On improvability of model averaging by penalized model selection
STAT(2023)
摘要
We propose MMAe, a modified MMA based on penalized Mallow's Cp. A weighted elastic net penalty is added to handle the inevitable collinearity among models, which is beneficial to high-dimensional data modelling. We proved the sparsity, the asymptotic optimality of its weight solution and also proved that its candidate model set can be exponentially enlarged under Gaussian noises. We further proved that an MMAe adjusted by generalized cross validation (GCV) has an asymptotically lower risk than MMA under more relaxed conditions. Our approach can be implemented efficiently by convex optimization algorithms. In simulation and real-life analysis, MMAe achieves higher prediction accuracy compared with other methods.
更多查看译文
关键词
asymptotic optimality,convex optimization,GCV,model averaging,sparsified weighting
AI 理解论文
溯源树
样例
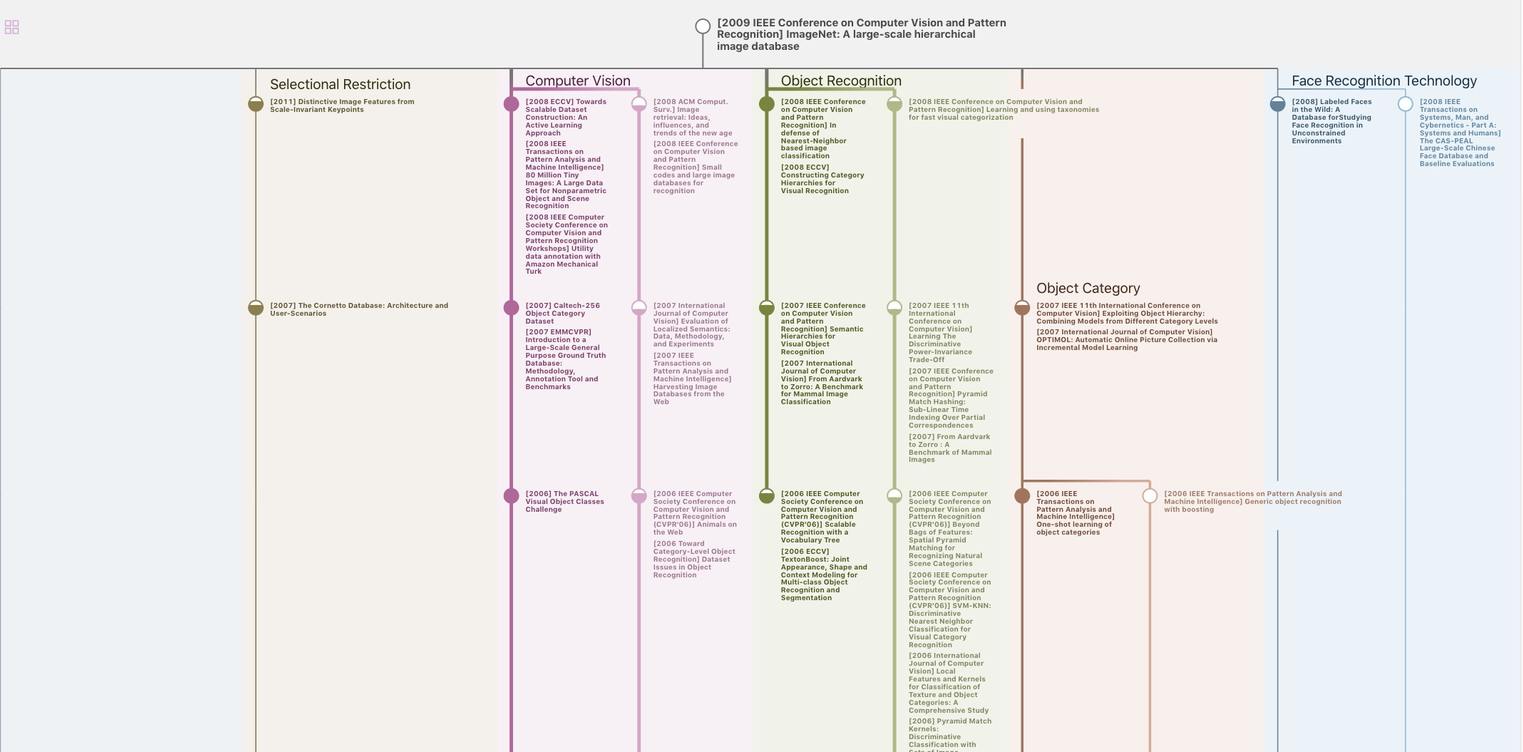
生成溯源树,研究论文发展脉络
Chat Paper
正在生成论文摘要