Ergodicity-to-localization transition on random regular graphs with large connectivity and in many-body quantum dots
arXiv (Cornell University)(2023)
Abstract
Anderson localization on random regular graphs (RRG) serves as a toy-model of many-body localization (MBL). We explore the transition for ergodicity to localization on RRG with large connectivity $m$. In the analytical part, we focus on the inverse participation ratio of eigenstates and identify several regimes on the ergodic side of the transition -- self-consistent golden rule, golden rule, precritical, and critical -- that the system consecutively goes through when the disorder increases towards the point $W_c$ of the localization transition. We also perform exact-diagonalization numerics as well as a population-dynamics analysis that combines analytical and numerical techniques. Results of all the approaches are in excellent mutual agreement. We further explore the evolution from ergodicity to localization in two models of Fock-space MBL: fermionic and spin quantum dots. Large-connectivity RRG models serve as an approximation for this class of many-body problems; one of our central goals is to better understand the status of this approximation. Our numerical simulations support the conjecture that, in the limit of a large system, there is a sharp ergodicity-to-localization transition in the quantum-dot models. Furthermore, our results are consistent the RRG-like scaling of the critical disorder, $W_c \sim m \ln m$. While in the golden-rule range the behavior of quantum-dot models is in agreement with analytical predictions based on RRG model, substantial deviations occur in the pre-critical regime. Our results indicate that the pre-critical and critical behavior (as well as the numerical coefficient in the formula for $W_c$) in the quantum-dot models may be different from what one would expect from the RRG-like approximation.
MoreTranslated text
Key words
random regular graphs,large connectivity,quantum,ergodicity-to-localization,many-body
AI Read Science
Must-Reading Tree
Example
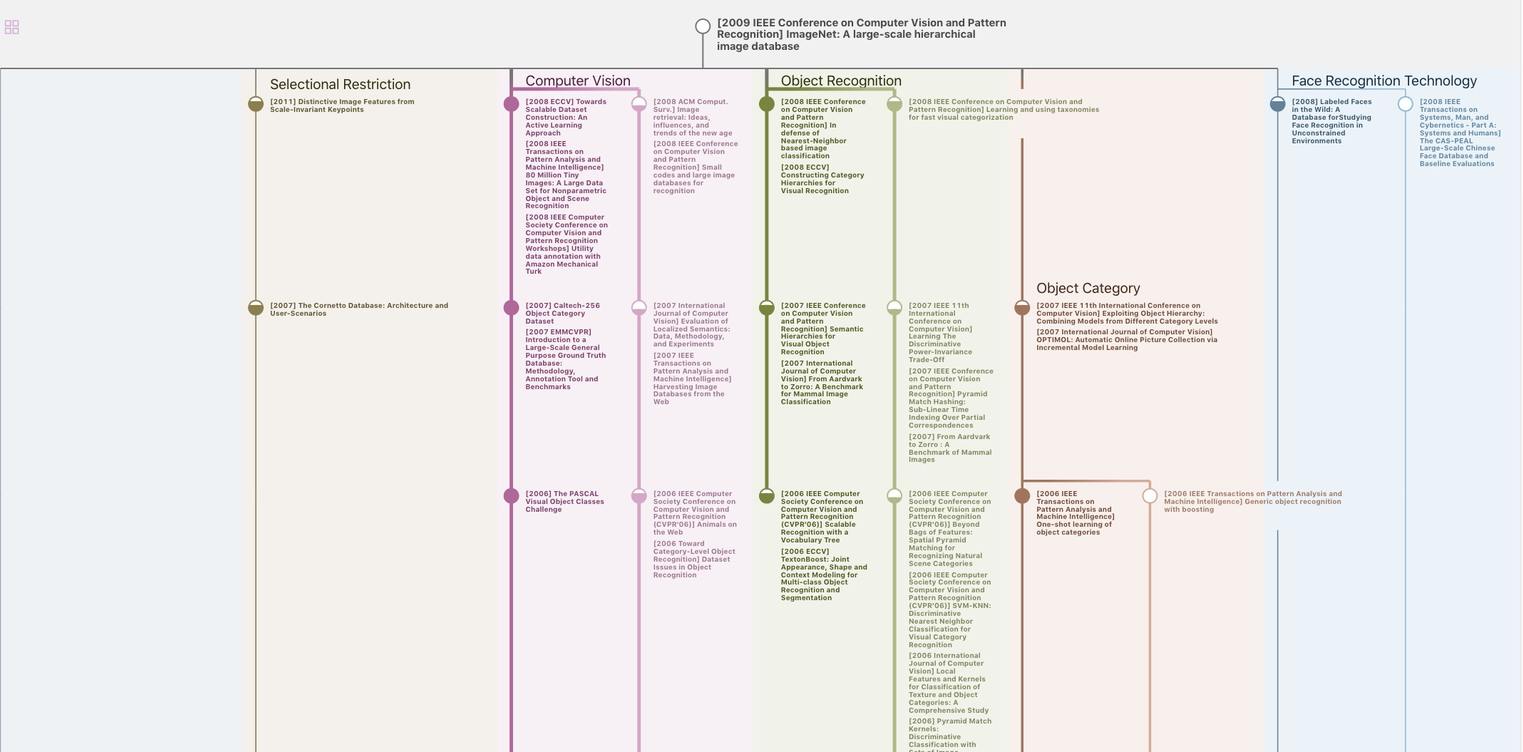
Generate MRT to find the research sequence of this paper
Chat Paper
Summary is being generated by the instructions you defined