Application of self-attention conditional deep convolutional generative adversarial networks in the fault diagnosis of planetary gearboxes
PROCEEDINGS OF THE INSTITUTION OF MECHANICAL ENGINEERS PART O-JOURNAL OF RISK AND RELIABILITY(2024)
摘要
The Generative Adversarial Network (GAN) can generate samples similar to the original data to solve the problem of fault sample imbalance in planetary gearbox fault diagnosis. Most of models rely heavily on convolution to model the dependencies across feature vectors of vibration signals. However, the characterization ability of convolution operator is limited by the size of convolution kernel and it cannot capture the long-distance dependence in the original data. In this paper, self-attention is introduced into Conditional Deep Convolutional Generative Adversarial Networks (C-DCGAN). In the model, vibration features are dynamically weighted and merged, so that it can adaptively focus "attention" on different times to solve the problem of sample differences caused by time-varying vibration signals. Finally, the proposed method is verified on the planetary gearbox experiment and the quality of the generated signal samples is evaluated with Dynamic Time Warping (DTW) algorithm. The visual experimental results indicated that the proposed model performed better than conditional deep convolutional generative adversarial networks (C-DCGAN) and could accurately diagnose various working states of planetary gearboxes.
更多查看译文
关键词
Self-attention mechanism,generative adversarial networks,planetary gearboxes,fault diagnosis
AI 理解论文
溯源树
样例
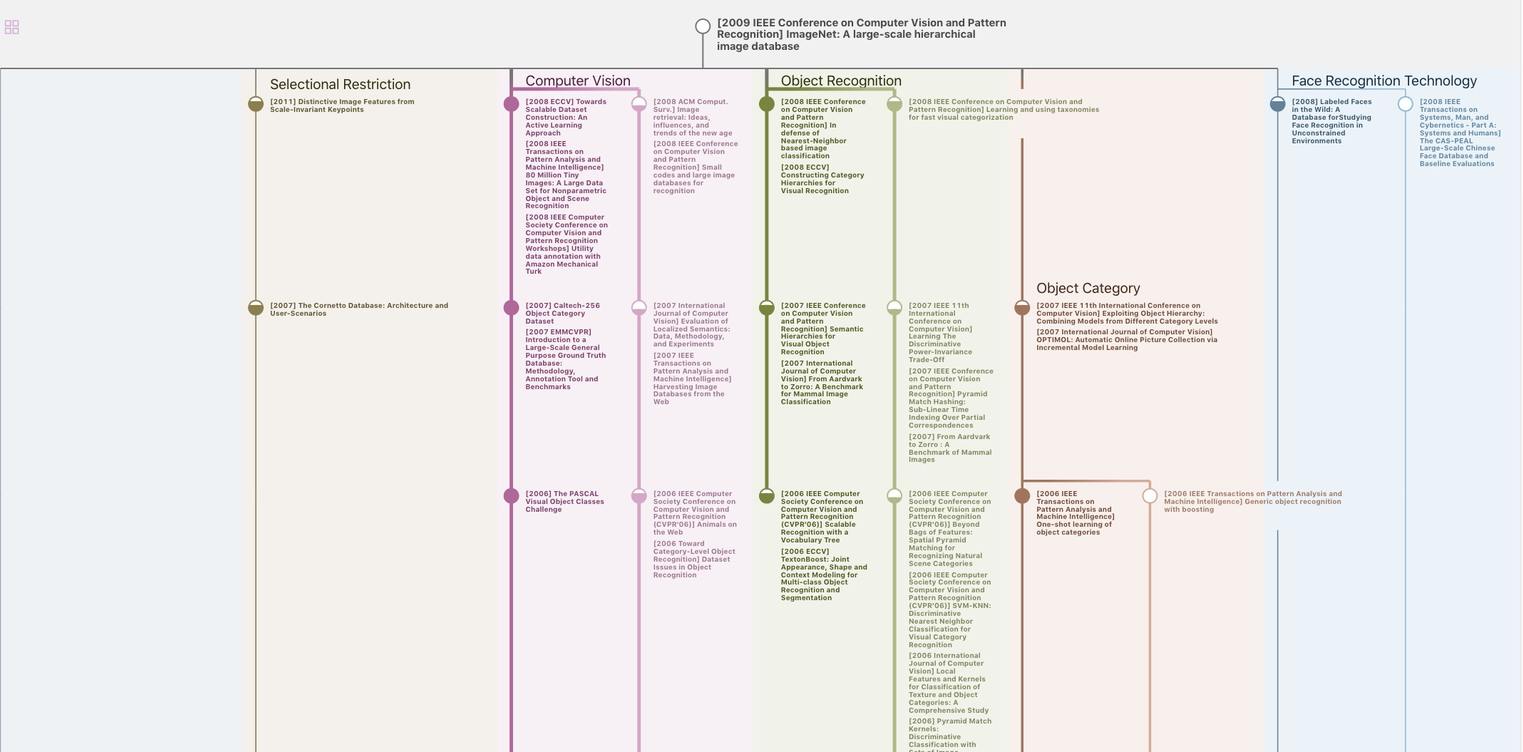
生成溯源树,研究论文发展脉络
Chat Paper
正在生成论文摘要