Interface Detector with online adaptive learning for anomaly detection
sciencepaper_online
摘要
Abstract: This paper describes an enhanced negative selection algorithm called interface detector, which is based on the boundary samples. There are two advantages of interface detector. On one hand, it can fully separate the self subspace from the non-self subspace with an appropriate self radius theoretically; on the other hand, it is able to learn during the testing stage, to adapt real-time variety of self subspace. Experiments are carried out using both 2-dimensional datasets and Iris dataset. The former was designed to demonstrate intuitively the online adaptive learning process of interface detector, while the latter was to show the detection ability of the interface detector with online adaptive learning. The experiments show that the proposed interface detector with online adaptive learning can achieve the higher detection rate and the lower false alarm rate in some cases, even if only one self sample is used for training.
更多查看译文
关键词
Artificial immune system,Negative selection algorithm,Anomaly detection,Interface detector,Online adaptive learning
AI 理解论文
溯源树
样例
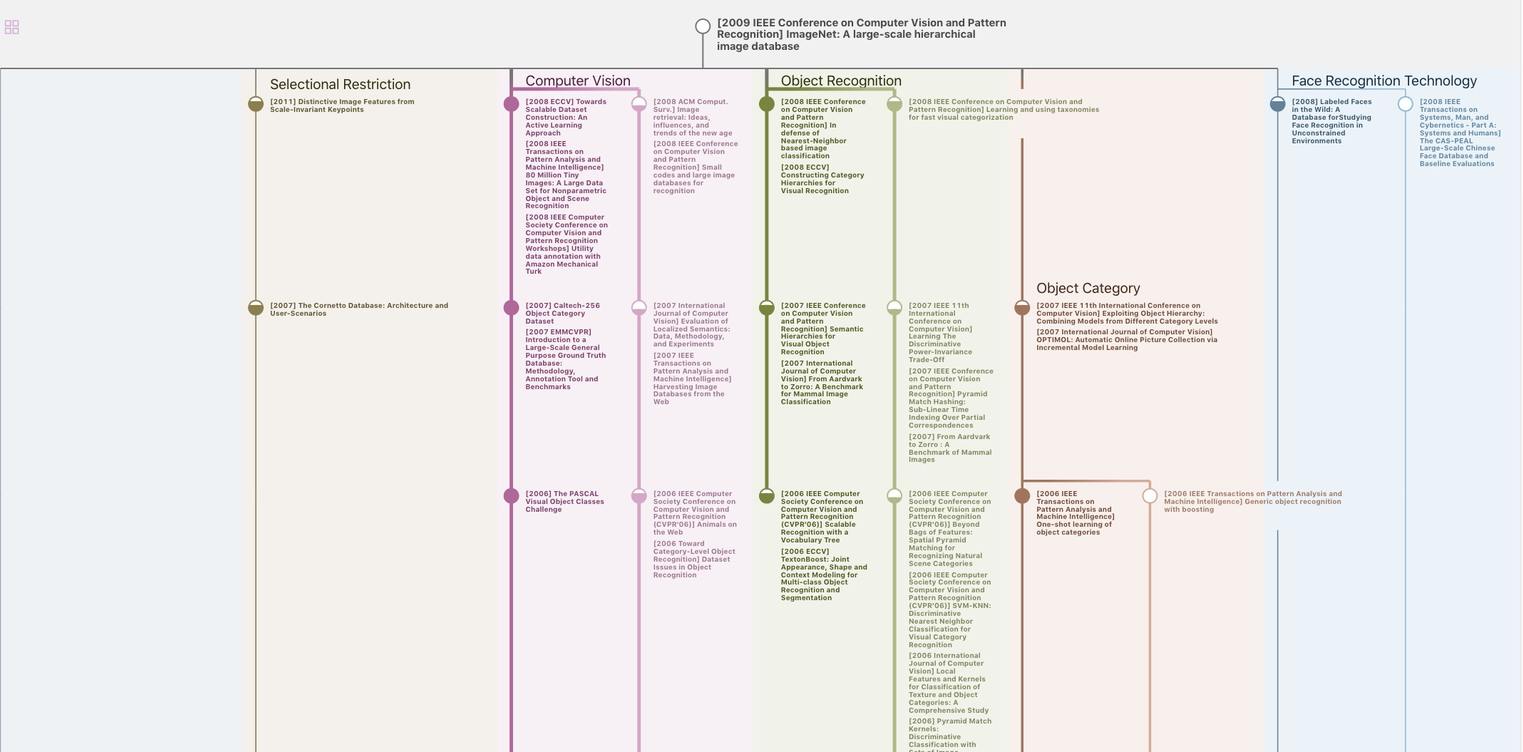
生成溯源树,研究论文发展脉络
Chat Paper
正在生成论文摘要