Parameter estimation with the Markov Chain Monte Carlo method aided by evolutionary neural networks in a water hammer model
COMPUTATIONAL & APPLIED MATHEMATICS(2023)
摘要
Fast transients in pumps and valves may induce significant variations in pressures and flow rates throughout pipelines that can even cause structural damages. This computational work deals with parameter estimation of a water hammer model, with focus on a transient friction coefficient and an empirical parameter related to the pipeline elasticity. The hyperbolic water hammer model was solved with a total variation diminishing version of the weighted average flux finite volume scheme. Simulated pressure and flow rate measurements taken near the inlet and the outlet of the pipeline were used for the solution of the parameter estimation problem with Markov Chain Monte Carlo methods. This work aimed at the reduction of the computational time of the inverse problem solution with two different strategies: (1) the application of a recent parallel computation version of the Metropolis–Hastings algorithm; and (2) the use of a machine learning metamodel obtained with the evolutionary neural network algorithm and the approximation error model approach. These two approaches are compared in terms of the parameter estimation accuracies and associated computational times.
更多查看译文
关键词
Bayesian statistics,Metropolis-Hastings algorithm,Parallel computation,Machine learning,EvoNN,Approximation error model
AI 理解论文
溯源树
样例
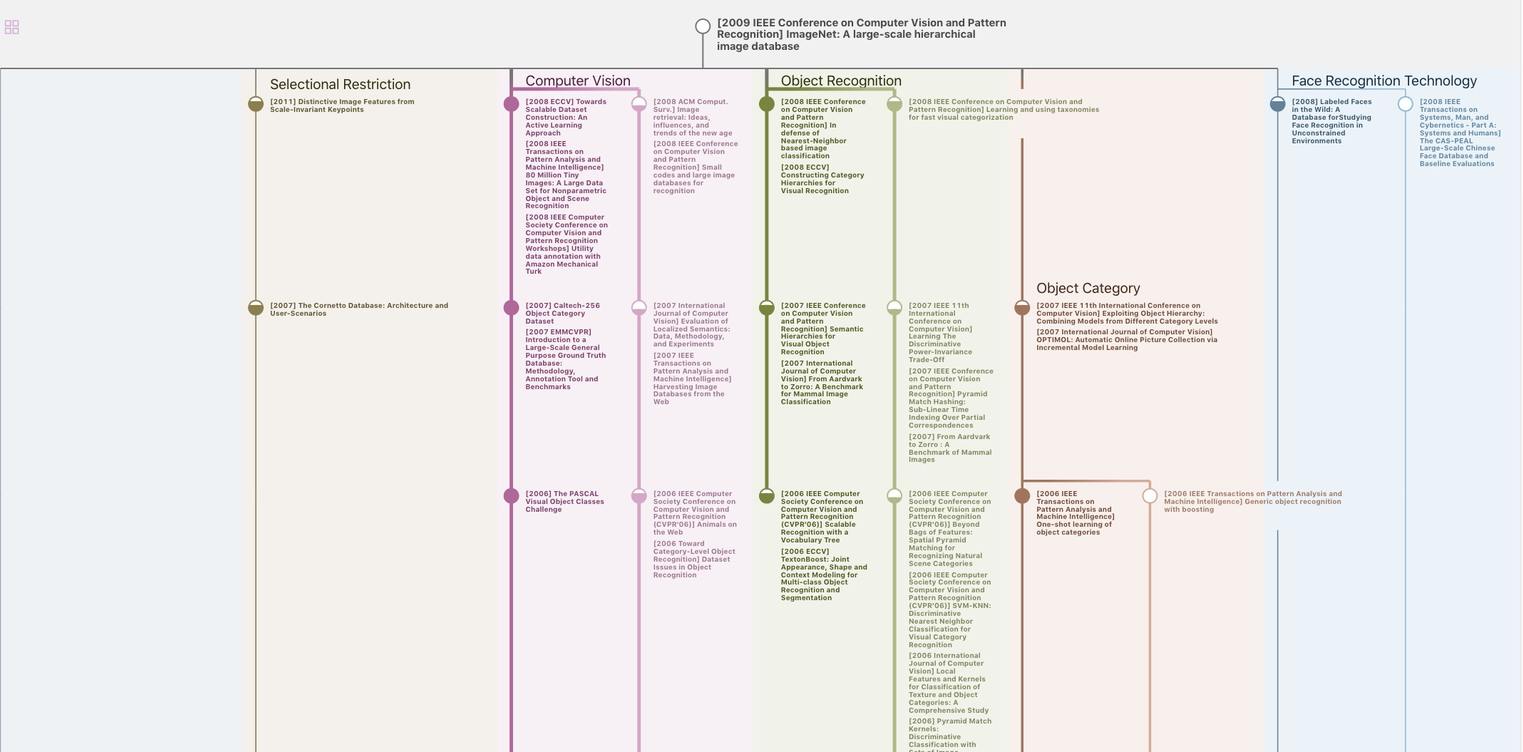
生成溯源树,研究论文发展脉络
Chat Paper
正在生成论文摘要