Predicting melt track geometry and part density in laser powder bed fusion of metals using machine learning
PROGRESS IN ADDITIVE MANUFACTURING(2023)
摘要
Laser powder bed fusion of metals (PBF-LB/M) is a process widely used in additive manufacturing (AM). It is highly sensitive to its process parameters directly determining the quality of the components. Hence, optimal parameters are needed to ensure the highest part quality. However, current approaches such as experimental investigation and the numerical simulation of the process are time-consuming and costly, requiring more efficient ways for parameter optimization. In this work, the use of machine learning (ML) for parameter search is investigated based on the influence of laser power and speed on simulated melt pool dimensions and experimentally determined part density. In total, four machine learning algorithms are considered. The models are trained to predict the melt pool size and part density based on the process parameters. The accuracy is evaluated based on the deviation of the prediction from the actual value. The models are implemented in python using the scikit-learn library. The results show that ML models provide generalized predictions with small errors for both the melt pool dimensions and the part density, demonstrating the potential of ML in AM. The main limitation is data collection, which is still done experimentally or simulatively. However, the results show that ML provides an opportunity for more efficient parameter optimization in PBF-LB/M.
更多查看译文
关键词
Ti-6Al-4V,Parameter optimization,Laser powder bed fusion of metals PBF-LB/M,Machine learning
AI 理解论文
溯源树
样例
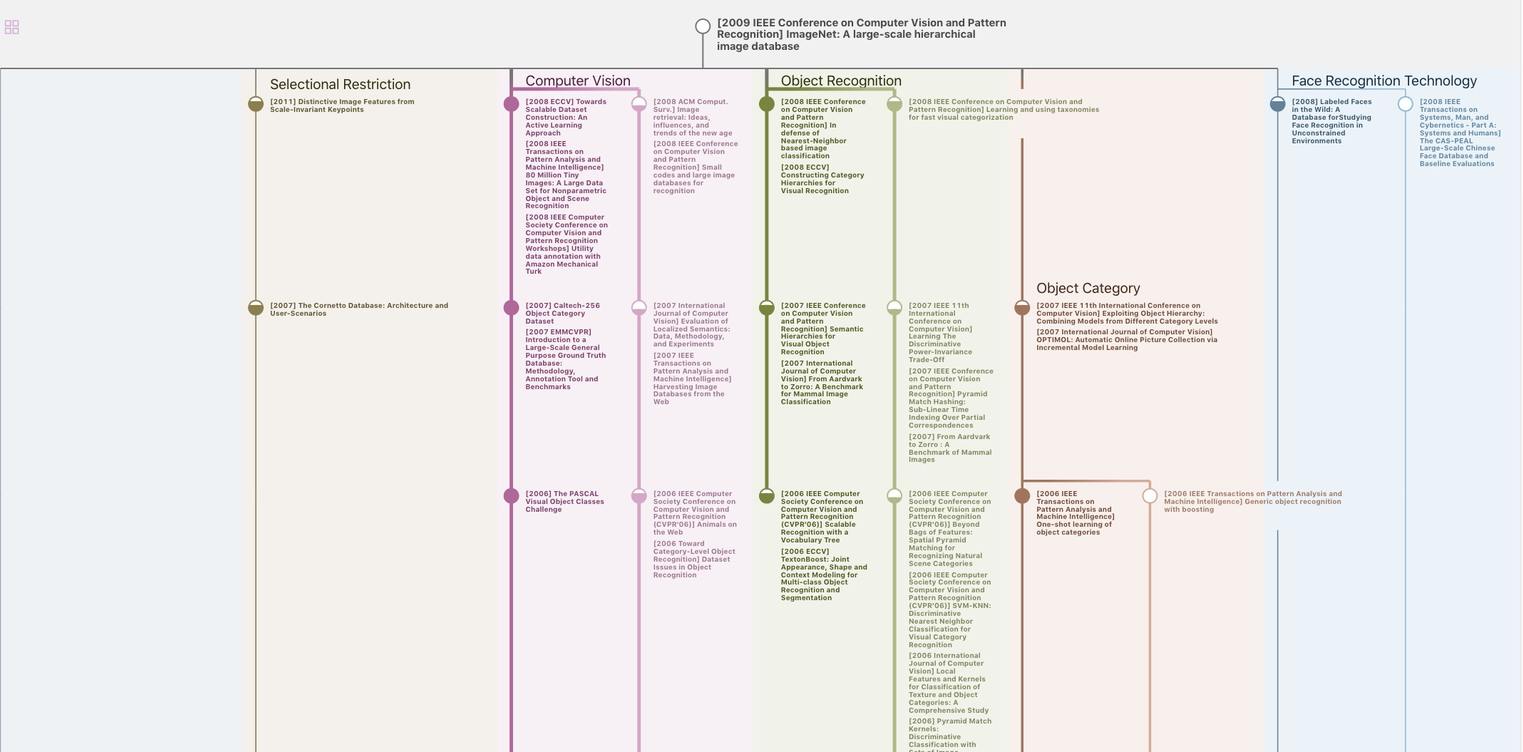
生成溯源树,研究论文发展脉络
Chat Paper
正在生成论文摘要