Learning Graph ARMA Processes From Time-Vertex Spectra
IEEE TRANSACTIONS ON SIGNAL PROCESSING(2024)
摘要
The modeling of time-varying graph signals as stationary time-vertex stochastic processes permits the inference of missing signal values by efficiently employing the correlation patterns of the process across different graph nodes and time instants. In this study, we propose an algorithm for computing graph autoregressive moving average (graph ARMA) processes based on learning the joint time-vertex power spectral density of the process from its incomplete realizations for the task of signal interpolation. Our solution relies on first roughly estimating the joint spectrum of the process from partially observed realizations and then refining this estimate by projecting it onto the spectrum manifold of the graph ARMA process through convex relaxations. The initially missing signal values are then estimated based on the learnt model. Experimental results show that the proposed approach achieves high accuracy in time-vertex signal estimation problems.
更多查看译文
关键词
Graph processes,time-vertex processes,time-varying graph signals,joint power spectral density,graph ARMA models
AI 理解论文
溯源树
样例
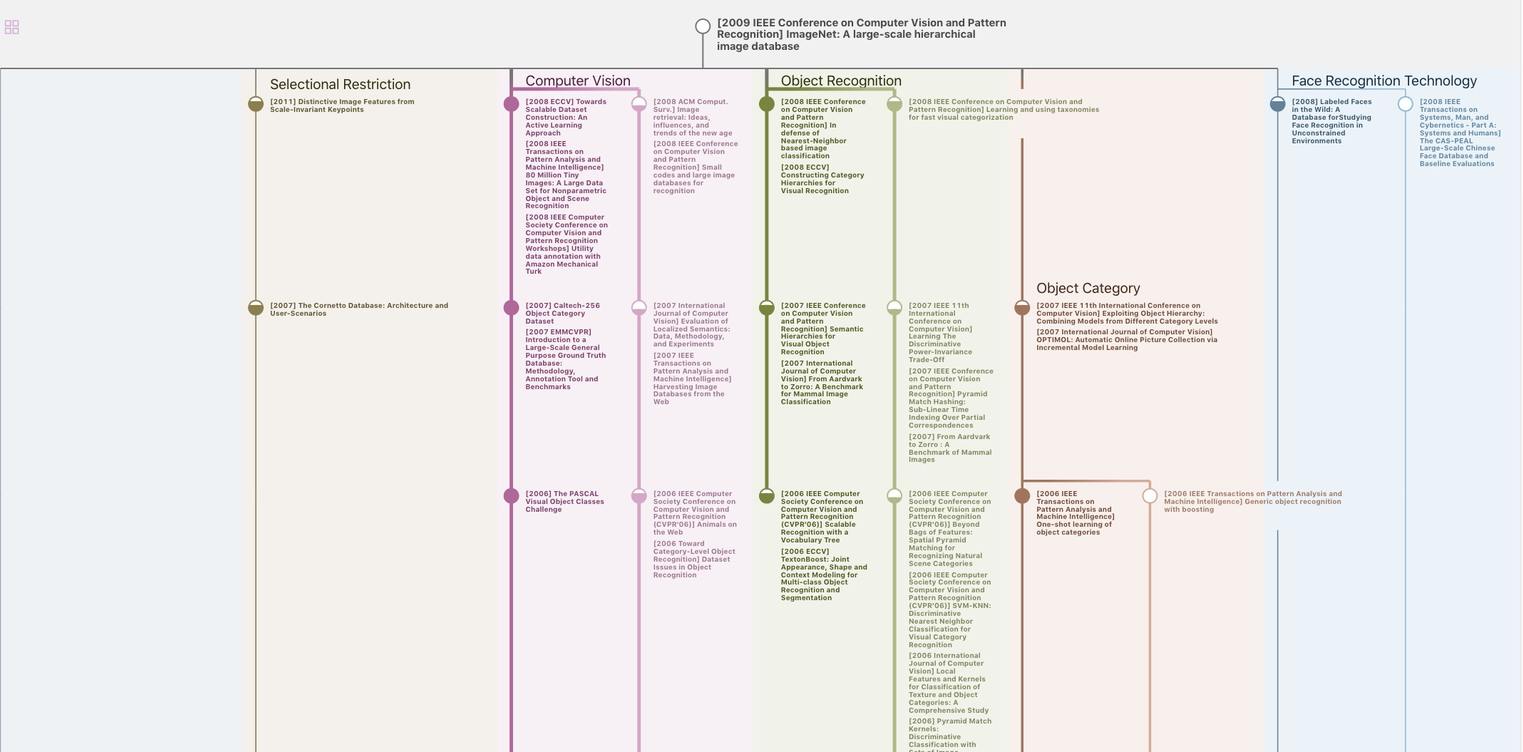
生成溯源树,研究论文发展脉络
Chat Paper
正在生成论文摘要