Short term photovoltaic power prediction based on transfer learning and considering sequence uncertainty
JOURNAL OF RENEWABLE AND SUSTAINABLE ENERGY(2023)
摘要
With the increasing proportion of solar grid-connected, the establishment of an accurate photovoltaic (PV) power prediction model is very important for safe operation and efficient dispatching of a power grid. Considering the multi-level periodicity of PV power caused by many factors, such as seasons and weather, a short-term PV power prediction model based on transfer component analysis is designed by introducing the idea of transfer learning. In order to measure the uncertainty of numerical weather prediction (NWP) and power sequence, a novel algorithm considering weather similarity and power trend similarity is proposed. First, the intrinsic trend is measured by extracting permutation entropy, variance, and mean from the historical PV power sequence. Second, weighting of NWP is accomplished based on the Pearson correlation coefficient. PV power data are divided into different clusters by K-medoids clustering. At the same time, the transfer component analysis alleviates the time-varying problem of data distribution caused by multi-level time periodicity and effectively improves the prediction accuracy of the model. Finally, simulation experiments are carried out on the PV power output dataset (PVOD). The results show that the prediction accuracy of the proposed method is better than the traditional methods, and the accuracy and applicability of the proposed method are verified.
更多查看译文
关键词
photovoltaic,transfer learning,prediction
AI 理解论文
溯源树
样例
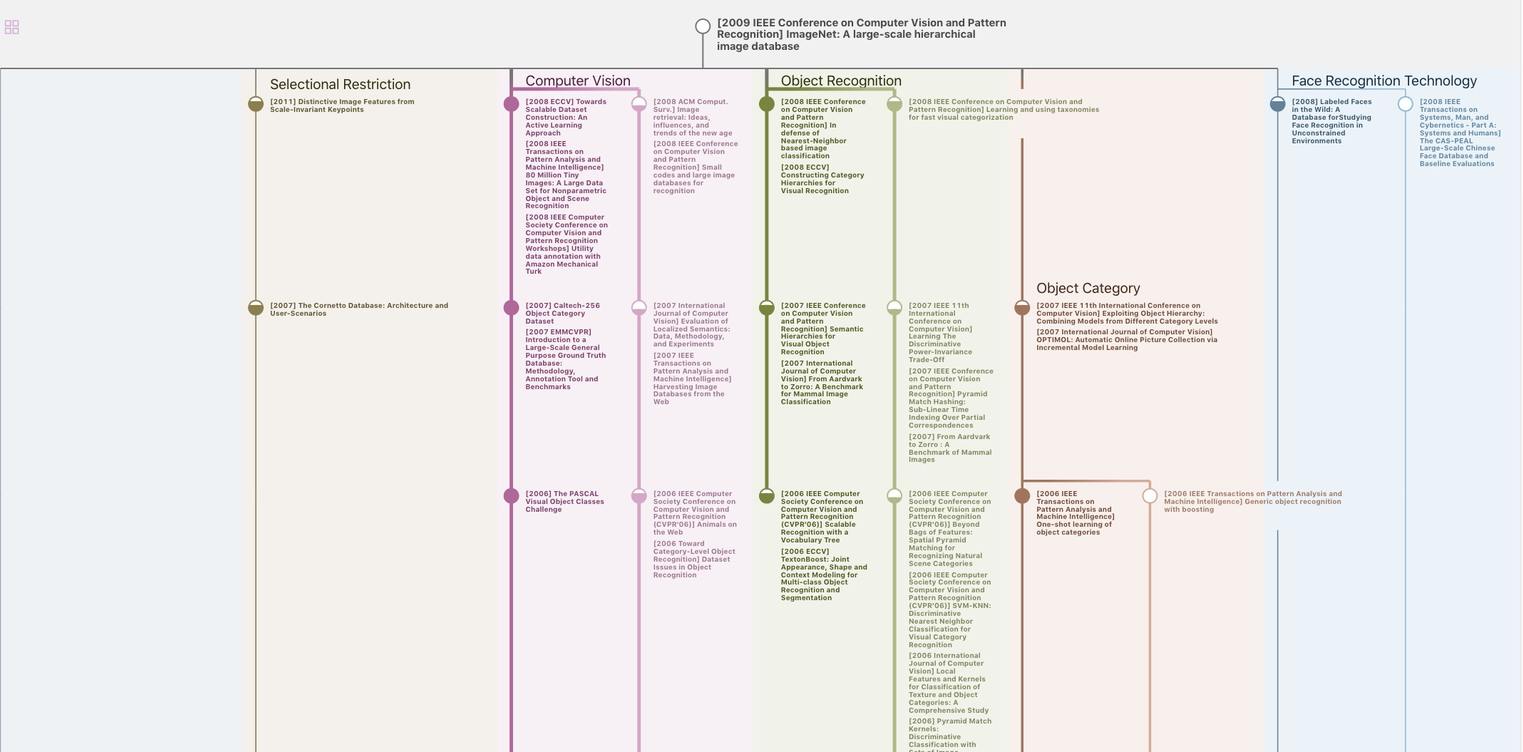
生成溯源树,研究论文发展脉络
Chat Paper
正在生成论文摘要