Expediting Distributed DNN Training With Device Topology-Aware Graph Deployment
IEEE Transactions on Parallel and Distributed Systems(2023)
摘要
This paper presents TAG, an automatic system to derive optimized DNN training graph and its deployment onto any device topology, for expedited training in device- and topology- heterogeneous ML clusters. We novelly combine both the DNN computation graph and the device topology graph as input to a graph neural network (GNN), and join the GNN with a search-based method to quickly identify optimized distributed training strategies. To reduce communication in a heterogeneous cluster, we further explore a lossless gradient compression technique and solve a combinatorial optimization problem to automatically apply the technique for training time minimization. We evaluate TAG with various representative DNN models and device topologies, showing that it can achieve up to 4.56x training speed-up as compared to existing schemes. TAG can produce efficient deployment strategies for both unseen DNN models and unseen device topologies, without heavy fine-tuning.
更多查看译文
关键词
Distributed systems,machine learning
AI 理解论文
溯源树
样例
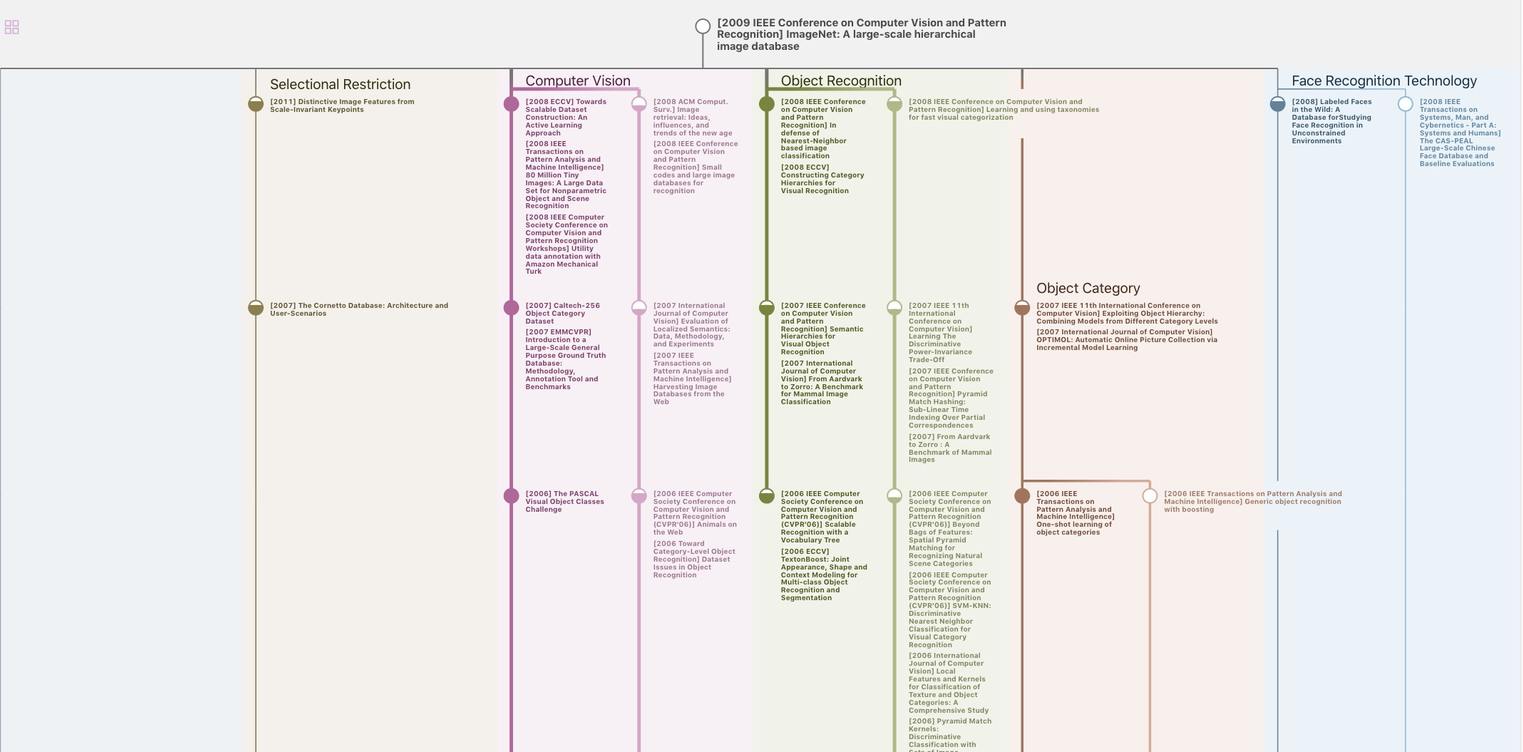
生成溯源树,研究论文发展脉络
Chat Paper
正在生成论文摘要