Efficient Propagation of Uncertainty via Reordering Monte Carlo Samples
arxiv(2023)
摘要
Uncertainty analysis in the outcomes of model predictions is a key element in decision-based material design to establish confidence in the models and evaluate the fidelity of models. Uncertainty Propagation (UP) is a technique to determine model output uncertainties based on the uncertainty in its input variables. The most common and simplest approach to propagate the uncertainty from a model inputs to its outputs is by feeding a large number of samples to the model, known as Monte Carlo (MC) simulation which requires exhaustive sampling from the input variable distributions. However, MC simulations are impractical when models are computationally expensive. In this work, we investigate the hypothesis that while all samples are useful on average, some samples must be more useful than others. Thus, reordering MC samples and propagating more useful samples can lead to enhanced convergence in statistics of interest earlier and thus, reducing the computational burden of UP process. Here, we introduce a methodology to adaptively reorder MC samples and show how it results in reduction of computational expense of UP processes.
更多查看译文
关键词
uncertainty,propagation,monte
AI 理解论文
溯源树
样例
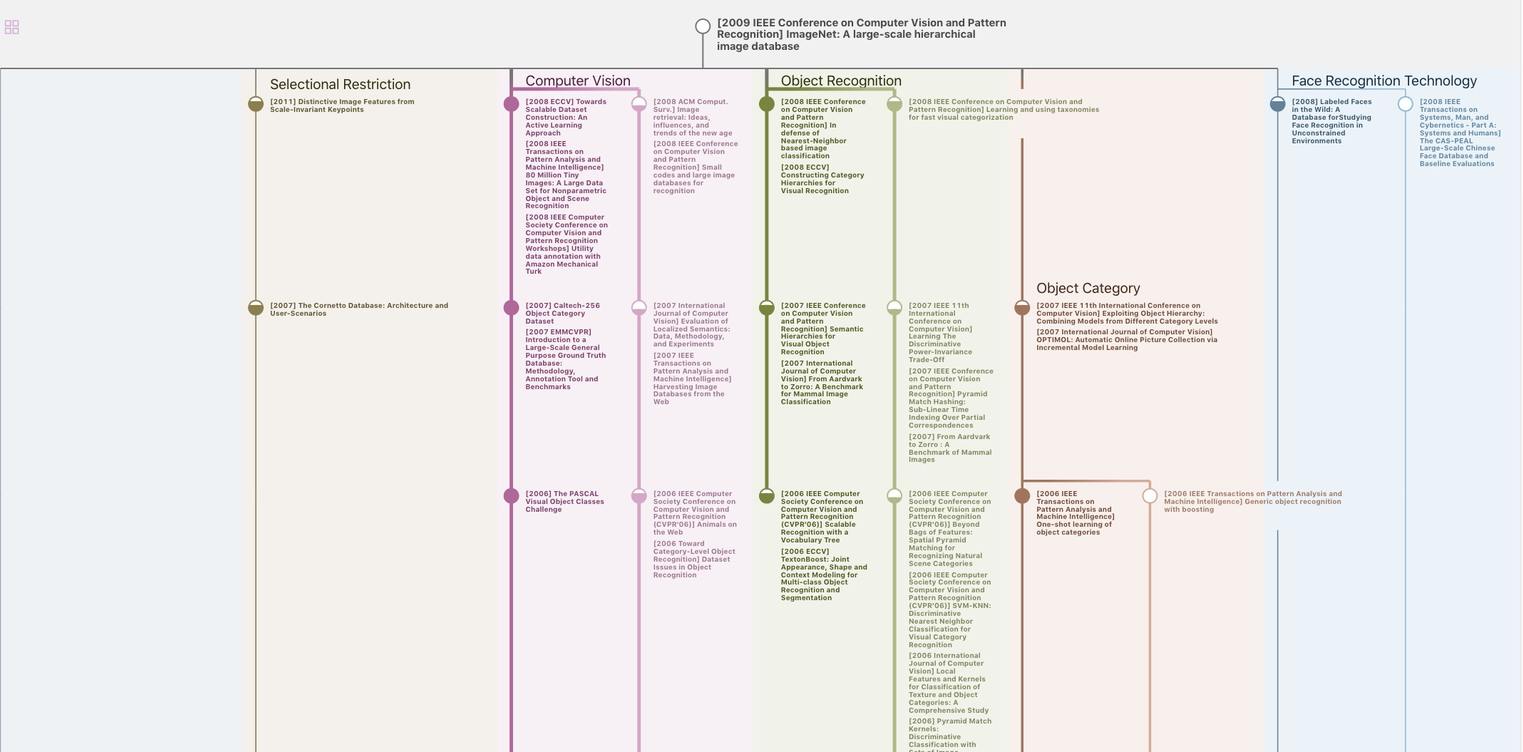
生成溯源树,研究论文发展脉络
Chat Paper
正在生成论文摘要