Surrogate Model Based on Data-Driven Model Reduction for Inelastic Behavior of Composite Microstructure
INTERNATIONAL JOURNAL OF AERONAUTICAL AND SPACE SCIENCES(2022)
摘要
On the microscale, most composite materials are composed of heterogeneous materials comprising two or more different phases, such as matrices and inclusions. In addition, composite materials may exhibit high variability, depending on the material and amount of material used. Hence, the effect of microstructure on the macroscopic structural analysis of composite materials must be considered. Computational homogenization can be used to describe an effective constitutive model for heterogeneous composites at the microscopic level. However, a significant computational cost may be incurred owing to the iterative procedure when considering the inelastic behavior of composite materials. Hence, an efficient data-driven model reduction technique, i.e., a deep-learned surrogate model, is proposed. The key idea of the proposed framework is twofold: (1) Data-driven unsupervised model reduction for efficiently managing high-dimensional data from the microstructure and for extracting those features, and (2) the construction of parameterized constitutive models with inelastic behavior by connecting macro- and microscopic levels. Each aspect leverages a variational autoencoder and a gated recurrent unit, which are state-of-the-art components for deep learning. To demonstrate the efficiency and accuracy of the proposed model, the proposed model is applied to a two-dimensional microstructure problem involving inelastic behavior. Consequently, it is discovered that the present surrogate model can provide improved computational efficiency and accuracy within a prescribed parametric space.
更多查看译文
关键词
Data-driven,Model order reduction,Inelasticity,Surrogate model,Deep learning
AI 理解论文
溯源树
样例
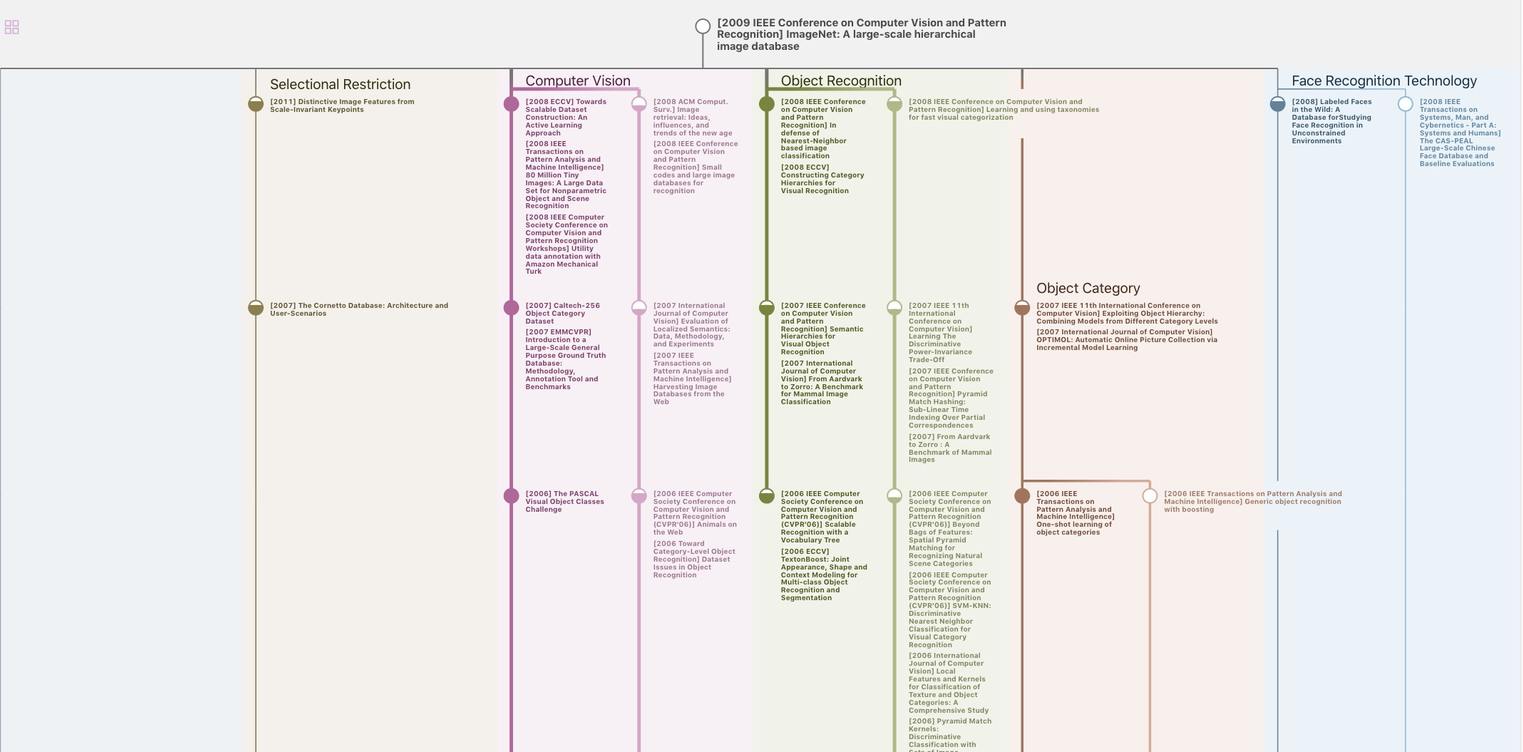
生成溯源树,研究论文发展脉络
Chat Paper
正在生成论文摘要