Investigating machine learning models to predict microbial activity during ozonation-biofiltration
ENVIRONMENTAL SCIENCE-WATER RESEARCH & TECHNOLOGY(2023)
摘要
Continuous online monitoring of water treatment process performance is an essential step in ensuring reliable water quality outcomes. In particular, it is important to ensure effective removal of microbial substances during advanced wastewater treatment processes. However, most microbial indicators cannot be continuously monitored by online processes. Therefore, it is necessary to monitor treatment process performance based on surrogate measures which can be reliably and continuously monitored. For example, water quality data such as colour, turbidity and chemical oxygen demand (COD) can be measured quickly and easily. In this study, a combined ozonation-biological media filtration process (O-3/BMF), was used to reduce microbial indicator concentration. After gathering water quality data and corresponding microbial indicator concentrations, we applied machine learning to develop models for predicting the amount of change in microbial indicator concentration following O-3/BMF treatment. Three microbial indicators were studied, namely Clostridium perfringens, E. coli, and somatic coliphage. The most effective physico-chemical predictors for the removal of these microbial indicators were determined by means of mutual information. Associations between changes in the predictors' concentration during O-3/BMF and the reduction of the microbial indicators were identified using a range of supervised learning algorithms including Naive Bayes, random forest, support vector machines and generalised linear model. The impact of the type of prediction algorithm on prediction accuracy was investigated and the superior classifier was determined. Performance measures for microbial removal prediction were found to be superior for the support vector machines (SVM) classifier. Using SVM with a Gaussian kernel classifier, prediction accuracy for all microbial removal was above 75%. Moreover, other performance measures such as area under curve (AUC) and kappa statistics (KS) were higher in SVM compared to the other applied classifiers (AUC >= 0.80; KS >= 0.34). From this study, we have identified an objective and efficient method that can predict the effectiveness of the O-3/BMF process in removing the three microbial indicators in water from a short list of commonly measured physico-chemical parameters.
更多查看译文
关键词
ozonation–biofiltration,microbial activity,machine learning models,machine learning,ozonation–biofiltration
AI 理解论文
溯源树
样例
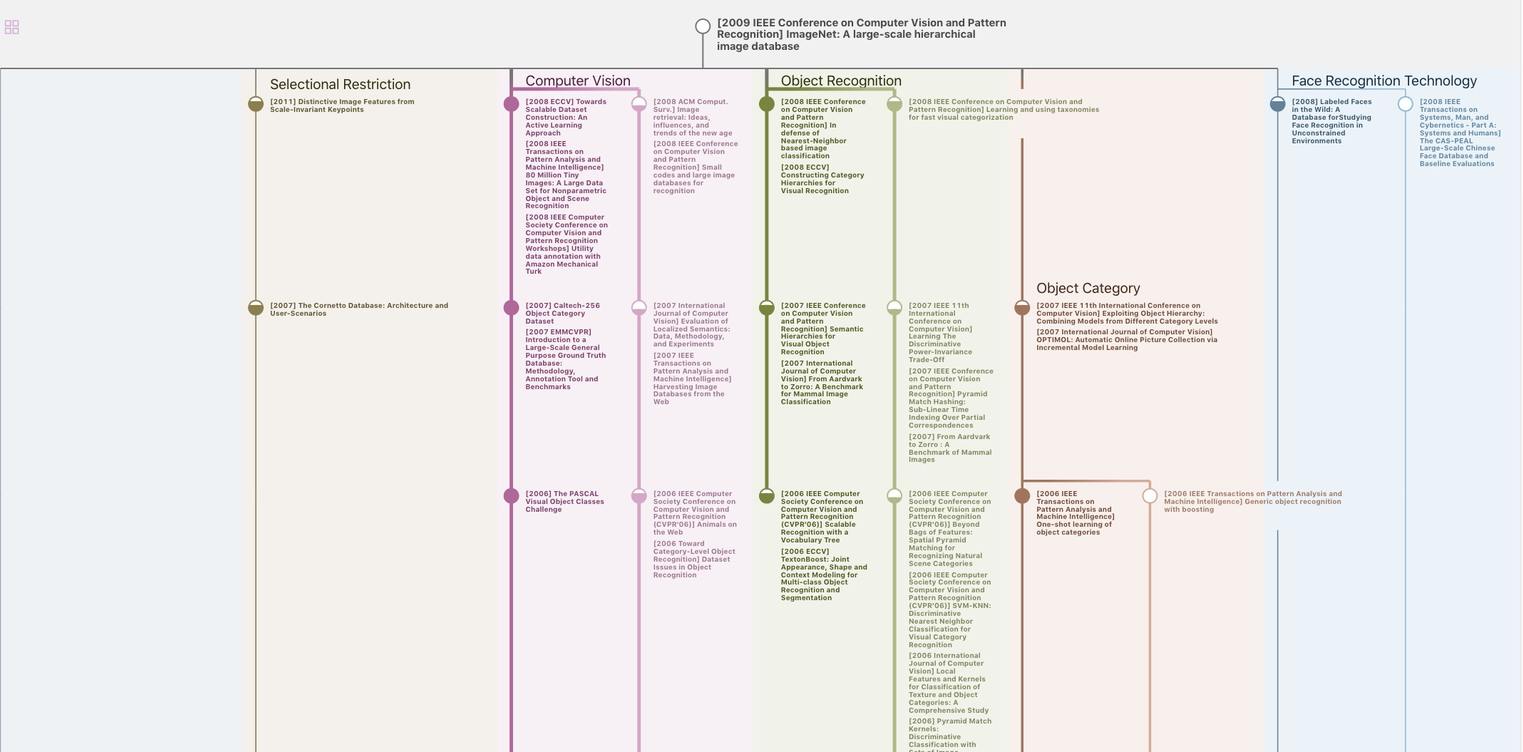
生成溯源树,研究论文发展脉络
Chat Paper
正在生成论文摘要