B-BACN: Bayesian Boundary-Aware Convolutional Network for Crack Characterization
arxiv(2023)
摘要
The accurate detection of crack boundaries is crucial for various purposes, such as condition monitoring, prognostics, and maintenance scheduling. To address this issue, we introduce a Bayesian Boundary-Aware Convolutional Network (B-BACN) that emphasizes the significance of both uncertainty quantification and boundary refinement to generate precise and reliable defect boundary detections. Our inspection model employs a multi-task learning approach, where we use Monte Carlo Dropout to learn the epistemic uncertainty and a Gaussian sampling function to predict each sample's aleatoric uncertainty. Moreover, we include a boundary refinement loss to B-BACN to enhance the determination of defect boundaries. The experimental results illustrate the effectiveness of our proposed approach in identifying crack boundaries with high accuracy, minimizing misclassification rate, and improving model calibration capabilities.
更多查看译文
AI 理解论文
溯源树
样例
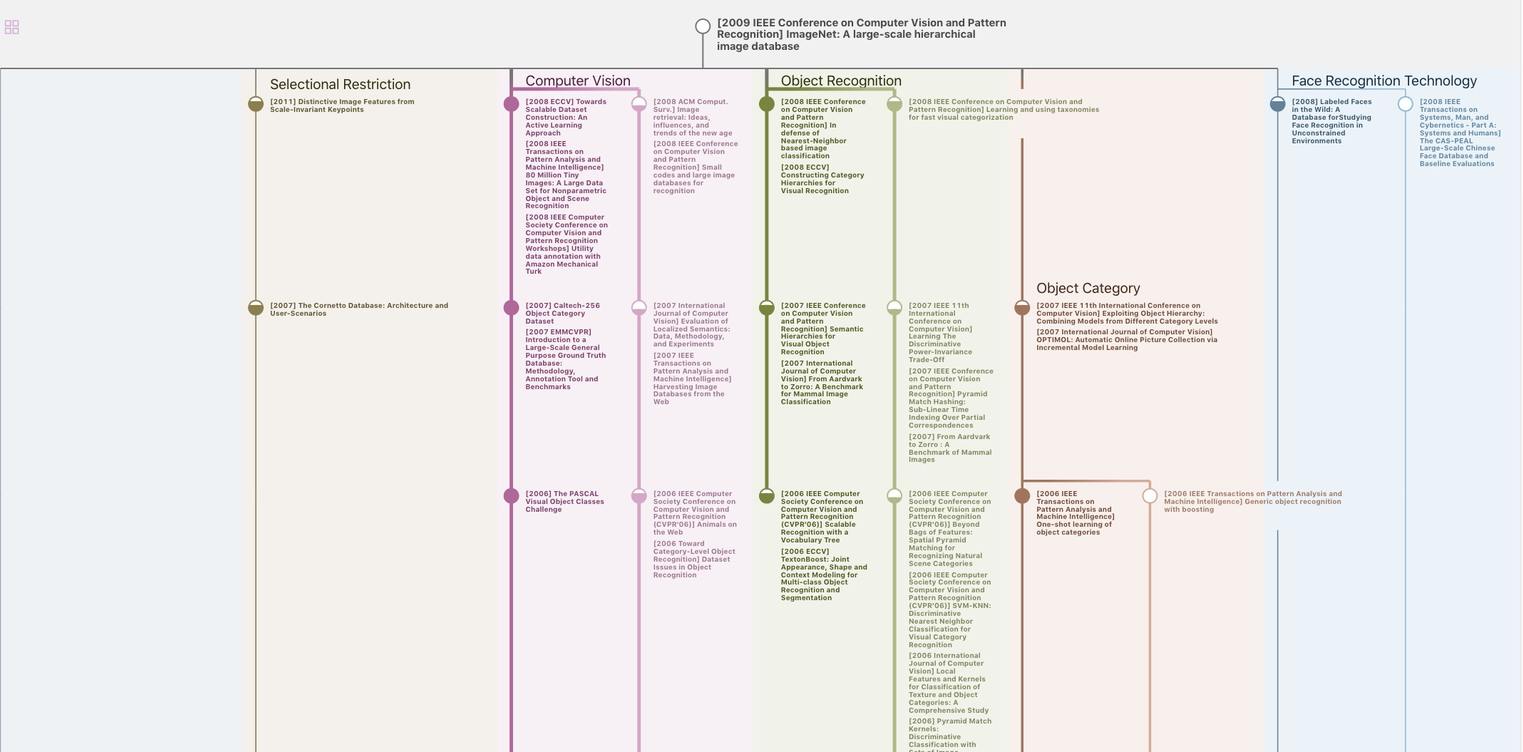
生成溯源树,研究论文发展脉络
Chat Paper
正在生成论文摘要