Joint Front–Edge–Cloud IoVT Analytics: Resource-Effective Design and Scheduling
IEEE Internet of Things Journal(2022)
摘要
A tremendous amount of visual data are bing collected by the Internet of Video Things (IoVT) systems in which ubiquitous cameras deployed in cities enable new applications in the domains of smart transportation and public security. However, the limited resources in terms of communication, computing, and caching (3C) in the conventional cellular network make it challenging to adopt centralized artificial intelligence (AI) to conduct real-time video-based data analytics. In this work, based on the 5G network architecture with edge servers, a three-phase resource-effective solution is proposed to perform surveillance operations in a large-scale wireless IoVT. The proposed strategy integrates front-end cameras with simple on-chip neural networks performing real-time object-of-interest segmentation, edge servers, and cloud servers with AI functionality carrying out image-based target recognition and video-based target analytics tasks. More importantly, we design the optimal 3C strategy to achieve the best video analytics performance constrained by computing offload ratio, network resource allocation and video-related parameters. Extensive simulations with deep neural networks implemented both at the front-end cameras and in the cloud server have validated the effectiveness of the proposed solution.
更多查看译文
关键词
Edge computing,Internet of Video Things (IoVT),resource scheduling,visual analytics
AI 理解论文
溯源树
样例
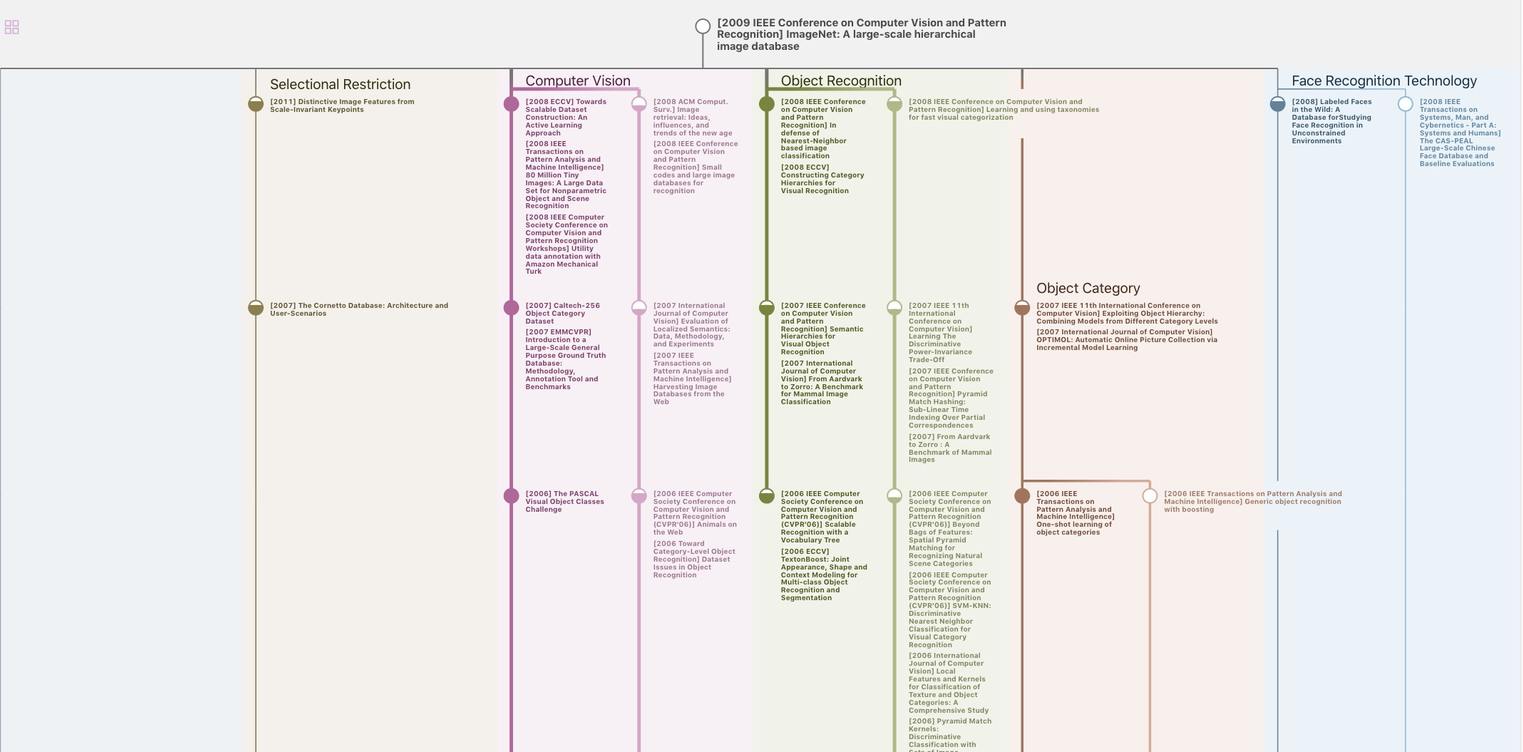
生成溯源树,研究论文发展脉络
Chat Paper
正在生成论文摘要