Deep neural operators can predict the real-time response of floating offshore structures under irregular waves
COMPUTERS & STRUCTURES(2024)
摘要
The utilization of neural operators in a digital twin model of an offshore floating structure holds the potential for a significant shift in the prediction of structural responses and health monitoring, offering valuable realtime control insights. In this work, we investigate the effectiveness of three neural operators, namely the deep operator network (DeepONet), the Fourier neural operator (FNO), and the Wavelet neural operator (WNO), to accurately capture the responses of a floating structure under six different sea state codes (3 - 8) based on the wave characteristics described by the World Meteorological Organization (WMO). To further enhance the accuracy of the vanilla architecture of the neural operators, novel extensions, such as wavelet-DeepONet and self adaptive WNO, are proposed in this paper. The results demonstrate that these high-precision neural operators can deliver structural responses more efficiently, up to two orders of magnitude faster than a dynamic analysis using conventional numerical solvers. Additionally, compared to gated recurrent units (GRUs), a commonly used recurrent neural network for time-series estimation, neural operators are both more accurate and efficient, especially in situations with limited data availability. Taken together, our study shows that FNO outperforms all other operators for approximating the mapping of one input functional space to the output space as well as for responses that have small bandwidth of the frequency spectrum. Conversely, DeepONet, with historical states, proves most accurate in learning the mapping of multiple input functions to the output space and capturing responses within a broad frequency spectrum.
更多查看译文
关键词
DeepONet,FNO,WNO,Floating offshore structures,Irregular wave
AI 理解论文
溯源树
样例
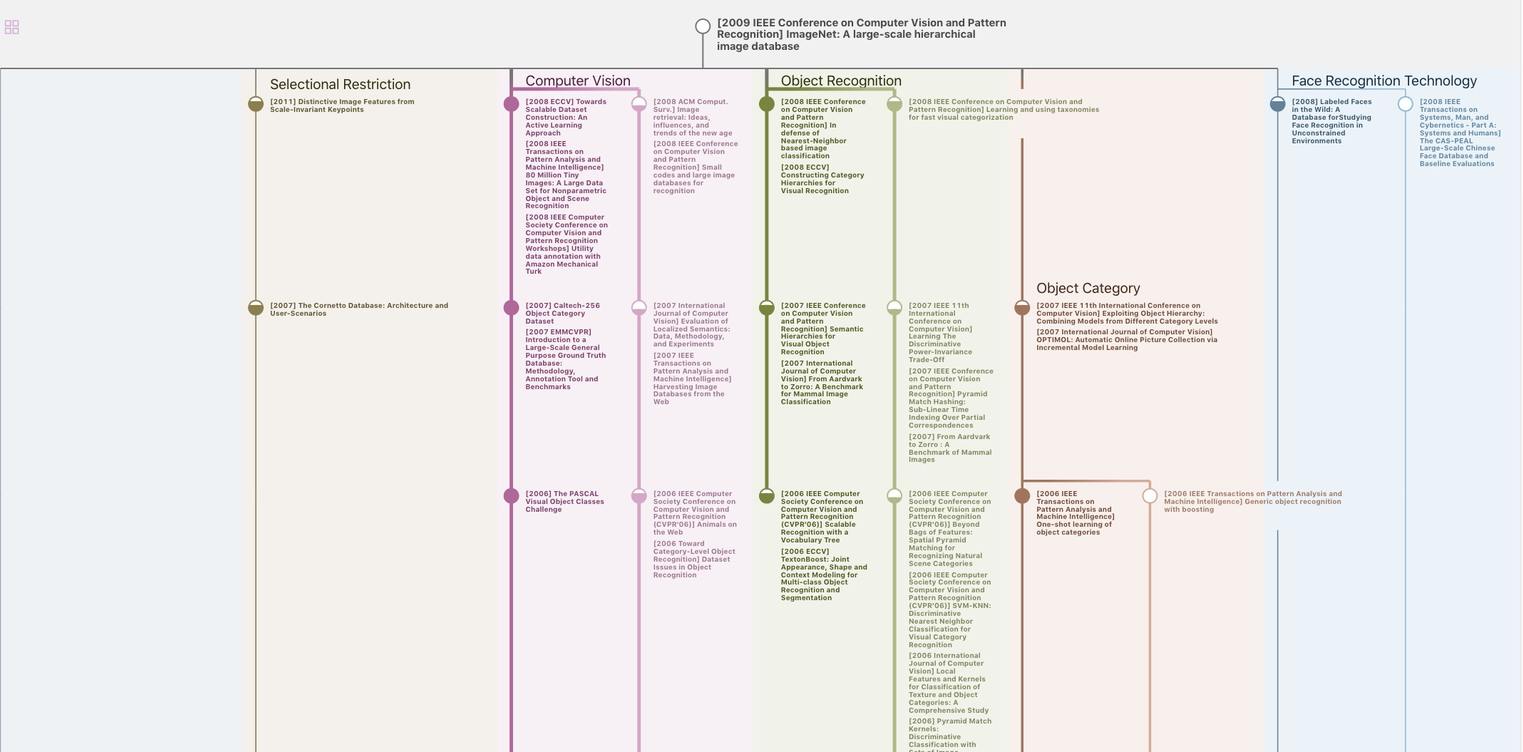
生成溯源树,研究论文发展脉络
Chat Paper
正在生成论文摘要