Algorithmic Aspects of the Log-Laplace Transform and a Non-Euclidean Proximal Sampler
arxiv(2023)
摘要
The development of efficient sampling algorithms catering to non-Euclidean geometries has been a challenging endeavor, as discretization techniques which succeed in the Euclidean setting do not readily carry over to more general settings. We develop a non-Euclidean analog of the recent proximal sampler of [LST21], which naturally induces regularization by an object known as the log-Laplace transform (LLT) of a density. We prove new mathematical properties (with an algorithmic flavor) of the LLT, such as strong convexity-smoothness duality and an isoperimetric inequality, which are used to prove a mixing time on our proximal sampler matching [LST21] under a warm start. As our main application, we show our warm-started sampler improves the value oracle complexity of differentially private convex optimization in $\ell_p$ and Schatten-$p$ norms for $p \in [1, 2]$ to match the Euclidean setting [GLL22], while retaining state-of-the-art excess risk bounds [GLLST23]. We find our investigation of the LLT to be a promising proof-of-concept of its utility as a tool for designing samplers, and outline directions for future exploration.
更多查看译文
关键词
proximal sampler,transform,log-laplace,non-euclidean
AI 理解论文
溯源树
样例
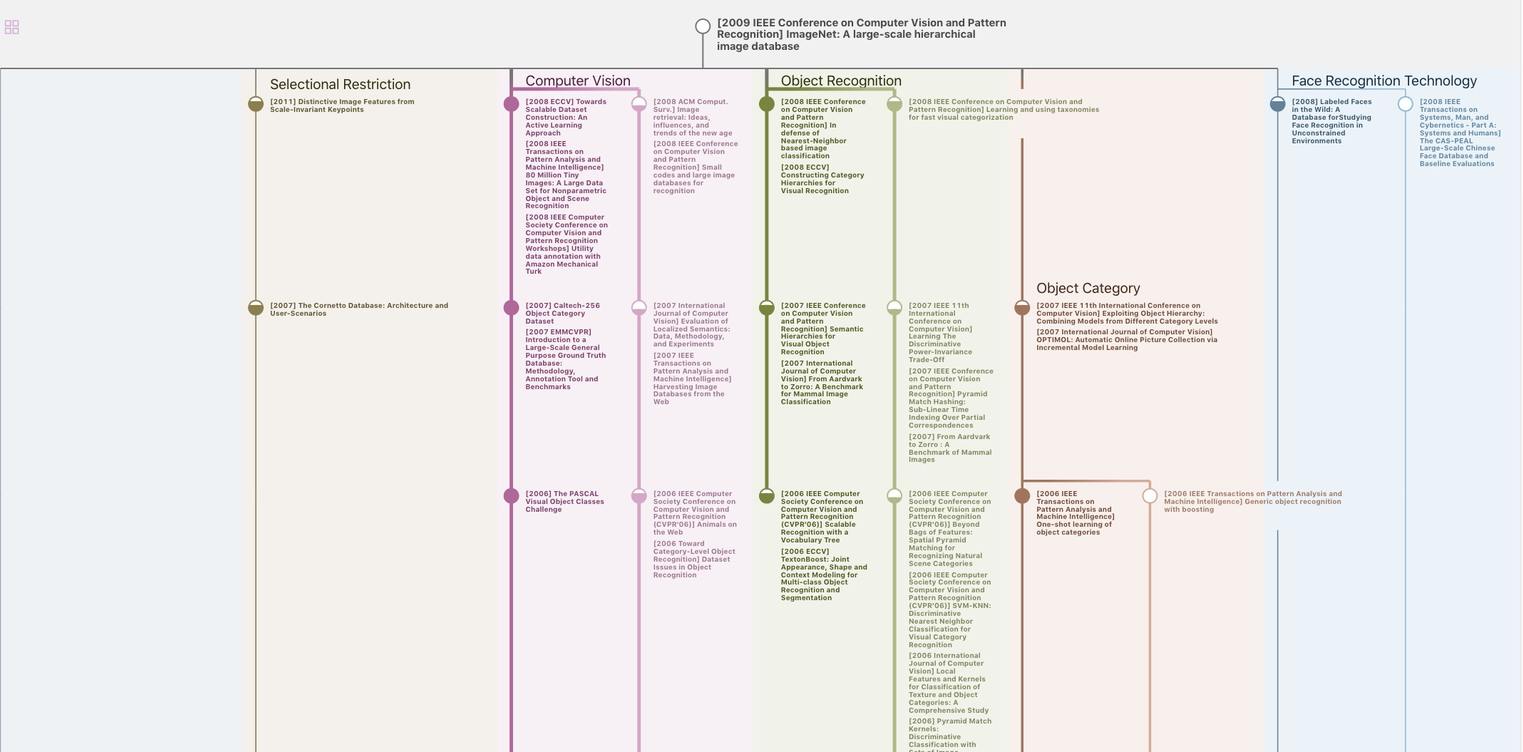
生成溯源树,研究论文发展脉络
Chat Paper
正在生成论文摘要