of defective samples make accurate segmentation of defect regions challenging. Therefore, in this paper, we propose a one-class classification algorithm called Residual Attention Guided PaDiM (RAG-PaDiM) for the segmentation of defects in railway tracks. First, the Residual Attention Guided U-Net, which is used as a backbone for generating embedding vectors in RAG-PaDiM, minimizes loss of information using residual connections and gives large weights to the regions of interest through an attention gate. Finally, the distribution of the normal data calculated by learning the training data consisting of only normal samples is used together with the Mahalanobis distance in the test phase to output the anomaly score. The proposed model was rigorously evaluated using the publicly available benchmark dataset, Rail Surface Defect Datasets (RSDD). Experimental results show that the proposed model correctly segmented the pixel-level area under the curve score by about 98.5% for defects in RSDD, suggesting that the proposed method improved the performance in the defect segmentation task for the railway track.
更多查看译文
关键词
Railway tracks,Defects segmentation,PaDiM,Attention gate,Residual connection
AI 理解论文
溯源树
样例
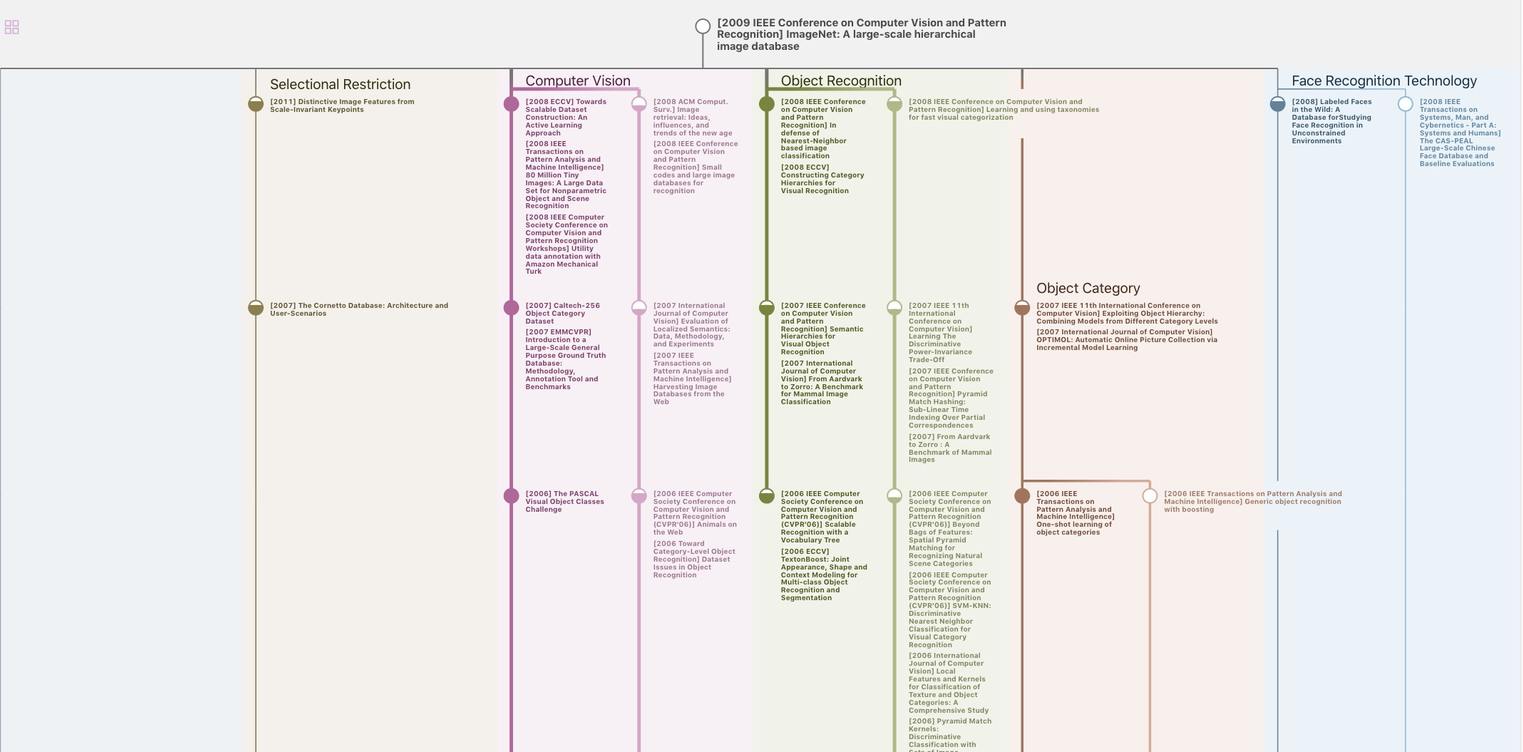
生成溯源树,研究论文发展脉络
Chat Paper
正在生成论文摘要