Feature-Contrastive Graph Federated Learning: Responsible AI in Graph Information Analysis
IEEE TRANSACTIONS ON COMPUTATIONAL SOCIAL SYSTEMS(2023)
摘要
Federated learning enables multiple clients to learn a general model without sharing local data, and the federated learning system also improves information security and advances responsible artificial intelligence (AI). However, the data of different clients in the system are non-independently and identically distributed (IID), which results in weight divergence, especially for complex graph data extraction. This article proposes a novel feature-contrastive graph federated (FcgFed) learning approach to improve the robustness of the federated learning system in graph data. First, we design an architecture for FcgFed learning systems to analyze graph information. Furthermore, we present a graph federated learning method based on contrastive learning to alleviate the weight divergence in federated learning. The experiments in node classification and graph classification demonstrate that our method achieves better performance than model-contrastive federated learning (MOON) and federated average (FedAvg). We also test the adaptability of our method in image classification, and the results demonstrate that weight similarity evaluation works for other frameworks and tasks.
更多查看译文
关键词
Federated learning,Data models,Artificial intelligence,Graph neural networks,Training,Learning systems,Servers,graph neural networks,responsible artificial intelligence (AI),weight divergence,weight similarity evaluation
AI 理解论文
溯源树
样例
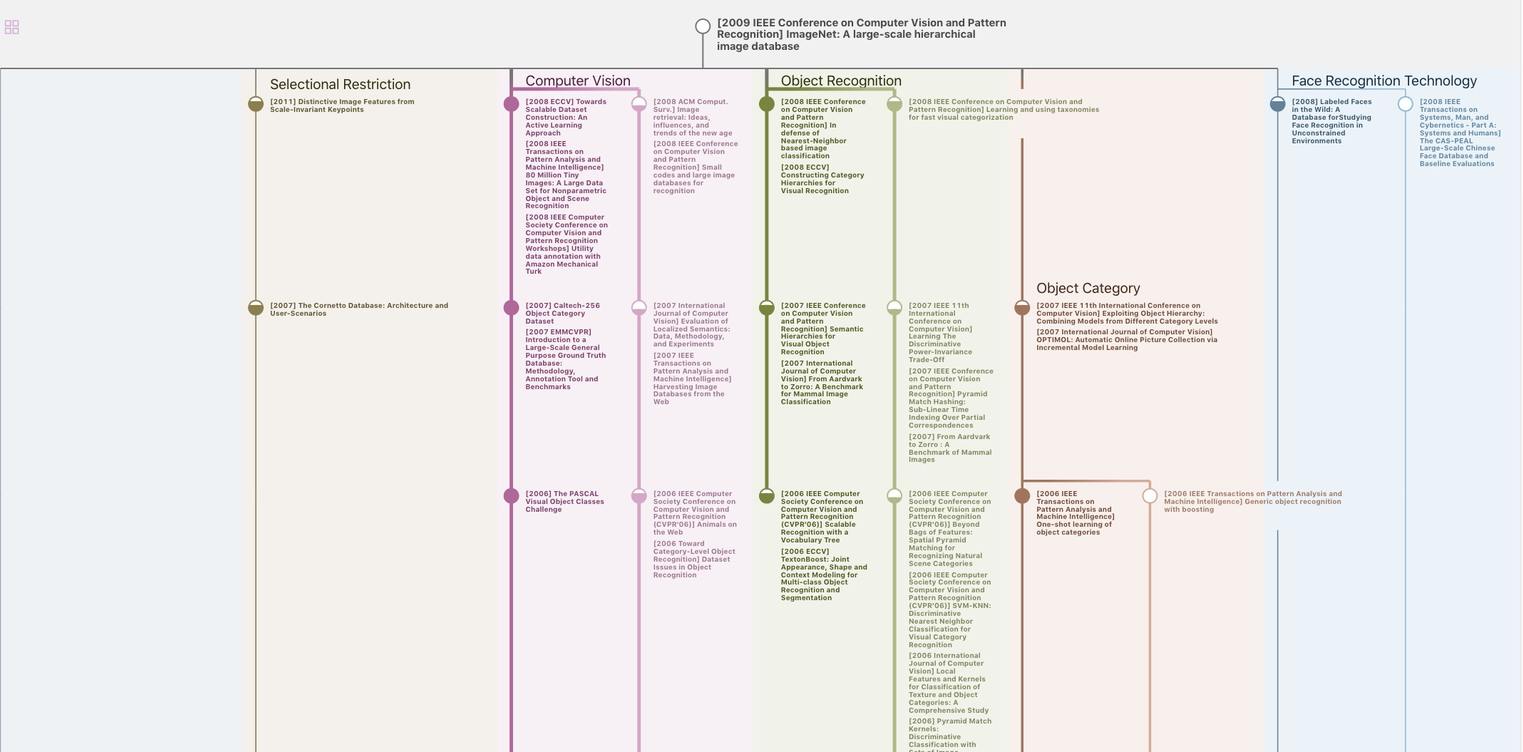
生成溯源树,研究论文发展脉络
Chat Paper
正在生成论文摘要