Estimation of state parameters and road adhesion coefficients for distributed drive electric vehicles based on a strong tracking SCKF
PROCEEDINGS OF THE INSTITUTION OF MECHANICAL ENGINEERS PART D-JOURNAL OF AUTOMOBILE ENGINEERING(2023)
摘要
To address the difficulties in accurately measuring driving state parameters and the road adhesion coefficient, in this paper, the permanent magnet synchronous motor (PMSM) was selected as the automotive in-wheel motor, and the distributed drive electric vehicle (DDEV) simulation model was built based on CarSim and MATLAB/Simulink software. PMSM speed estimation by Adaptive Sliding Mode Observer (ASMO) was used as input information for subsequent studies of state parameters and road adhesion coefficient estimation. Next, this paper established a nonlinear three-degrees-of-freedom whole-vehicle dynamics reference model based on the Dugoff tire model. We derived a suboptimal fading factor using strong tracking theory (STT) and introduced it into the square root of the prediction error covariance of the square-root cubature Kalman filter (SCKF) algorithm. A strong tracking square-root cubature Kalman filter (ST-SCKF) observer was constructed. Improved algorithm estimation accuracy and the ability to cope with sudden changes in operating conditions improve the estimation accuracy of the DDEV driving state parameters and road adhesion coefficient. Finally, a joint CarSim and MATLAB/Simulink simulation and experiments on real vehicles were carried out. The results show that, compared to the traditional CKF (cubature Kalman filter, CKF) and SCKF algorithms, the ST-SCKF algorithm is able to estimate vehicle state parameters and road adhesion coefficients more accurately while maintaining good estimation accuracy for docked road conditions with sudden changes in the road adhesion coefficient.
更多查看译文
关键词
Distributed drive electric vehicle,strong tracking theory,state parameter estimation,road adhesion coefficient,square-root cubature Kalman filter
AI 理解论文
溯源树
样例
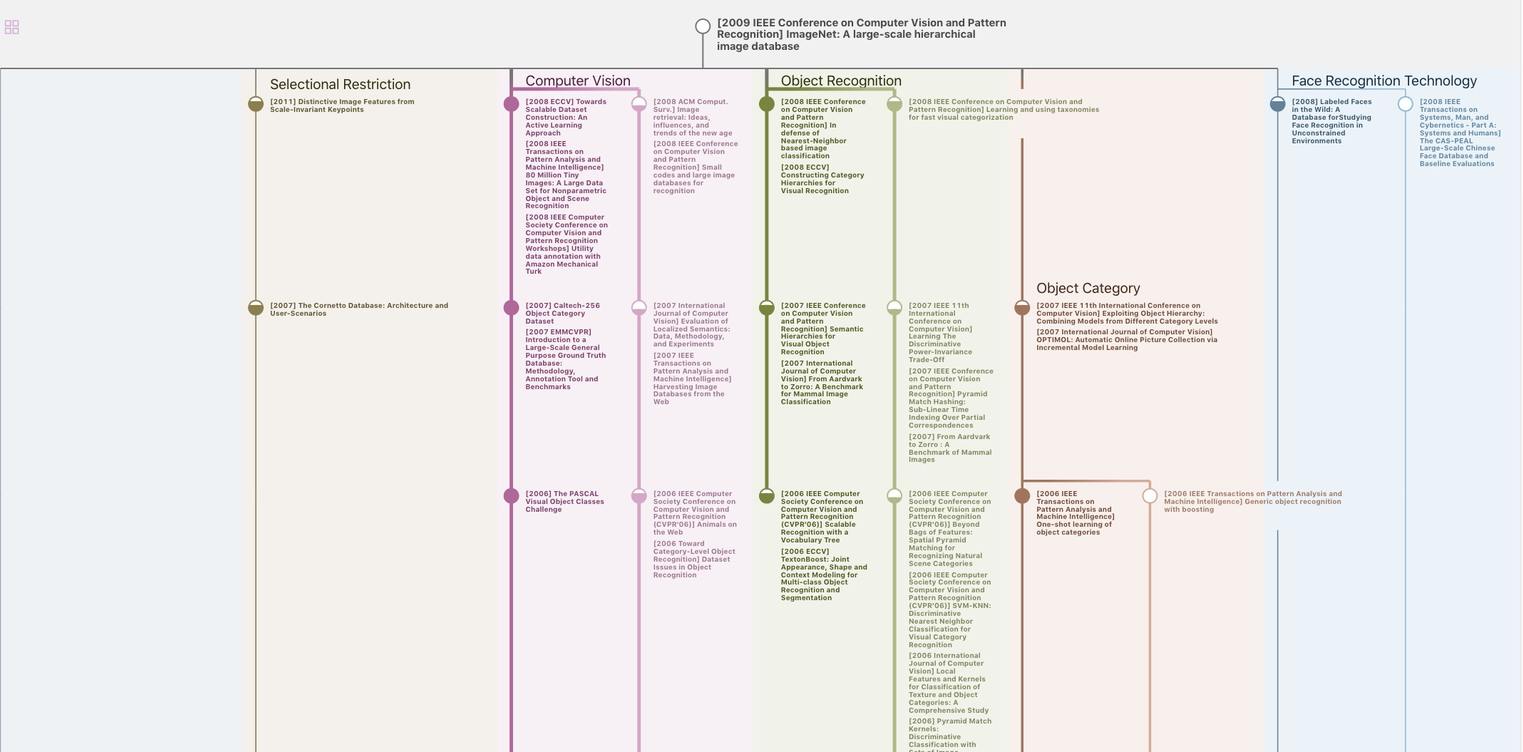
生成溯源树,研究论文发展脉络
Chat Paper
正在生成论文摘要