Deep visual transformer neural network with imbalanced algorithms for identifying weeds in tea gardens using long-tailed image datasets
JOURNAL OF NONLINEAR AND CONVEX ANALYSIS(2022)
摘要
Weeding is a necessary step during the tea cultivation process, but there are many species of weeds in tea gardens. At present, the accuracy of weed identification models in tea gardens is generally not high, which is related to the fact that the number of collected weed images conforms to the distribution of long-tailed data, and the imbalanced algorithm is one of the effective ways to solve this problem. Thus, this manuscript constructs deep learning models with an imbalanced algorithm to classify weeds in several tea gardens. The classification performance of the Vision Transformer (ViT) and other models on 38 weed species was compared. The best classification accuracy of the ViT model was 92.19%. Furthermore, weed image datasets from natural environment collections tend to have long-tailed distributions because of high cost for collecting and screening images. This study evaluated the performance of the best model with three imbalanced algorithms. The trained ViT model with over-sampling achieved an accuracy of 97.31%, which is an increase of 5.12%. The over-sampling methods increase the accuracy of the model for most weed classes in long-tailed data. Specifically, the accuracy increased from about 60% to about 80% for five species of weeds (Digitaria sanguinalis, Cynodon dactylon, Hernistepta lyrata, Cocculus orbiculatus, and Amaranthus retroflexus). Therefore, this paper provides a solution to the problem of weed classification and identification in the natural tea garden environment and provides a theoretical basis for solving the long-tailed data problem.
更多查看译文
关键词
Weed identification,class imbalance,convolutional neural networks,deep learning,image classification,long-tailed data
AI 理解论文
溯源树
样例
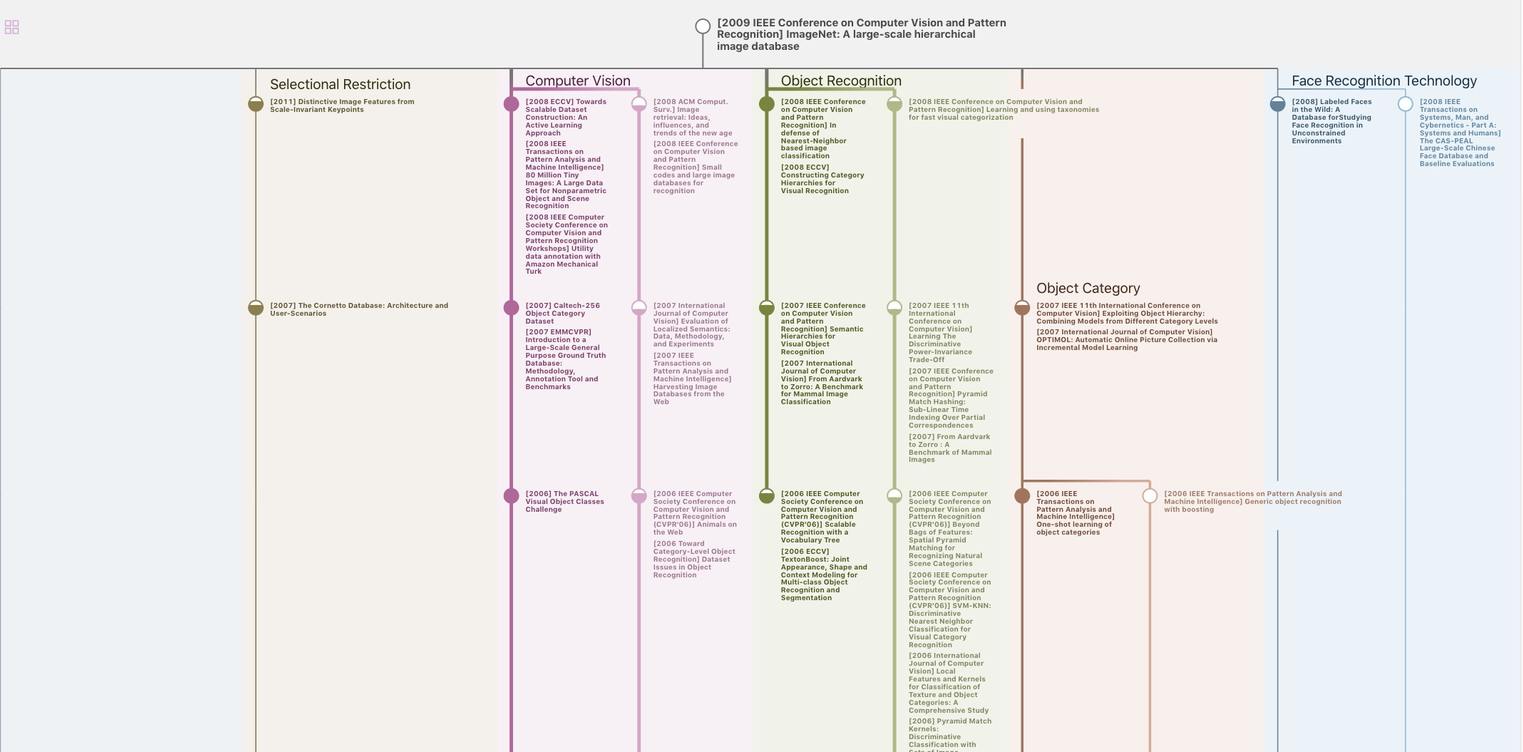
生成溯源树,研究论文发展脉络
Chat Paper
正在生成论文摘要