Industrial and Medical Anomaly Detection Through Cycle-Consistent Adversarial Networks
arxiv(2023)
摘要
In this study, a new Anomaly Detection (AD) approach for industrial and
medical images is proposed. This method leverages the theoretical strengths of
unsupervised learning and the data availability of both normal and abnormal
classes. Indeed, the AD is often formulated as an unsupervised task, implying
only normal images during training. These normal images are devoted to be
reconstructed, through an autoencoder architecture for instance. However, the
information contained in abnormal data, when available, is also valuable for
this reconstruction. The model would be able to identify its weaknesses by
better learning how to transform an abnormal (respectively normal) image into a
normal (respectively abnormal) one, helping the entire model to learn better
than a single normal to normal reconstruction. To address this challenge, the
proposed method uses Cycle-Generative Adversarial Networks (Cycle-GAN) for
(ab)normal-to-normal translation. After an input image has been reconstructed
by the normal generator, an anomaly score quantifies the differences between
the input and its reconstruction. Based on a threshold set to satisfy a
business quality constraint, the input image is then flagged as normal or not.
The proposed method is evaluated on industrial and medical datasets. The
results demonstrate accurate performance with a zero false negative constraint
compared to state-of-the-art methods. The code is available at
https://github.com/ValDelch/CycleGANS-AnomalyDetection.
更多查看译文
关键词
medical anomaly detection,anomaly detection,networks,cycle-consistent
AI 理解论文
溯源树
样例
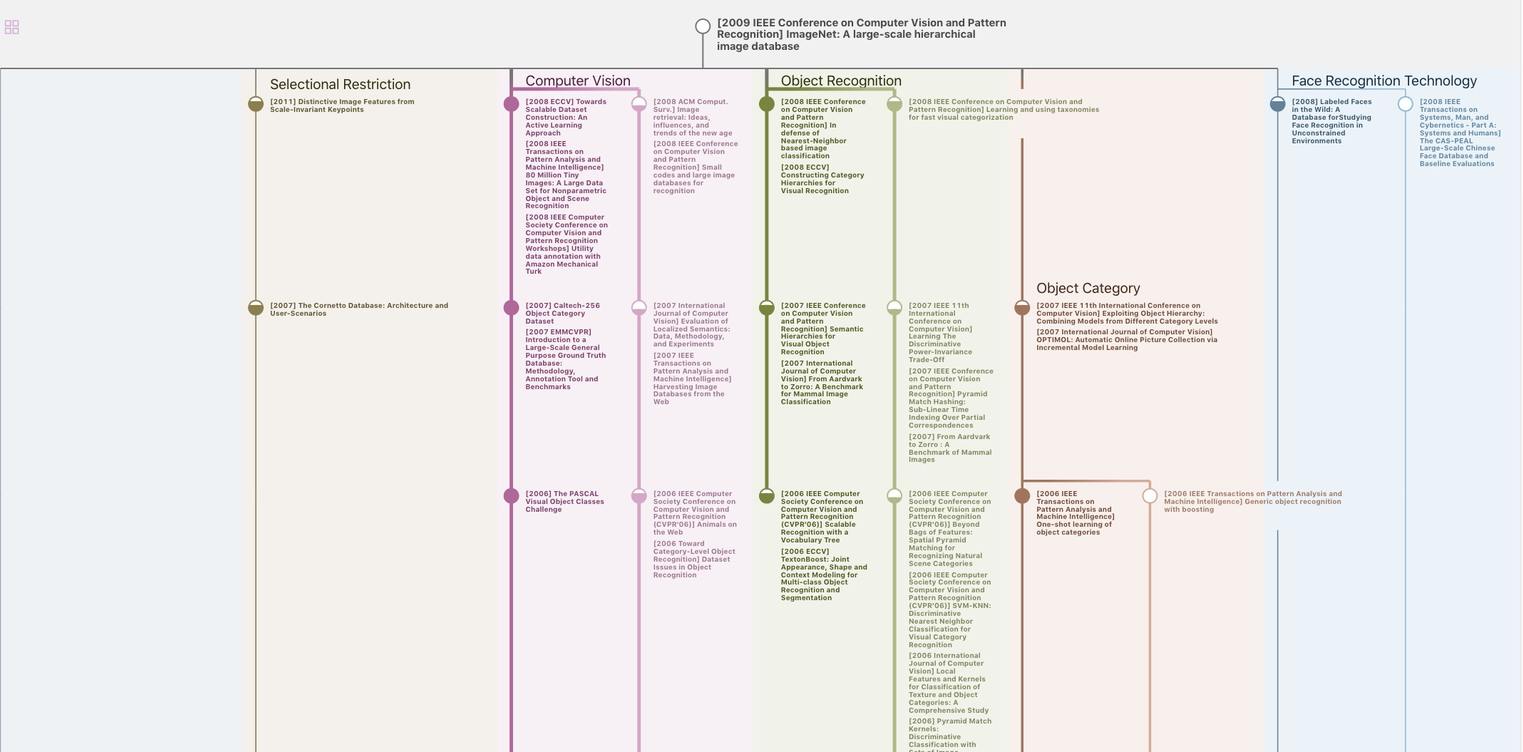
生成溯源树,研究论文发展脉络
Chat Paper
正在生成论文摘要