Multi-task learning framework for echocardiography segmentation
2022 IEEE INTERNATIONAL ULTRASONICS SYMPOSIUM (IEEE IUS)(2022)
摘要
Automatic delineation of cardiac structure in echocardiography (echo) is of significant importance in the diagnosis and prognosis of cardiovascular diseases (CVDs). Although numerous convolutional neural network (CNN) models have been developed for this purpose, they mainly focused on multi-structure segmentation or single-structure segmentation. However, there are some cases whereby only a specific structure needs to be analyzed, and training multiple deep learning models to achieve multiple single structure segmentation tasks is time-consuming and involves many complex training steps. In this paper, we investigate the feasibility of training a single CNN to achieve multiple cardiac structure segmentation tasks. Specifically, we propose a multitask learning (MTL) framework to segment left ventricle (LV), LV wall and LV+LV wall, simultaneously. It is worth noting that this is the first attempt to consider the segmentation of LV+LV wall as an auxiliary task to enhance the performance of LV and LV wall segmentation, respectively. Moreover, besides multi-structure segmentation, no study has investigated the individual segmentation of LV and LV wall using a single CNN model. The advantages of our proposed framework are assessed through conducting extensive segmentation experiments with two state-of-the-art segmentation models: UNet and DeeplabV3. The obtained results indicate that better performance can be achieved using a single CNN model to delineate multiple cardiac structures simultaneously.
更多查看译文
关键词
Echocardiography,deep learning,multi-task learning,cardiac structure segmentation
AI 理解论文
溯源树
样例
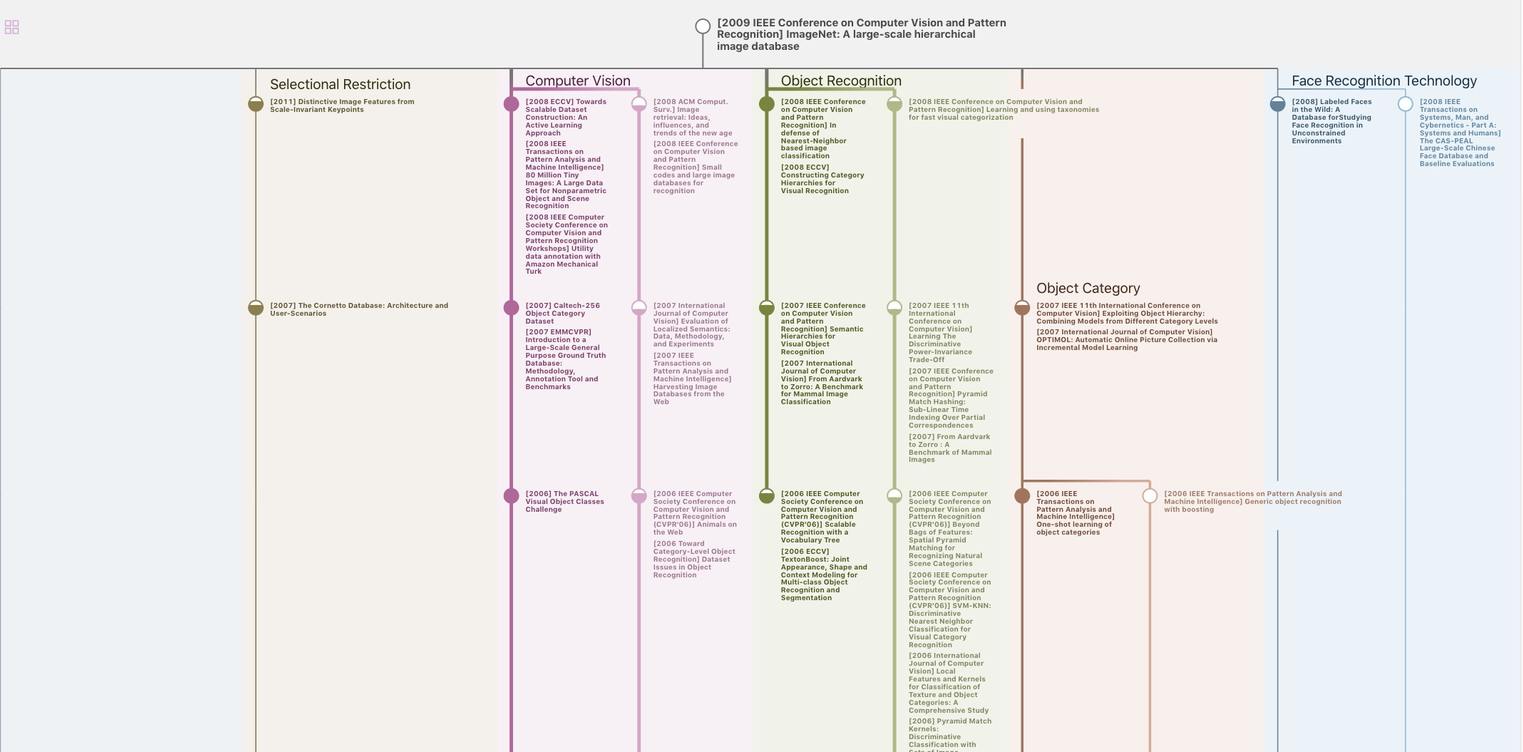
生成溯源树,研究论文发展脉络
Chat Paper
正在生成论文摘要