Machine Learning Assisted Bad Data Detection for High-throughput Substation Communication
ICC 2023 - IEEE INTERNATIONAL CONFERENCE ON COMMUNICATIONS(2023)
摘要
Electrical substations are becoming more prone to cyber-attacks due to increasing digitalization. Prevailing defence measures based on cyber rules are often inadequate to detect attacks that use legitimate-looking measurements. In this work, we design and implement a bad data detection solution for electrical substations called ResiGate, that effectively combines a physics-based approach and a machine-learning-based approach to provide substantial speed-up in high-throughput substation communication scenarios, while still maintaining high detection accuracy and confidence. While many existing physics-based schemes are designed for deployment in control centers (due to their high computational requirement), ResiGate is designed as a security appliance that can be deployed on low-cost industrial computers at the edge of the smart grid so that it can detect local substation-level attacks in a timely manner. A key challenge for this is to continuously run the computationally demanding physics-based analysis to monitor the measurement data frequently transmitted in a typical substation. To provide high throughput without sacrificing accuracy, ResiGate uses machine learning to effectively filter out most of the non-suspicious (normal) data and thereby reducing the overall computational load, allowing efficient performance even with a high volume of network traffic. We implement ResiGate on a low-cost industrial computer and our experiments confirm that ResiGate can detect attacks with zero error while sustaining a high throughput.
更多查看译文
AI 理解论文
溯源树
样例
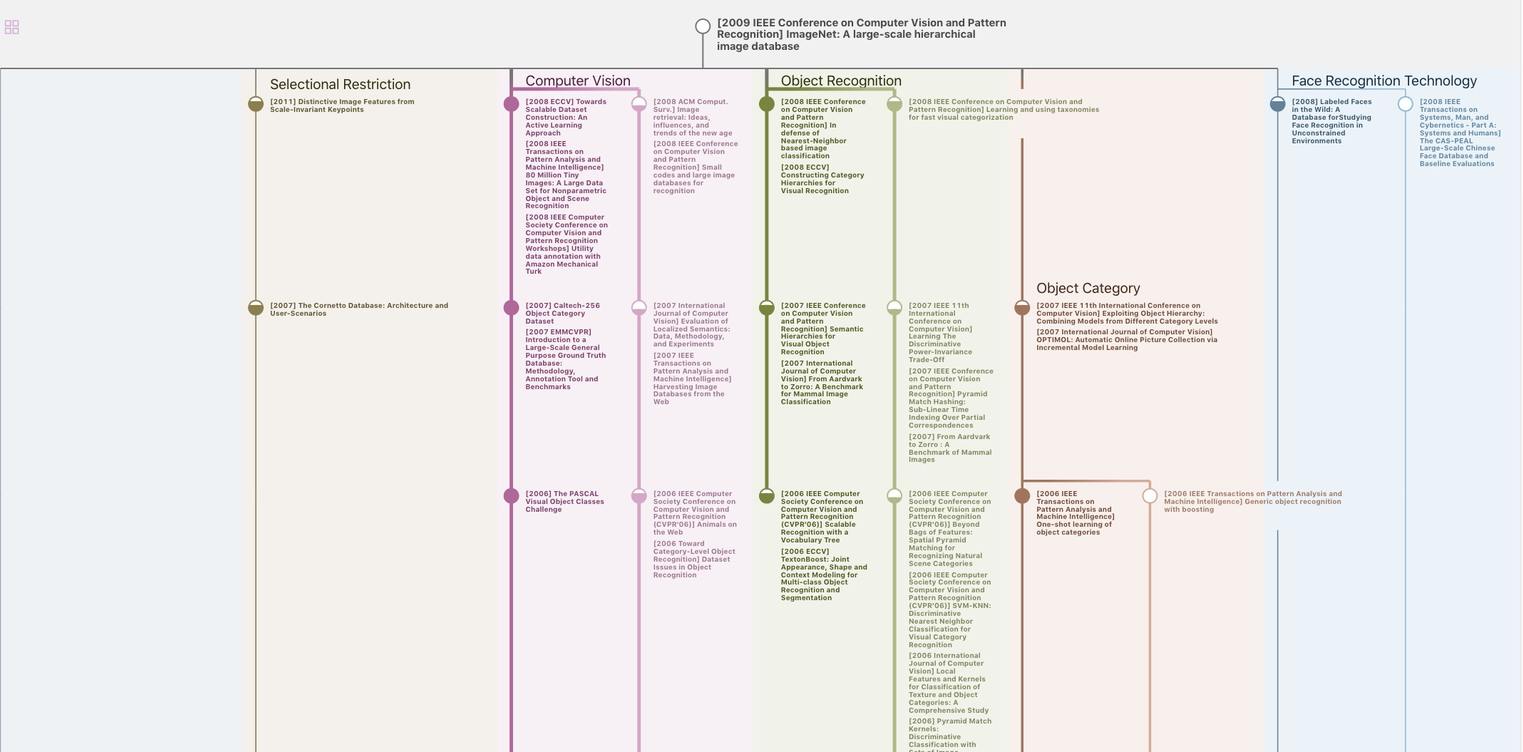
生成溯源树,研究论文发展脉络
Chat Paper
正在生成论文摘要