A multitask approach for automated detection and segmentation of thyroid nodules in ultrasound images
COMPUTERS IN BIOLOGY AND MEDICINE(2024)
摘要
An increase in the incidence and diagnosis of thyroid nodules and thyroid cancer underscores the need for a better approach to nodule detection and risk stratification in ultrasound (US) images that can reduce healthcare costs, patient discomfort, and unnecessary invasive procedures. However, variability in ultrasound technique and interpretation makes the diagnostic process partially subjective. Therefore, an automated approach that detects and segments nodules could improve performance on downstream tasks, such as risk stratification. Ultrasound studies were acquired from 280 patients at UCLA Health, totaling 9888 images, and annotated by collaborating radiologists. Current deep learning architectures for segmentation are typically semi-automated because they are evaluated solely on images known to have nodules and do not assess ability to identify suspicious images. However, the proposed multitask approach both detects suspicious images and segments potential nodules; this allows for a clinically translatable model that aptly parallels the workflow for thyroid nodule assessment. The multitask approach is centered on an anomaly detection (AD) module that can be integrated with any UNet architecture variant to improve image-level nodule detection. Of the evaluated multitask models, a UNet with a ImageNet pretrained encoder and AD achieved the highest F1 score of 0.839 and image-wide Dice similarity coefficient of 0.808 on the hold-out test set. Furthermore, models were evaluated on two external validations datasets to demonstrate generalizability and robustness to data variability. Ultimately, the proposed architecture is an automated multitask method that expands on previous methods by successfully both detecting and segmenting nodules in ultrasound.
更多查看译文
关键词
Segmentation,Anomaly detection,Thyroid nodules,Ultrasound,Multitask
AI 理解论文
溯源树
样例
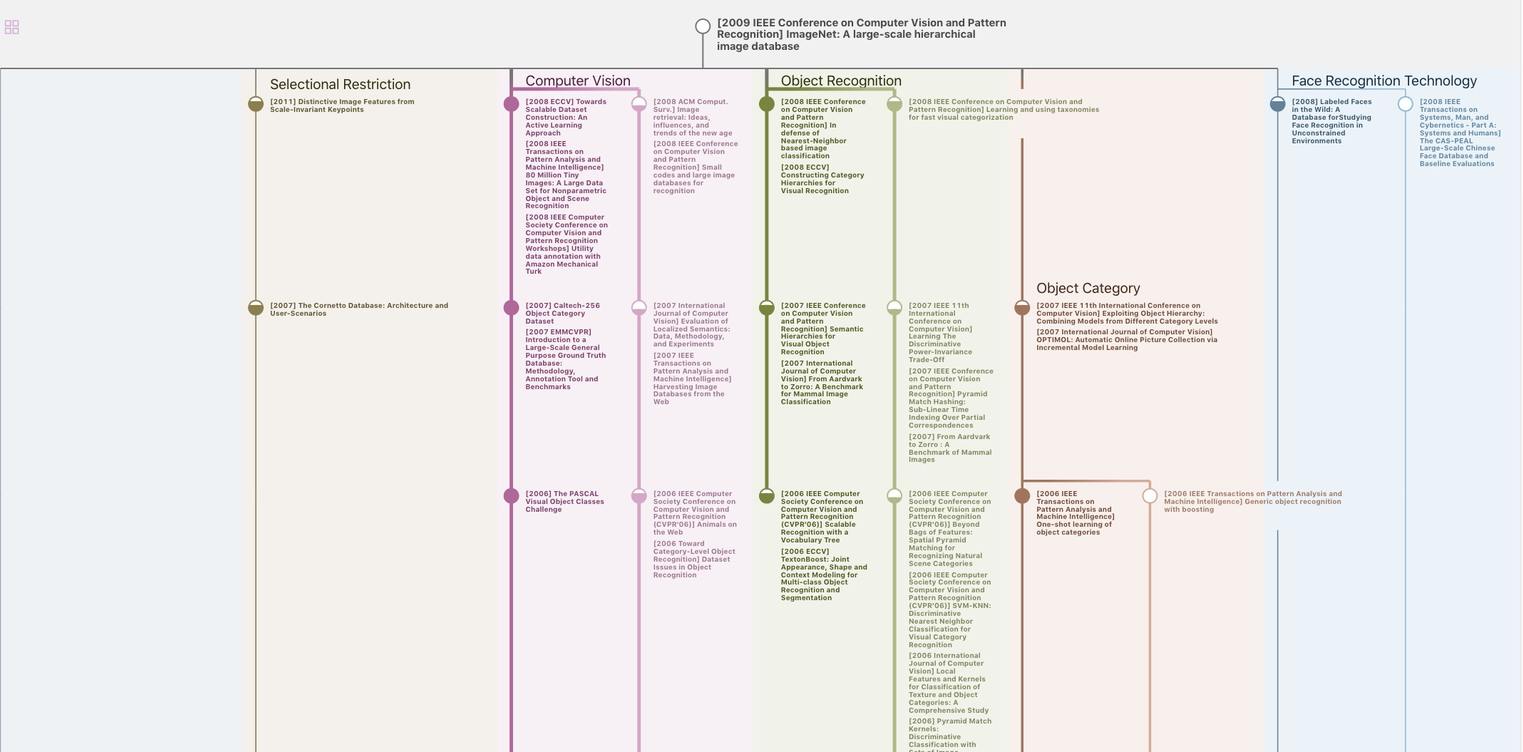
生成溯源树,研究论文发展脉络
Chat Paper
正在生成论文摘要