Breast Cancer Classification from Mammogram Images Using Extreme Learning Machine-Based DenseNet121 Model
JOURNAL OF SENSORS(2022)
Abstract
Breast cancer is characterized by abnormal discontinuities in the lining cells of a woman's milk duct. Large numbers of women die from breast cancer as a result of developing symptoms in the milk ducts. If the diagnosis is made early, the death rates can be decreased. For radiologists and physicians, manually analyzing mammography images for breast cancer become time-consuming. To prevent manual analysis and simplify the work of classification, this paper introduces a novel hybrid DenseNet121-based Extreme Learning Machine Model (ELM) for classifying breast cancer from mammogram images. The mammogram images were processed through preprocessing and data augmentation phase. The features were collected separately after the pooling and flatten layer at the first stage of the classification. Further, the features are fed as input to the proposed DenseNet121-ELM model's fully connected layer as input. An extreme learning machine model has replaced the fully connected layer. The weights of the extreme learning machine have been updated by the AdaGrad optimization algorithm to increase the model's robustness and performance. Due to its faster convergence speed than other optimization techniques, the AdaGrad algorithm optimization was chosen. In this research, the Digital Database for Screening Mammography (DDSM) dataset mammogram images were utilized, and the results are presented. We have considered the batch size of 32, 64, and 128 for the performance measure, accuracy, sensitivity, specificity, and computational time. The proposed DenseNet121+ELM model achieves 99.47% and 99.14% as training accuracy and testing accuracy for batch size 128. Also, it achieves specificity, sensitivity, and computational time of 99.37%, 99.94%, and 159.7731 minutes, respectively. Further, the comparison result of performance measures is presented for batch sizes 32, 64, and 128 to show the robustness of the proposed DenseNet121+ELM model. The automatic classification performance of the DenseNet121+ELM model has much potential to be applied to the clinical diagnosis of breast cancer.
MoreTranslated text
Key words
mammogram images,breast cancer,classification,model,machine-based
AI Read Science
Must-Reading Tree
Example
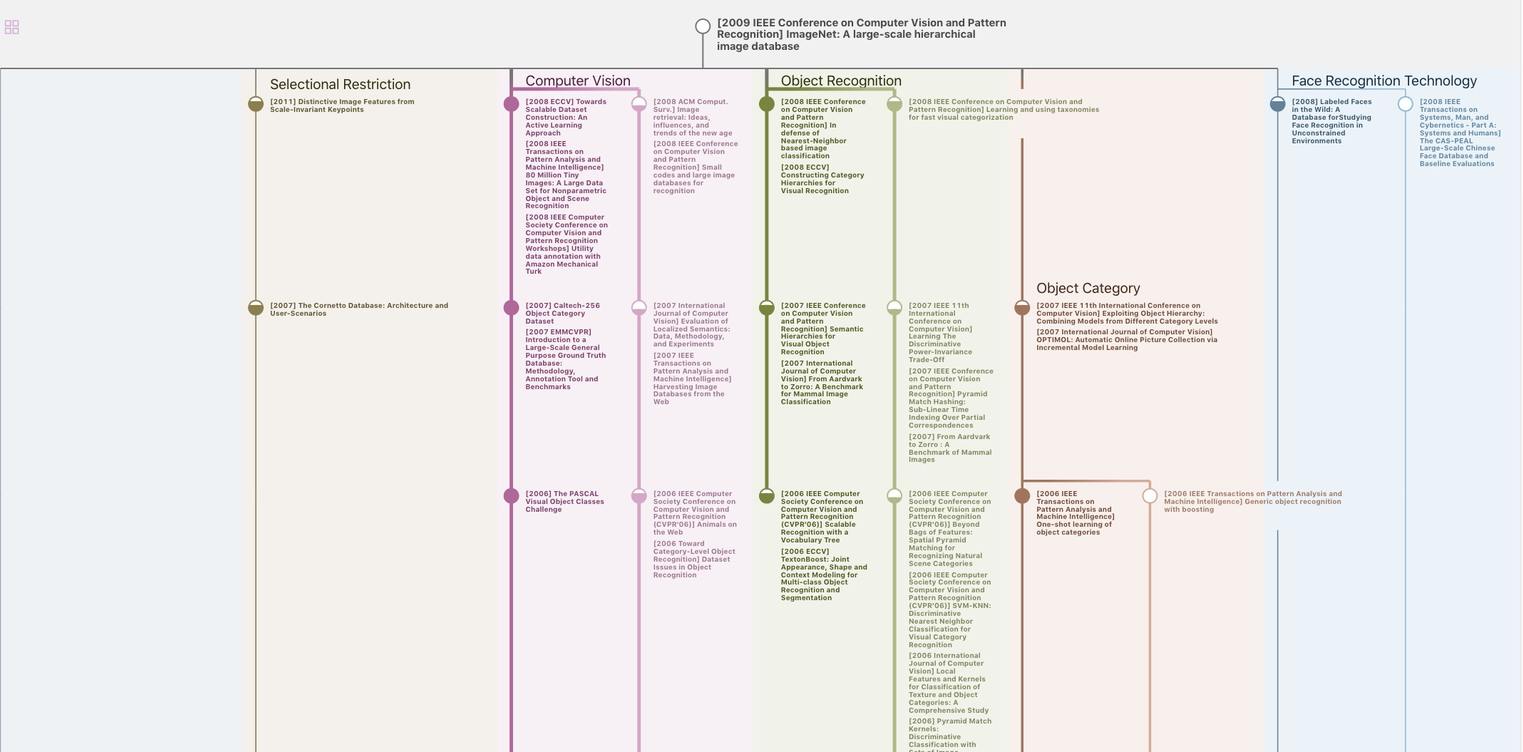
Generate MRT to find the research sequence of this paper
Chat Paper
Summary is being generated by the instructions you defined