Data-Driven Stochastic Motion Evaluation and Optimization with Image by Spatially-Aligned Temporal Encoding
2023 IEEE INTERNATIONAL CONFERENCE ON ROBOTICS AND AUTOMATION, ICRA(2023)
Abstract
This paper proposes a probabilistic motion prediction method for long motions. The motion is predicted so that it accomplishes a task from the initial state observed in the given image. While our method evaluates the task achievability by the Energy-Based Model (EBM), previous EBMs are not designed for evaluating the consistency between different domains (i.e., image and motion in our method). Our method seamlessly integrates the image and motion data into the image feature domain by spatially-aligned temporal encoding so that features are extracted along the motion trajectory projected onto the image. Furthermore, this paper also proposes a data-driven motion optimization method, Deep Motion Optimizer (DMO), that works with EBM for motion prediction. Different from previous gradient-based optimizers, our self-supervised DMO alleviates the difficulty of hyper-parameter tuning to avoid local minima. The effectiveness of the proposed method is demonstrated with a variety of experiments with similar SOTA methods.
MoreTranslated text
Key words
data-driven motion optimization method,data-driven stochastic Motion evaluation,Deep Motion Optimizer,EBM,Energy-Based Model,image feature domain,initial state,long motions,motion data,motion trajectory,previous EBMs,previous gradient-based optimizers,probabilistic motion prediction method,similar SOTA methods,spatially-aligned temporal encoding,task achievability
AI Read Science
Must-Reading Tree
Example
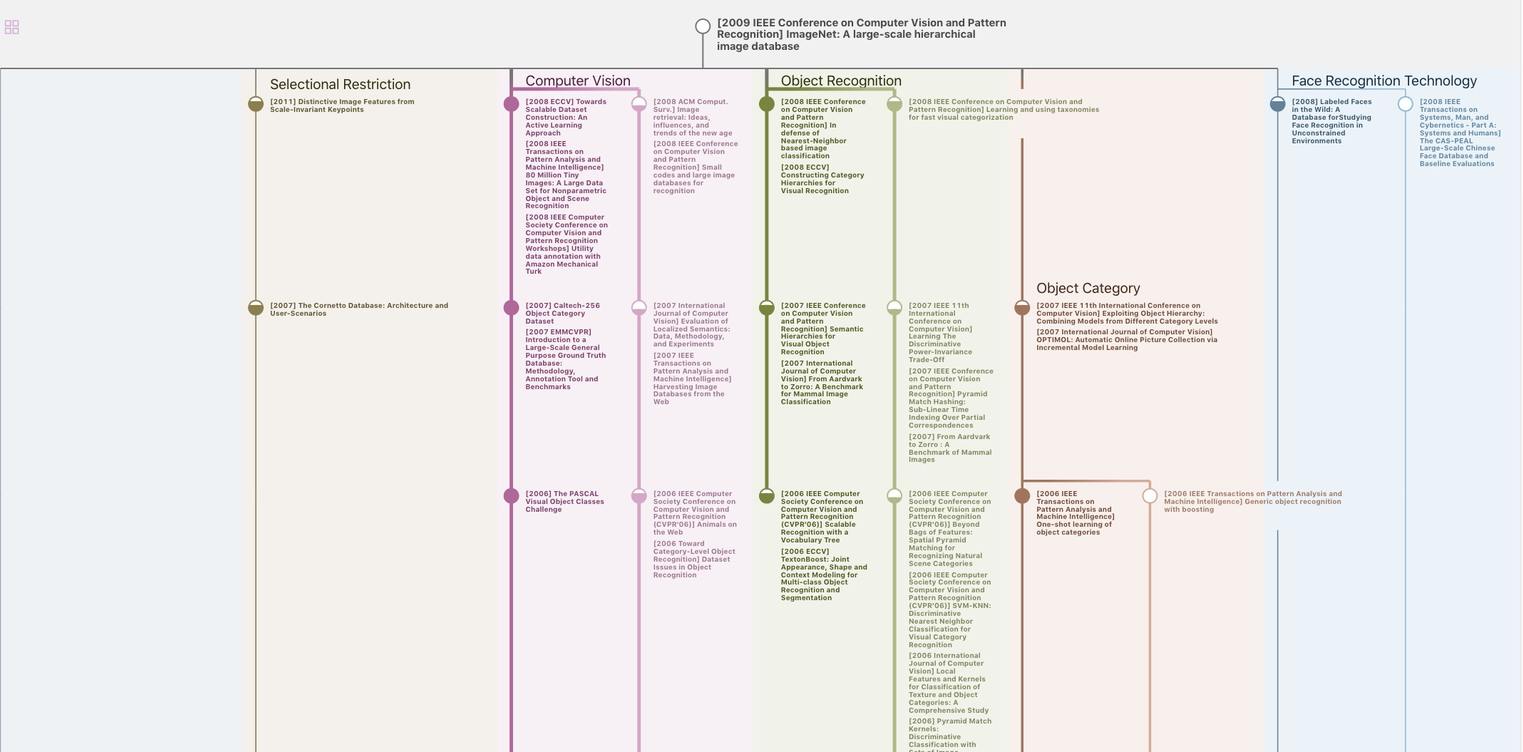
Generate MRT to find the research sequence of this paper
Chat Paper
Summary is being generated by the instructions you defined