Bridging the Gap Between Artificial Neural Networks and Kernel Regressions for Vector-Valued Problems in Microwave Applications
IEEE TRANSACTIONS ON MICROWAVE THEORY AND TECHNIQUES(2023)
摘要
Thanks to their convex formulation, kernel regressions have shown an improved accuracy with respect to artificial neural network (ANN) structures in regression problems where a reduced set of training samples are available. However, despite the above interesting features, kernel regressions are inherently less flexible than ANN structures since their implementations are usually limited to scalar-output regression problems. This article presents a vector-valued (multioutput) formulation of the kernel ridge regression (KRR) aimed at bridging the gap between multioutput ANN structures and scalar kernel-based approaches. The proposed vector-valued KRR relies on a generalized definition of the reproducing kernel Hilbert space (RKHS) and on a new multioutput kernel structure. The mathematical background of the proposed vector-valued formulation is extensively discussed together with different matrix kernel functions and training schemes. Moreover, a compression strategy based on the Nystrom approximation is presented to reduce the computational complexity of the model training. The effectiveness and the performance of the proposed vector-valued KRR are discussed on an illustrative example consisting of a high-speed link and on the optimization of a Doherty amplifier.
更多查看译文
关键词
Kernel,kernel ridge regression (KRR),microwave structures,optimization,parametric modeling,repro-ducing kernel Hilbert space (RKHS),vector-valued kernel regression
AI 理解论文
溯源树
样例
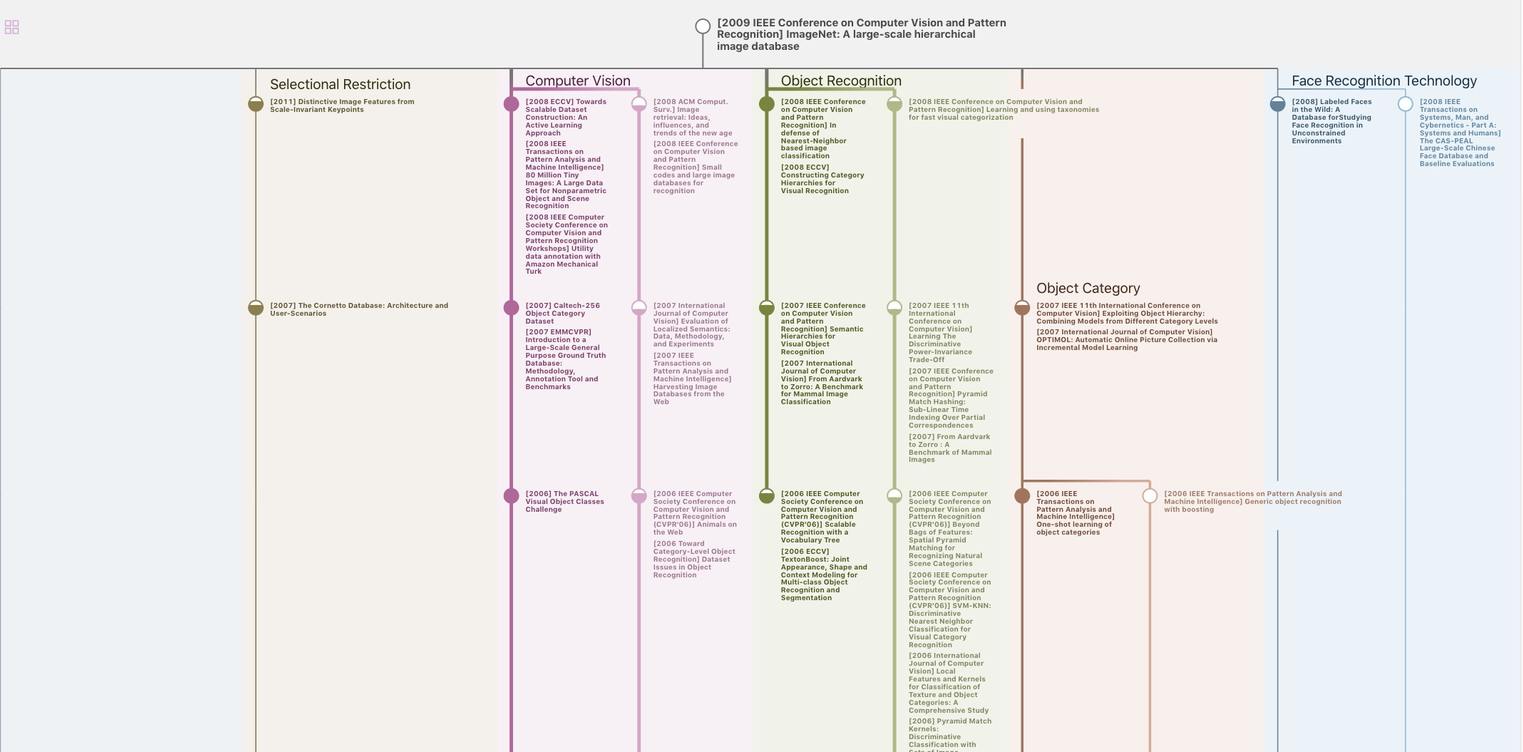
生成溯源树,研究论文发展脉络
Chat Paper
正在生成论文摘要