Grey wolf optimization (GWO) with the convolution neural network (CNN)-based pattern recognition system
IMAGING SCIENCE JOURNAL(2023)
摘要
The dynamic video frame dataset's automated feature analysis addresses the complexity of intensity mapping with normal and abnormal classes. Iterative modelling is needed to learn the component of a video frame in several patterns for various video frame data types for threshold-based data clustering and feature analysis. GWO optimises the Convoluted Pattern of Wavelet Transform (CPWT) feature vectors employed in this paper's CNN feature analysis technique. A median filter reduces noise and smooths the video frame before normalising it. Edge information represents the video frame's bright spot boundary. Neural network based video frame classification clusters pixels using feature recurrent learning with minimal dataset training. The filtered video frame's features were evaluated using complex wavelet transformation feature extraction algorithms. These features demonstrate video frame spatial and textural classifications. CNN classifiers help analyse video frame instances and classify action labels. Categorization improves with the fewest training datasets. This strategy may be beneficial if compared to optimal practises.
更多查看译文
关键词
Action recognition,pattern recognition system,convoluted pattern of wavelet transform (CPWT),grey wolf optimization (GWO),convolution neural network (CNN),Deep bidirectional long short-term memory (DBiLSTM),Deep Convolution Symmetric Neural Network with PCANET Weaklysupervisedaction localization (WSAL),UCF sports dataset
AI 理解论文
溯源树
样例
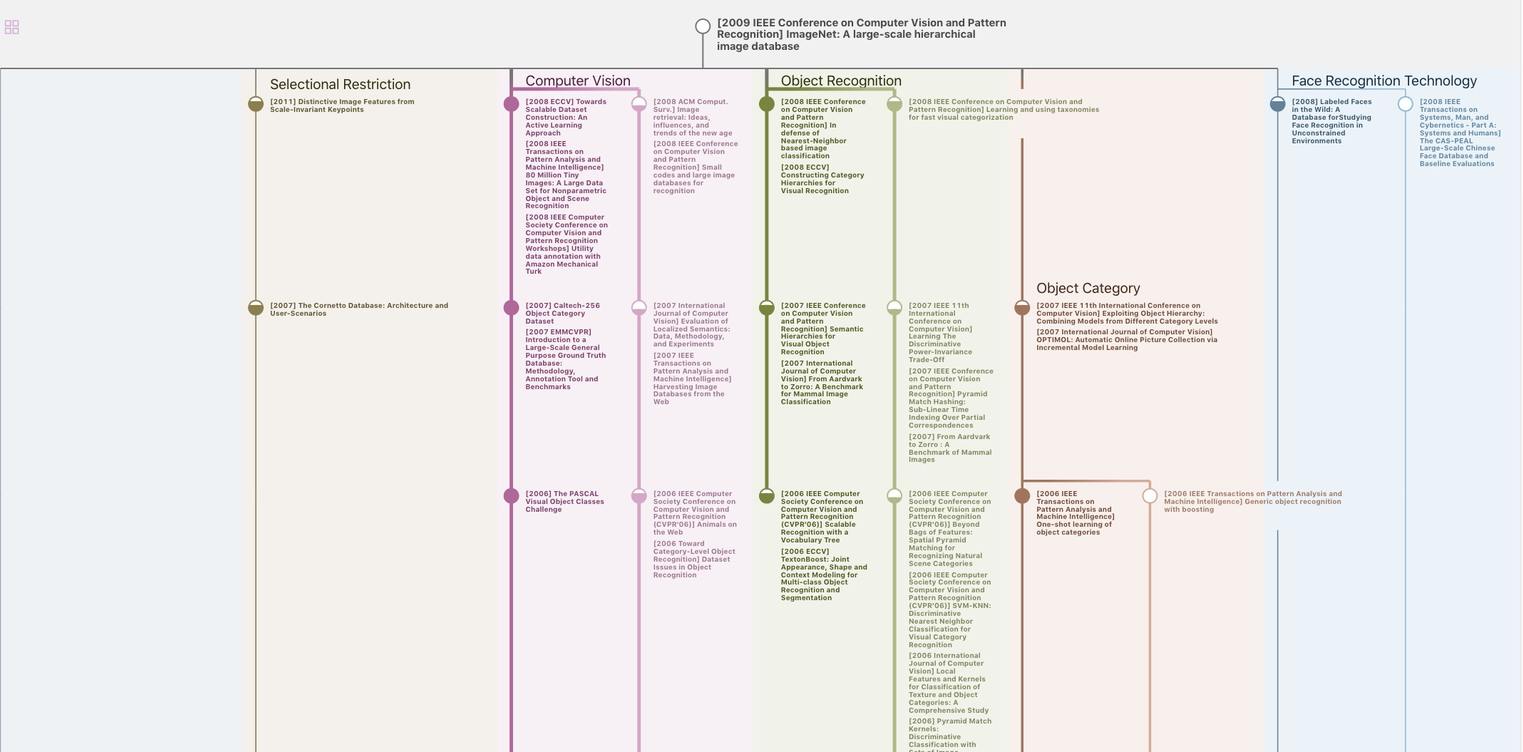
生成溯源树,研究论文发展脉络
Chat Paper
正在生成论文摘要