Estimation of crash type frequency accounting for misclassification in crash data.
Accident; analysis and prevention(2023)
摘要
Crash misclassification (MC) - e.g., a crash of one type or severity being mistakenly miscategorized as another - is a relatively common problem in transportation safety. Crash frequency models for individual crash categories estimated using datasets with MC errors could result in biased parameter estimates and thus lead to ineffective countermeasure planning. This study proposes a novel methodological formulation to directly account for this MC error and incorporates it into the two most common count data models used for crash frequency prediction: Poisson and Negative Binomial (NB) regression. The proposed framework introduces probabilistic MC rates among different crash types and modifies the likelihood function of the count models accordingly. The paper also demonstrates how this approach can be integrated into reformulated models that express each count model as a discrete choice model. The capability of the proposed models to estimate true parameters, given the existence of MC error, is examined via simulation analysis. Then, the proposed models are applied to empirical data to examine the presence of MC in crash data and further examine the robustness of the proposed models. Although the MC rates are found to be very low in the empirical data, the fit of proposed models are found to be better compared to the models that ignore MC error and thus likely provide more reliable parameter estimates.
更多查看译文
关键词
Count model,Crash data,Crash frequency,Misclassification,Reformulated count model
AI 理解论文
溯源树
样例
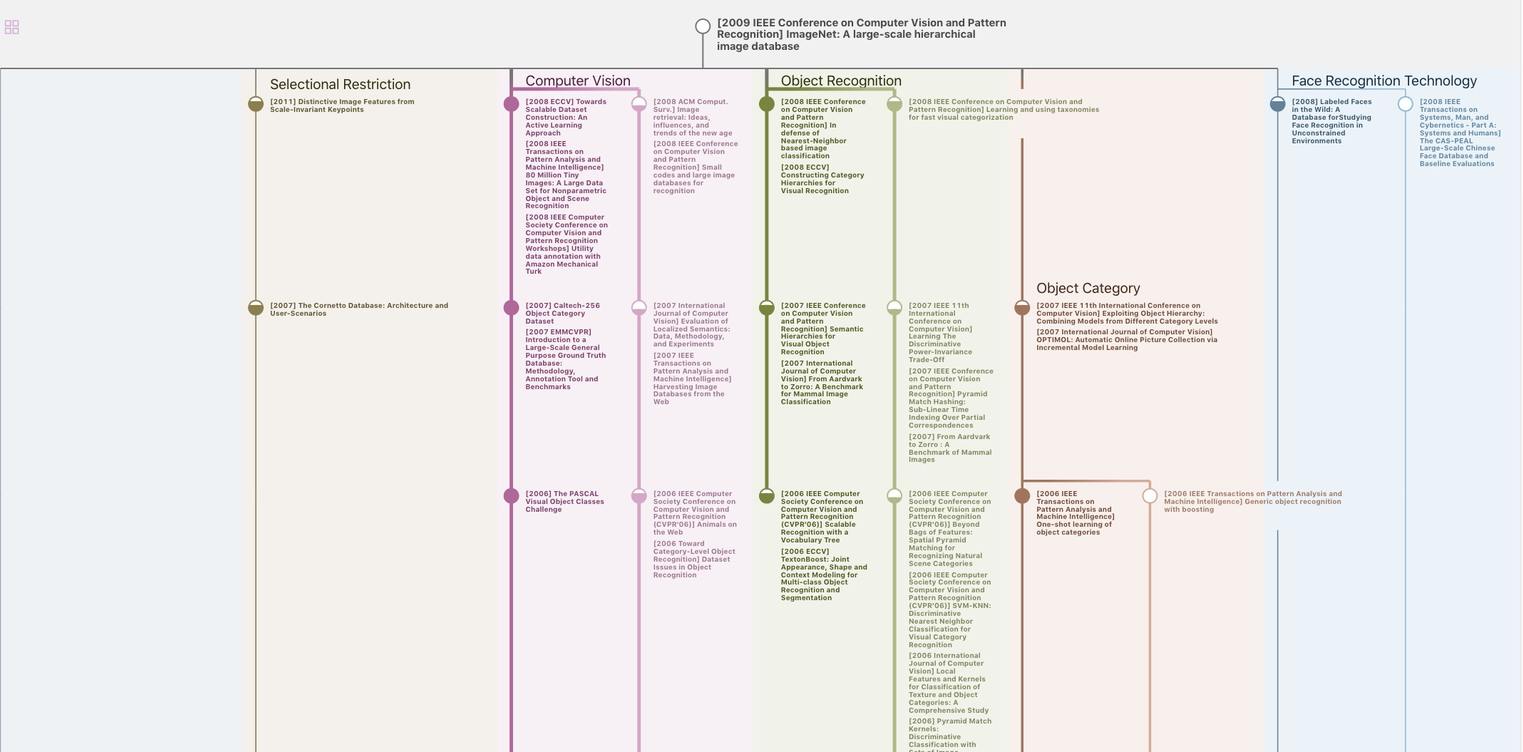
生成溯源树,研究论文发展脉络
Chat Paper
正在生成论文摘要