2SRS: Two-Stream Residual Separable Convolution Neural Network for Hyperspectral Image Classification
IEEE Geoscience and Remote Sensing Letters(2023)
Abstract
Typically, hyperspectral image suffers from redundant information, data scarcity, and class imbalance problems. This letter proposes a hyperspectral image classification framework named a two-stream residual separable convolution (2SRS) network that aims to mitigate these problems. Principal component analysis (PCA) is first employed to reduce the spectral dimension of the hyperspectral image. Subsequently, the data scarcity and class imbalance problems are overcome via spatial and spectral data augmentations. A novel spectral data creation from image patches is proposed. The augmented samples are fed into the proposed 2SRS network for hyperspectral image classification. We evaluated the proposed method on three benchmark datasets, namely, 1) Indian Pines (IP); 2) Pavia University; and 3) Salinas Scene (SA). The proposed method achieved state-of-the-art performance in terms of overall accuracy (OA), average accuracy (AA), and Kappa coefficient (Kappa) for both 30% and 10% training set ratios.
MoreTranslated text
Key words
Convolutional neural networks (CNNs),hyperspectral image classification,remote sensing,residual learning,separable 2-D-CNN
AI Read Science
Must-Reading Tree
Example
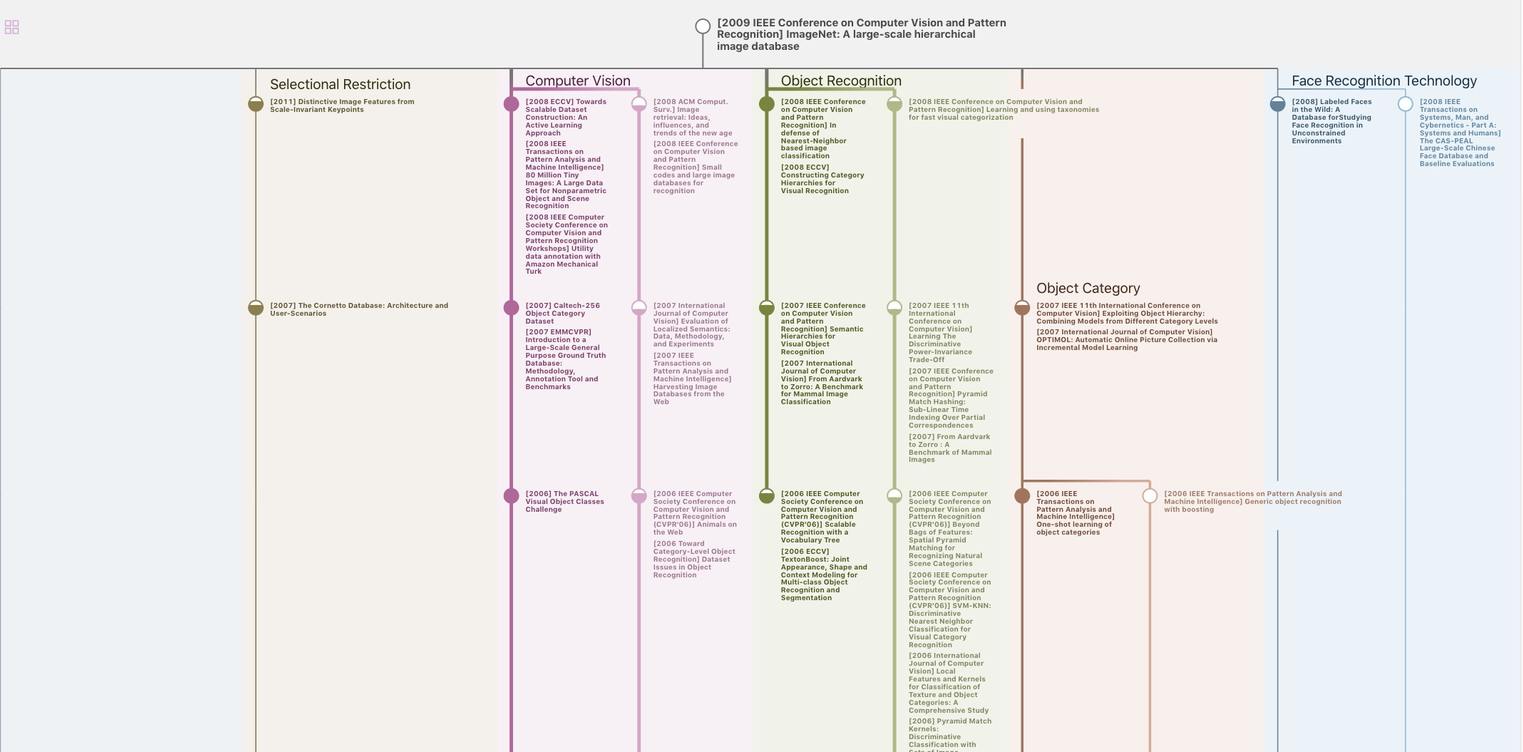
Generate MRT to find the research sequence of this paper
Chat Paper
Summary is being generated by the instructions you defined