Exploring Category Structure with Contextual Language Models and Lexical Semantic Networks
17TH CONFERENCE OF THE EUROPEAN CHAPTER OF THE ASSOCIATION FOR COMPUTATIONAL LINGUISTICS, EACL 2023(2023)
摘要
Recent work on predicting category structure with distributional models, using either static word embeddings (Heyman and Heyman, 2019) or contextualized language models (CLMs) (Misra et al., 2021), report low correlations with human ratings, thus calling into question their plausibility as models of human semantic memory. In this work, we revisit this question testing a wider array of methods for probing CLMs for predicting typicality scores. Our experiments, using BERT (Devlin et al., 2018), show the importance of using the right type of CLM probes, as our best BERT-based typicality prediction methods substantially improve over previous works. Second, our results highlight the importance of polysemy in this task: our best results are obtained when using a disambiguation mechanism. Finally, additional experiments reveal that Information Contentbased WordNet (Miller, 1995), also endowed with disambiguation, matches the performance of the best BERT-based method, and in fact captures complementary information, which can be combined with BERT to achieve enhanced typicality predictions.
更多查看译文
关键词
lexical semantic networks,contextual language models,category structure
AI 理解论文
溯源树
样例
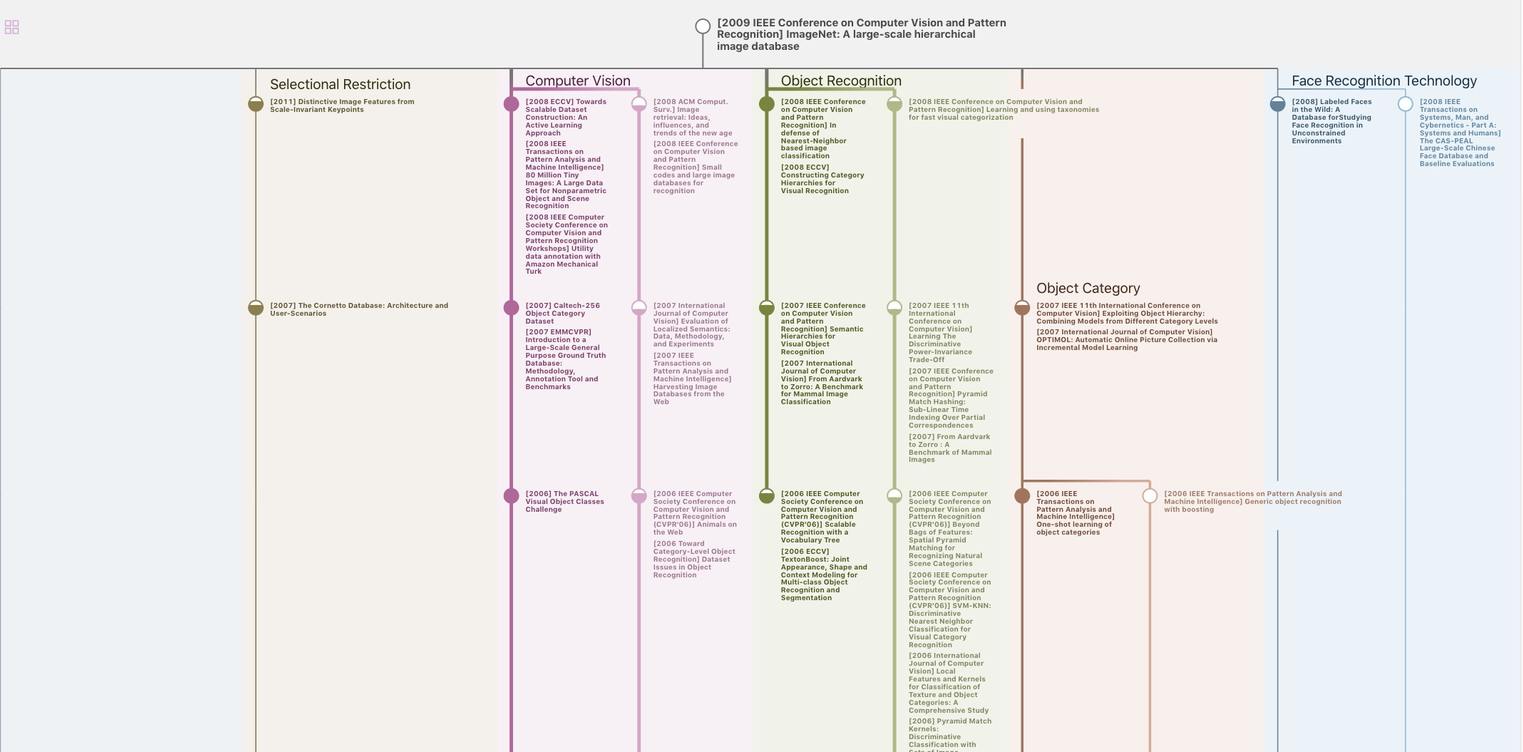
生成溯源树,研究论文发展脉络
Chat Paper
正在生成论文摘要