Cross validation for model selection: A review with examples from ecology
ECOLOGICAL MONOGRAPHS(2023)
摘要
Specifying, assessing, and selecting among candidate statistical models is fundamental to ecological research. Commonly used approaches to model selection are based on predictive scores and include information criteria such as Akaike's information criterion, and cross validation. Based on data splitting, cross validation is particularly versatile because it can be used even when it is not possible to derive a likelihood (e.g., many forms of machine learning) or count parameters precisely (e.g., mixed-effects models). However, much of the literature on cross validation is technical and spread across statistical journals, making it difficult for ecological analysts to assess and choose among the wide range of options. Here we provide a comprehensive, accessible review that explains important-but often overlooked-technical aspects of cross validation for model selection, such as: bias correction, estimation uncertainty, choice of scores, and selection rules to mitigate overfitting. We synthesize the relevant statistical advances to make recommendations for the choice of cross-validation technique and we present two ecological case studies to illustrate their application. In most instances, we recommend using exact or approximate leave-one-out cross validation to minimize bias, or otherwise k-fold with bias correction if k < 10. To mitigate overfitting when using cross validation, we recommend calibrated selection via our recently introduced modified one-standard-error rule. We advocate for the use of predictive scores in model selection across a range of typical modeling goals, such as exploration, hypothesis testing, and prediction, provided that models are specified in accordance with the stated goal. We also emphasize, as others have done, that inference on parameter estimates is biased if preceded by model selection and instead requires a carefully specified single model or further technical adjustments.
更多查看译文
关键词
cross validation,information theory,model selection,overfitting,parsimony,post-selection inference
AI 理解论文
溯源树
样例
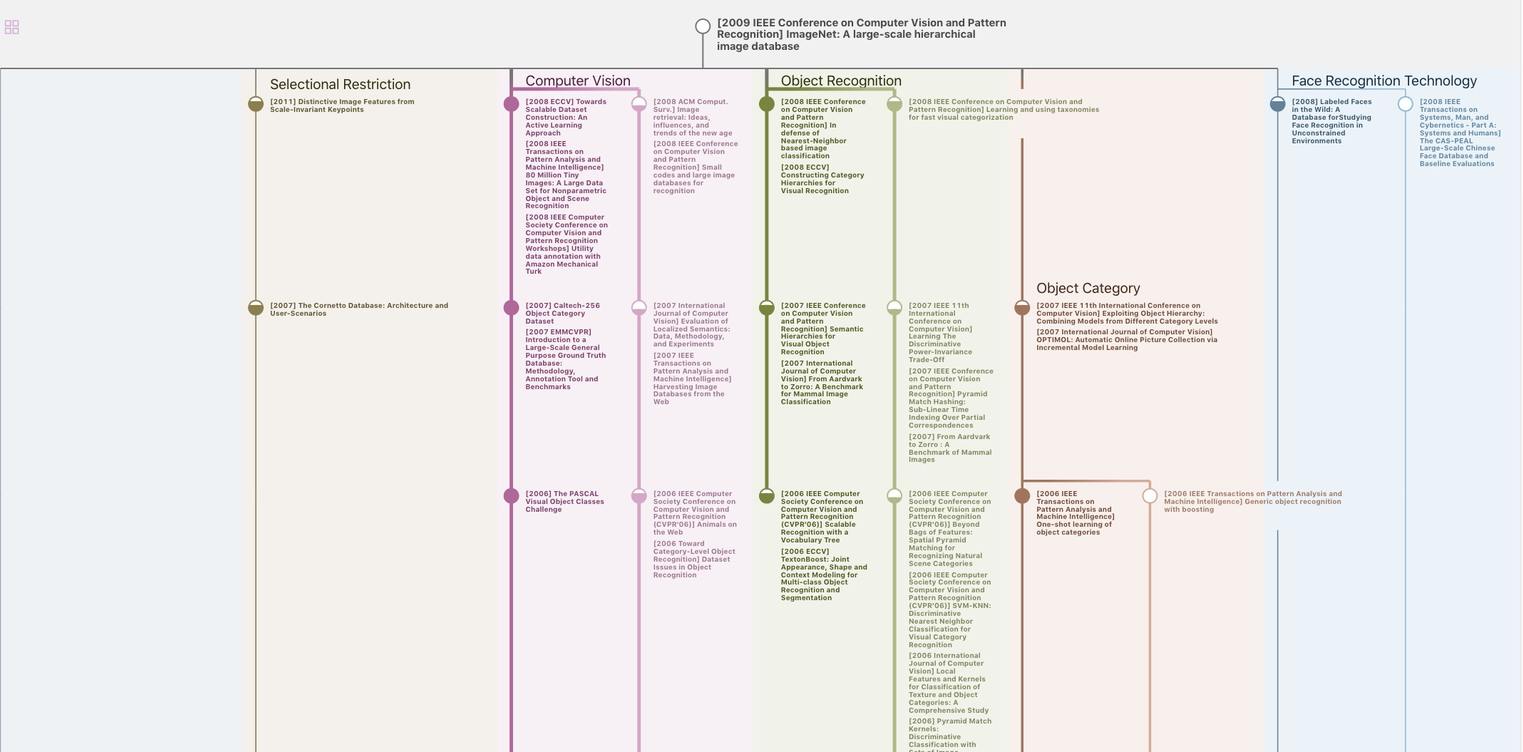
生成溯源树,研究论文发展脉络
Chat Paper
正在生成论文摘要