Session-Based Recommendations for e-Commerce with Graph-Based Data Modeling
APPLIED SCIENCES-BASEL(2023)
摘要
Conventional recommendation methods such as collaborative filtering cannot be applied when long-term user models are not available. In this paper, we propose two session-based recommendation methods for anonymous browsing in a generic e-commerce framework. We represent the data using a graph where items are connected to sessions and to each other based on the order of appearance or their co-occurrence. In the first approach, called Hierarchical Sequence Probability (HSP), recommendations are produced using the probabilities of items' appearances on certain structures in the graph. Specifically, given a current item during a session, to create a list of recommended next items, we first compute the probabilities of all possible sequential triplets ending in each candidate's next item, then of all candidate item pairs, and finally of the proposed item. In our second method, called Recurrent Item Co-occurrence (RIC), we generate the recommendation list based on a weighted score produced by a linear recurrent mechanism using the co-occurrence probabilities between the current item and all items. We compared our approaches with three state-of-the-art Graph Neural Network (GNN) models using four session-based datasets one of which contains data collected by us from a leather apparel e-shop. In terms of recommendation effectiveness, our methods compete favorably on a number of datasets while the time to generate the graph and produce the recommendations is significantly lower.
更多查看译文
关键词
recommender systems,session-based recommendations,e-commerce,data and web mining,item co-occurrence,graph data model
AI 理解论文
溯源树
样例
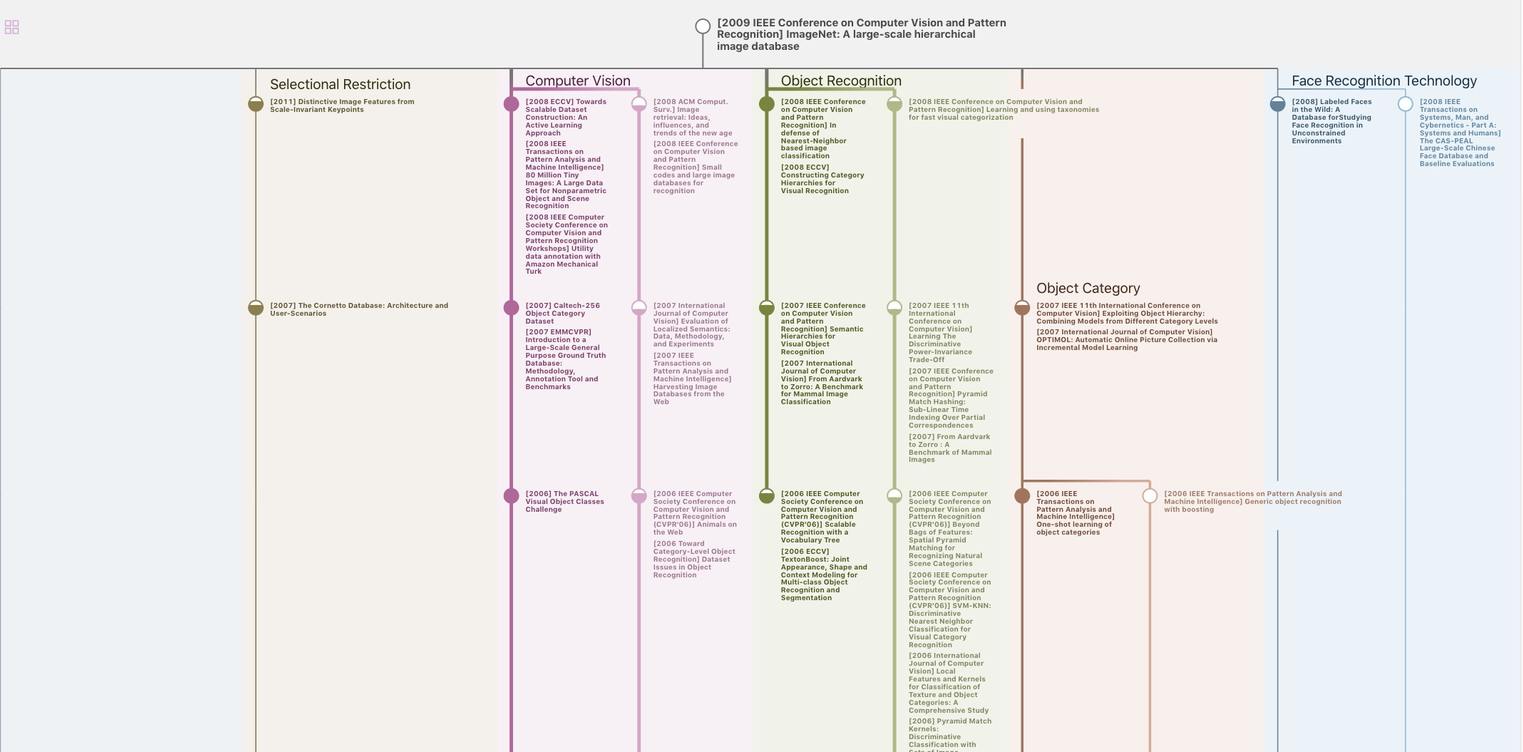
生成溯源树,研究论文发展脉络
Chat Paper
正在生成论文摘要