Impact of brain parcellation on prediction error in models of cognition and demographics
biorxiv(2023)
摘要
Brain connectivity analysis begins with the selection of a parcellation scheme that will define brain regions as nodes whose connections will be studied. Brain connectivity has already been used in predictive modelling of cognition, but it remains unclear if parcellation schemes can systematically impact the predictive model error. In this work, structural, functional and combined connectivity were each defined with 5 parcellation schemes. Principal Component Regression with elastic net regularisation was fitted to each connectivity to predict individual differences in age, education, sex, Executive Function, Self-regulation, Language, Encoding and Sequence Processing. It was found that low-resolution functional parcellation consistently performed above chance at producing generalisable models of both demographics and cognition. However, no single parcellation scheme proved superior at predictive modelling. In addition, although parcellation schemes have influenced the global organisation of each connectivity type, this difference could not account for the error of the models. Taken together, this demonstrates that while high-resolution parcellations may be beneficial for modelling specific individual differences, partial voluming of signals achieved by higher resolution of parcellation likely disrupts model generalisability.
### Competing Interest Statement
The authors have declared no competing interest.
更多查看译文
AI 理解论文
溯源树
样例
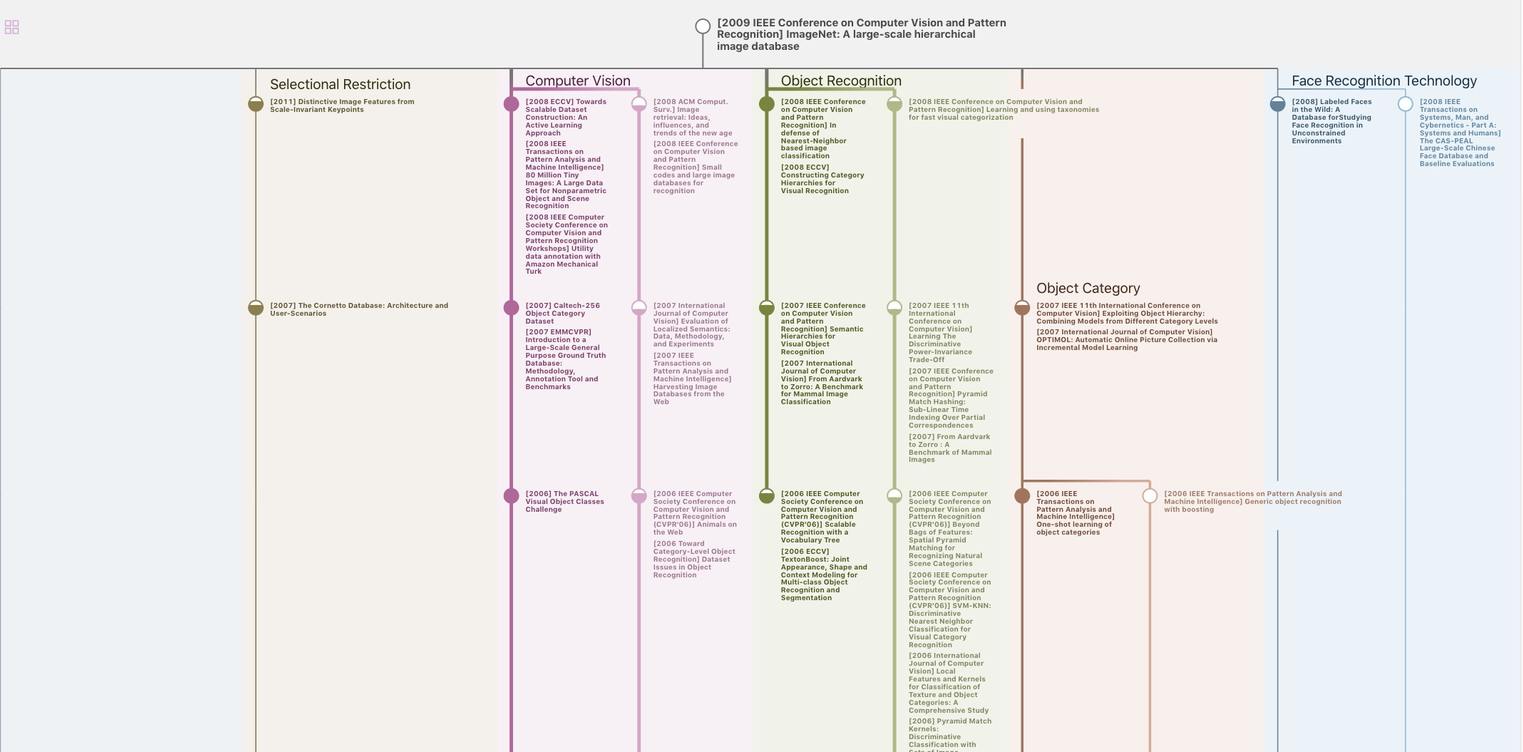
生成溯源树,研究论文发展脉络
Chat Paper
正在生成论文摘要