Probabilistic traffic breakdown forecasting through Bayesian approximation using variational LSTMs
TRANSPORTMETRICA B-TRANSPORT DYNAMICS(2023)
Abstract
This paper proposes a framework for short-term traffic breakdown probability calculation using a Variational LSTM neural network model. Considering that traffic breakdown is a stochastic event, this forecast framework was devised to produce distributions as outputs, which cannot be achieved using standard deterministic recurrent neural networks. Therefore, the model counts on the robustness of neural networks but also includes the stochastic characteristics of highway capacity. The framework consists of three main steps: (i) build and train a probabilistic speed forecasting neural network, (ii) forecast speed distributions with the trained model using Monte Carlo (MC) dropout, and therefore perform Bayesian approximation, and (iii) establish a speed threshold that characterizes breakdown occurrence and calculate breakdown probabilities based on the speed distributions. The proposed framework produced an efficient control over traffic breakdown occurrence, can deal with many independent variables or features, and can be combined with traffic management strategies.
MoreTranslated text
Key words
Traffic breakdown,traffic forecasting,neural networks,Bayesian statistics,machine learning
AI Read Science
Must-Reading Tree
Example
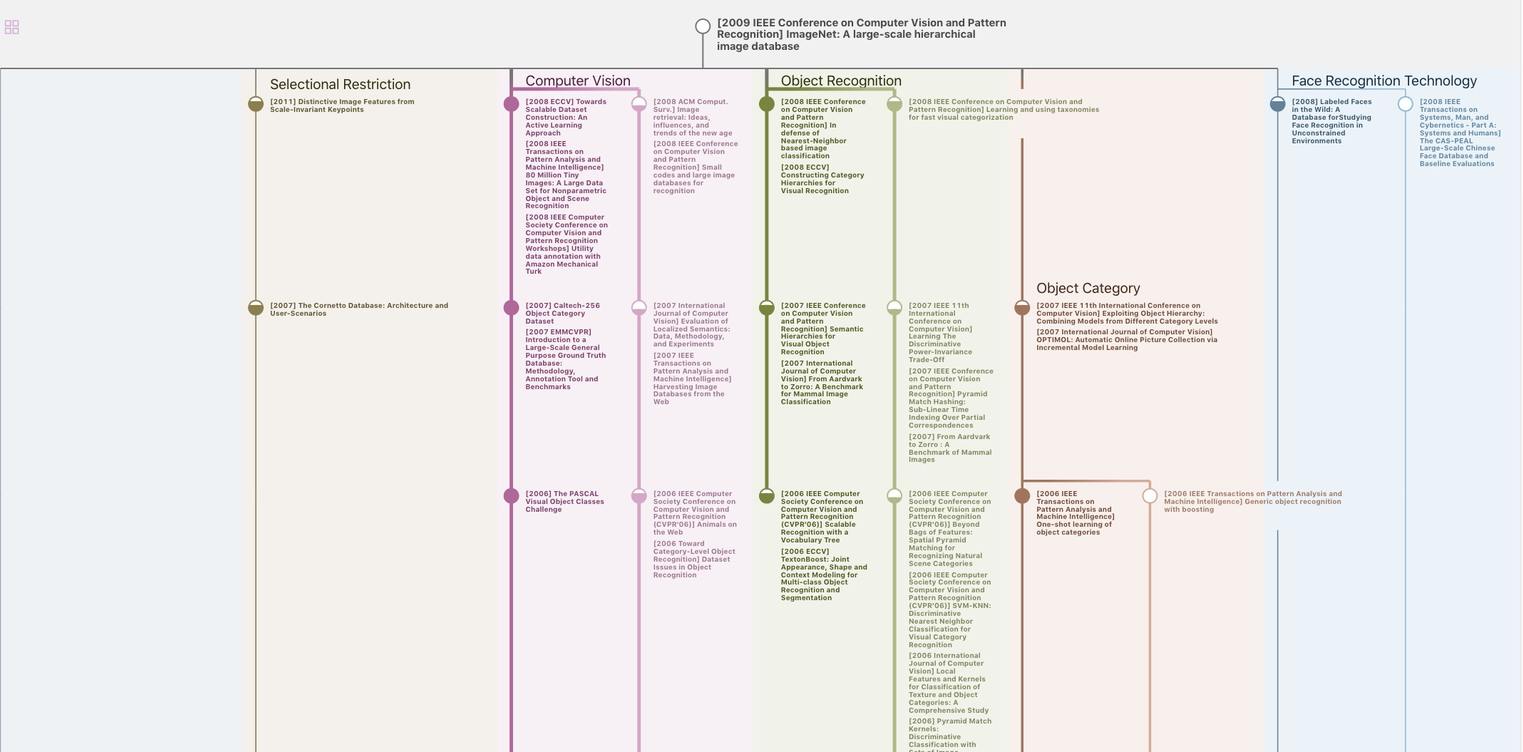
Generate MRT to find the research sequence of this paper
Chat Paper
Summary is being generated by the instructions you defined